Dual time-scale state-coupled co-estimation of state of charge, state of health and remaining useful life for lithium-ion batteries via Deep Inter and Intra-Cycle Attention Network
JOURNAL OF ENERGY STORAGE(2024)
摘要
The reliable and safe battery operations demand accurate estimation of battery states in the battery management system (BMS). Due to the complex electrochemical process, it is challenging to directly measure SOH and RUL of batteries for online applications. Moreover, the battery aging can strongly influence SOC estimation. The paper proposes a SOC, SOH, and RUL co-estimation method based on Deep Inter and IntraCycle Attention Network (DIICAN). From BMS data, the dual time-scale inter-cycle and intra-cycle features are automatically extracted with neural networks. The state degradation attention (SDA) unit is employed to recognize the state evolution pattern and evaluate battery degradation degree based on inter-cycle features. In addition, the extracted inter-cycle and intra-cycle features are simultaneously coupled for the SOC estimation to calibrate the capacity degradation. The mean absolute error (MAE) and root mean square error (RMSE) of the state of health (SOH) estimation are 0.30% and 0.43%, respectively. For the remaining useful life (RUL) estimation, the MAE is 178.5, and the RMSE is 230.3. With state coupling, the MAE of the state of charge (SOC) estimation decreases from 2.36% to 1.00%, and the RMSE decreases from 3.27% to 1.36% over the entire lifespan. In summary, the proposed method can not only accurately co-estimate SOH and RUL, but improve SOC estimation accuracy over the entire battery life.
更多查看译文
关键词
Lithium-ion battery,Remaining useful life (RUL),State of health (SOH),State of charge (SOC),Deep learning,Attention mechanism
AI 理解论文
溯源树
样例
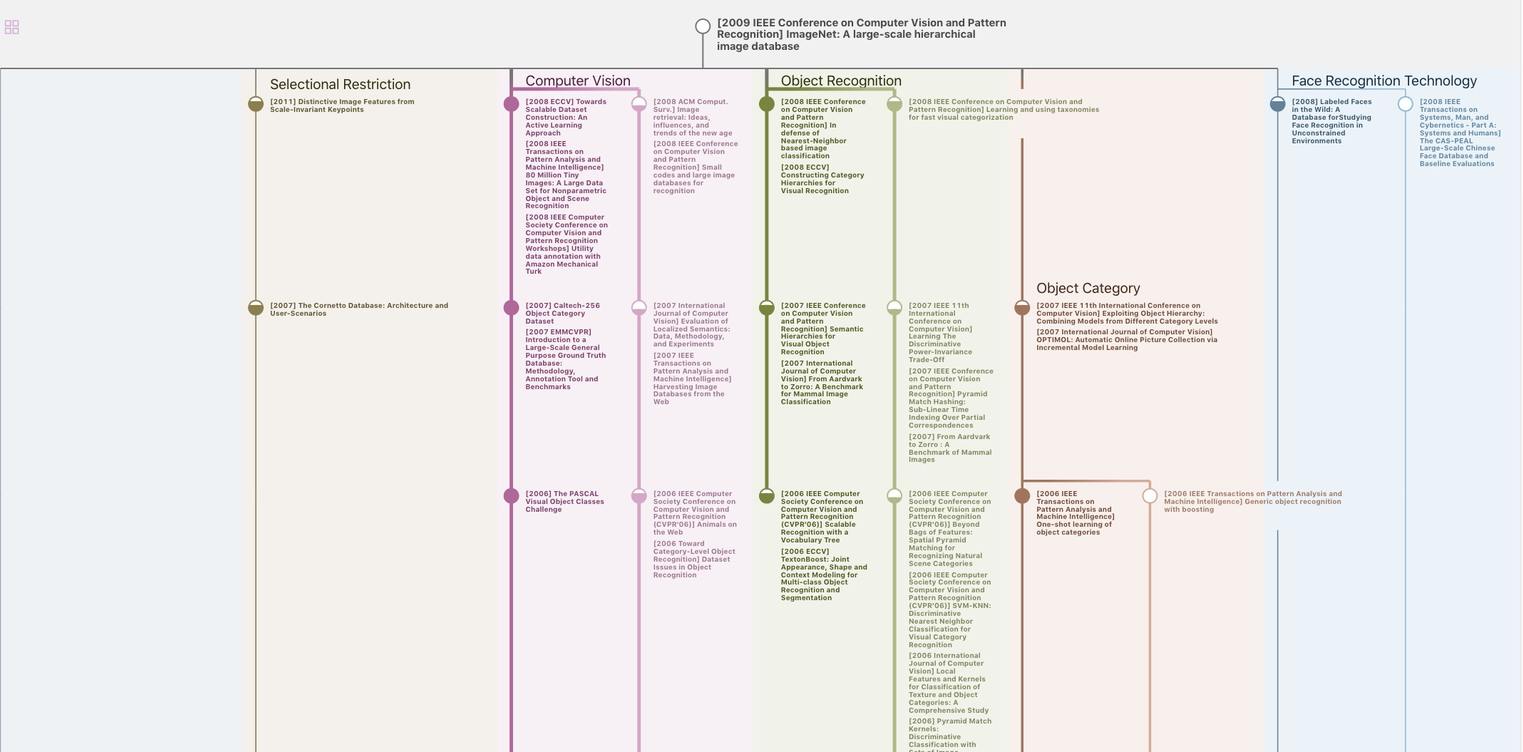
生成溯源树,研究论文发展脉络
Chat Paper
正在生成论文摘要