Let's Do the Time-Warp-attend: Learning Topological Invariants of Dynamical Systems
ICLR(2024)
摘要
Dynamical systems across the sciences, from electrical circuits to ecological networks, undergo qualitative and often catastrophic changes in behavior called \textit{bifurcations} when their underlying parameters cross a threshold. Existing methods predict oncoming catastrophes from time-series in individual systems but struggle both to categorize qualitative dynamical regimes across diverse systems and to generalize to real data. To address this challenge, we propose a data-driven, physically-informed deep-learning framework for classifying dynamical regimes and characterizing bifurcation boundaries based on the extraction of topologically invariant features. We focus on the paradigmatic case of the supercritical Hopf bifurcation, which is used to model periodic dynamics across a wide range of applications. Our convolutional attention method is trained with data augmentations that encourage the learning of topological invariants which can be used to detect bifurcation boundaries in unseen systems and to design models of biological systems like oscillatory gene regulatory networks. We further demonstrate our method's use in analyzing real data, recovering distinct proliferation and differentiation dynamics along pancreatic endocrinogenesis trajectory in gene expression space based on single-cell data. Our method provides valuable insights into the qualitative, long-term behavior of a wide range of dynamical systems as well as detect bifurcations or catastrophic transitions in large-scale physical and biological systems.
更多查看译文
关键词
dynamical systems,bifurcations,topological invariance,Hopf bifurcation,physics-informed machine learning,augmentation,RNA velocity,repressilator,pancreatic endocrinogenesis
AI 理解论文
溯源树
样例
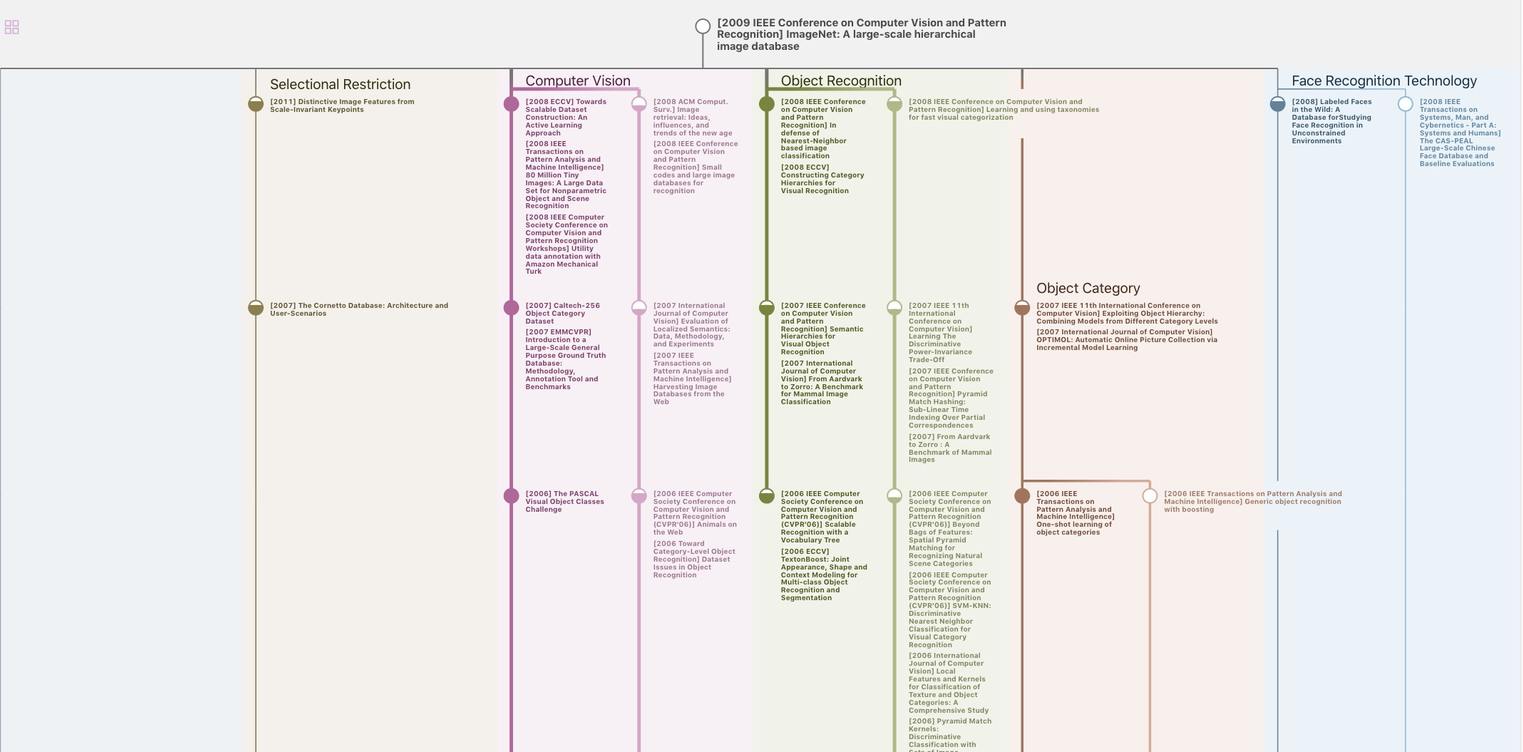
生成溯源树,研究论文发展脉络
Chat Paper
正在生成论文摘要