Embedded deep-learning based sample-to-answer device for on-site malaria diagnosis
medrxiv(2023)
摘要
In response to the ongoing global health problem caused by malaria, especially in resource-limited settings, digital microscopy must be improved to overcome the limitations associated with manual microscopy. In order to present a malaria diagnosis method that is not only accurate at the cell level but also clinically performs well, improvements in deep-learning algorithms and consistent staining results are necessary. The device employs a solid hydrogel staining method for consistent blood film preparation, eliminating the need for complex equipment and liquid reagent maintenance. By leveraging deformable staining patches, the miLab™ ensures consistent, high-quality reproducible blood films can be made across various hematocrits. Embedded deep-learning enables the miLab™ to detect and classify malaria parasites from the autofocused images of stained blood cells by internal optical system, achieving a high correlation with manual microscopy images. This innovation not only minimizes human error but also facilitates remote assistance and review by experts through digital image transmission, revolutionizing the landscape of on-site malaria diagnosis. The miLab™ algorithm for malaria detection shows a total accuracy of 98.83% for infected RBC classification. Clinical validation in Malawi demonstrates an overall percent agreement of 92.21%, highlighting the miLab™’s potential as a reliable and efficient tool for decentralized malaria diagnosis.
### Competing Interest Statement
The authors declare the following competing financial interest(s): CYB, YMS, MK, YS, HJL, KHK, YJK, SC, BMW, KHC are currently employees of Noul Co., Ltd. HWL, BK, SH were employees of Noul Co., Ltd. DKL and HB are a member of Scientific Advisory Board (Technical Consultant) in Noul Co., Ltd.
### Funding Statement
This work was funded by a grant of the Korea Health Technology R&D Project through the Korea Health Industry Development Institute (KHIDI), funded by the Ministry of Health & Welfare, Republic of Korea (grant number: HI18C1685).
### Author Declarations
I confirm all relevant ethical guidelines have been followed, and any necessary IRB and/or ethics committee approvals have been obtained.
Yes
The details of the IRB/oversight body that provided approval or exemption for the research described are given below:
Ethics committee/IRB of the Korea National Institute for Bioethics Policy (P01-202003-31-007, Seoul, Korea) and GCLabs (GCL-2020-1011-01, Yongin, Korea) gave ethical approval for this work. Ethics committee/IRB of National Health Sciences Research Committee (IRB00003905, Ministry of Health, Malawi) gave ethical approval for this work.
I confirm that all necessary patient/participant consent has been obtained and the appropriate institutional forms have been archived, and that any patient/participant/sample identifiers included were not known to anyone (e.g., hospital staff, patients or participants themselves) outside the research group so cannot be used to identify individuals.
Yes
I understand that all clinical trials and any other prospective interventional studies must be registered with an ICMJE-approved registry, such as ClinicalTrials.gov. I confirm that any such study reported in the manuscript has been registered and the trial registration ID is provided (note: if posting a prospective study registered retrospectively, please provide a statement in the trial ID field explaining why the study was not registered in advance).
Yes
I have followed all appropriate research reporting guidelines, such as any relevant EQUATOR Network research reporting checklist(s) and other pertinent material, if applicable.
Yes
All data produced in the present study are available upon reasonable request to the authors
更多查看译文
AI 理解论文
溯源树
样例
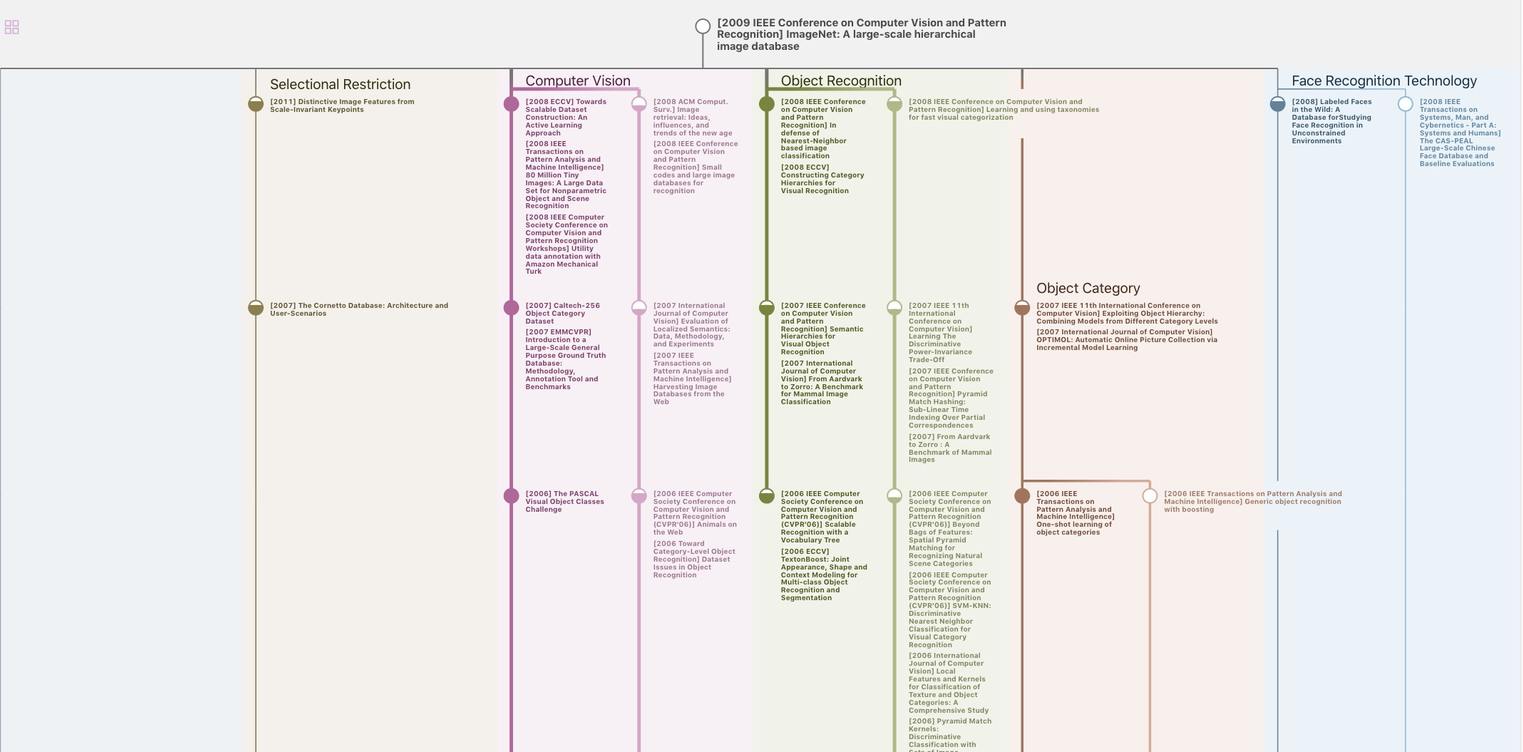
生成溯源树,研究论文发展脉络
Chat Paper
正在生成论文摘要