SAM-Net: Self-Attention based Feature Matching with Spatial Transformers and Knowledge Distillation
EXPERT SYSTEMS WITH APPLICATIONS(2024)
摘要
In this research paper, we introduce a novel approach to enhance the performance of 2D feature matching and pose estimation through the integration of a hierarchical attention mechanism and knowledge distillation. Our proposed hierarchical attention mechanism operates at multiple scales, enabling both global context awareness and precise matching of 2D features, which is crucial for various computer vision tasks. To further improve our model's performance, we incorporate insights from an existing model PixLoc (Sarlin et al., 2021) through knowledge distillation, effectively acquiring its behavior and capabilities by ignoring dynamic objects. SAM-Net outperforms state-of-the-art methods, validated on both indoor and outdoor public datasets. For the indoor dataset, our approach achieves remarkable AUC (5 degrees /10 degrees /20 degrees) scores of 55.31/71.70/83.37. Similarly, for the outdoor dataset, we demonstrate outstanding AUC values of 26.01/46.44/63.61. Furthermore, SAM-Net achieves top ranking among published methods in two public visual localization benchmarks, highlighting the real benefits of the proposed method. The code and test suite can be accessed at link.1
更多查看译文
关键词
Geometric features extraction,Self-attention,Knowledge-distillation,Spatial transformers,Pose estimation
AI 理解论文
溯源树
样例
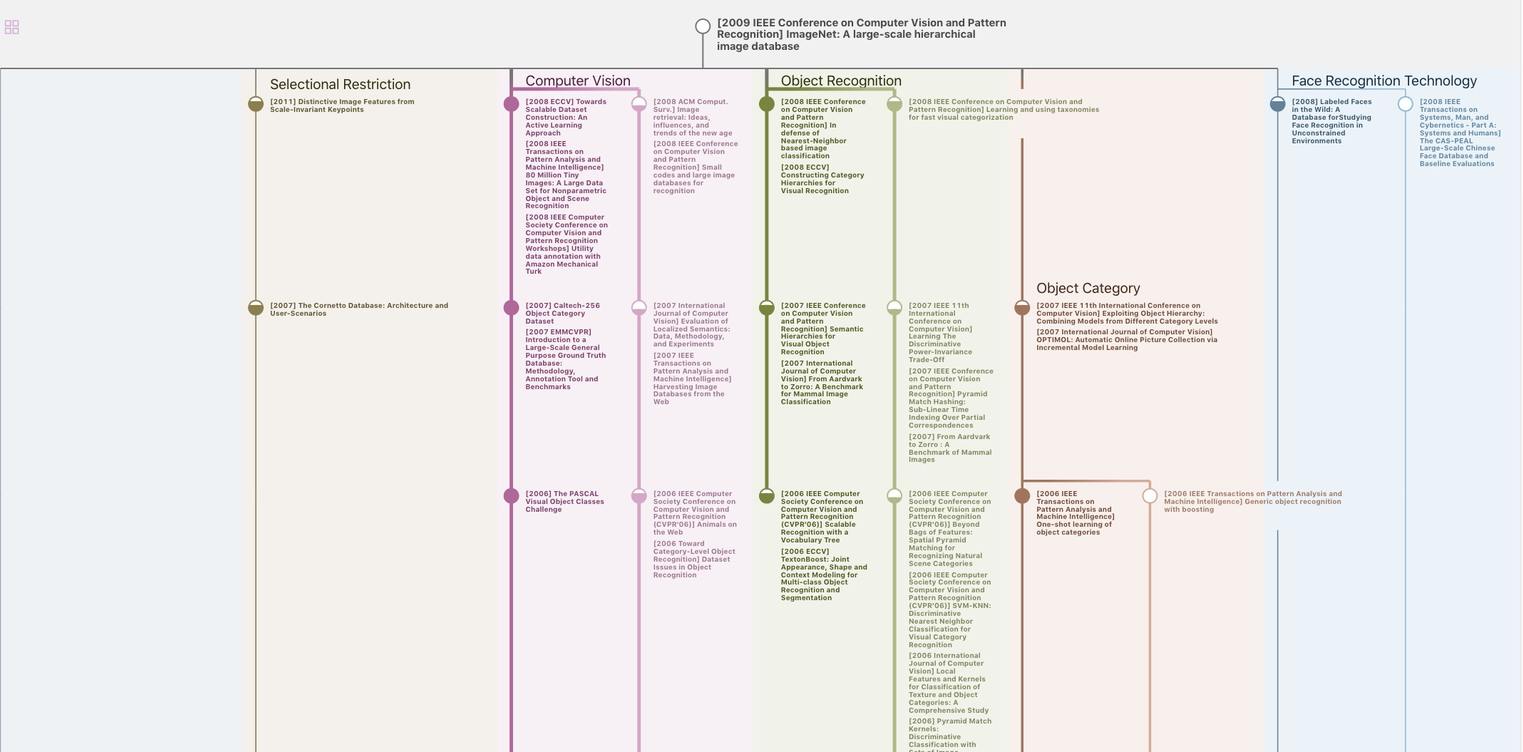
生成溯源树,研究论文发展脉络
Chat Paper
正在生成论文摘要