ICLR: Instance Credibility-Based Label Refinement for label noisy person re-identification
PATTERN RECOGNITION(2024)
摘要
Person re-identification (Re-ID) has demonstrated remarkable performance when trained on accurately annotated data. However, in practical applications, the presence of annotation errors is unavoidable, which can undermine the accuracy and robustness of the Re-ID model training. To address the adverse impacts of label noise, especially in scenarios with limited training samples for each identity (ID), a common approach is to utilize all the available sample labels. Unfortunately, these labels contain incorrect labels, leading to the model being influenced by noise and compromising its performance. In this paper, we propose an Instance Credibility based Label Refinement and Re-weighting (ICLR) framework to exploit partially credible labels to refine and re-weight incredible labels effectively. Specifically, the Label-Incredibility Optimization (LIO) module is proposed to optimize incredible labels before model training, which partitions the samples into credible and incredible samples and propagates credible labels to others. Furthermore, we design an Incredible Instance Re-weight (I2R) strategy, aiming to emphasize instances that contribute more significantly and dynamically adjust the weight of each instance. The proposed method seamlessly reinforces accuracy without requiring additional information or discarding any samples. Extensive experimental results conducted on Market-1501 and Duke-MTMC datasets demonstrate the effectiveness of our proposed method, leading to a substantial improvement in performance under both random noise and pattern noise settings. Code will be available at https://github.com/whut16/ReID-Label-Noise.
更多查看译文
关键词
Person re-identification,Label noise,Label-Incredibility Optimization,Incredible Instance Re-Weight
AI 理解论文
溯源树
样例
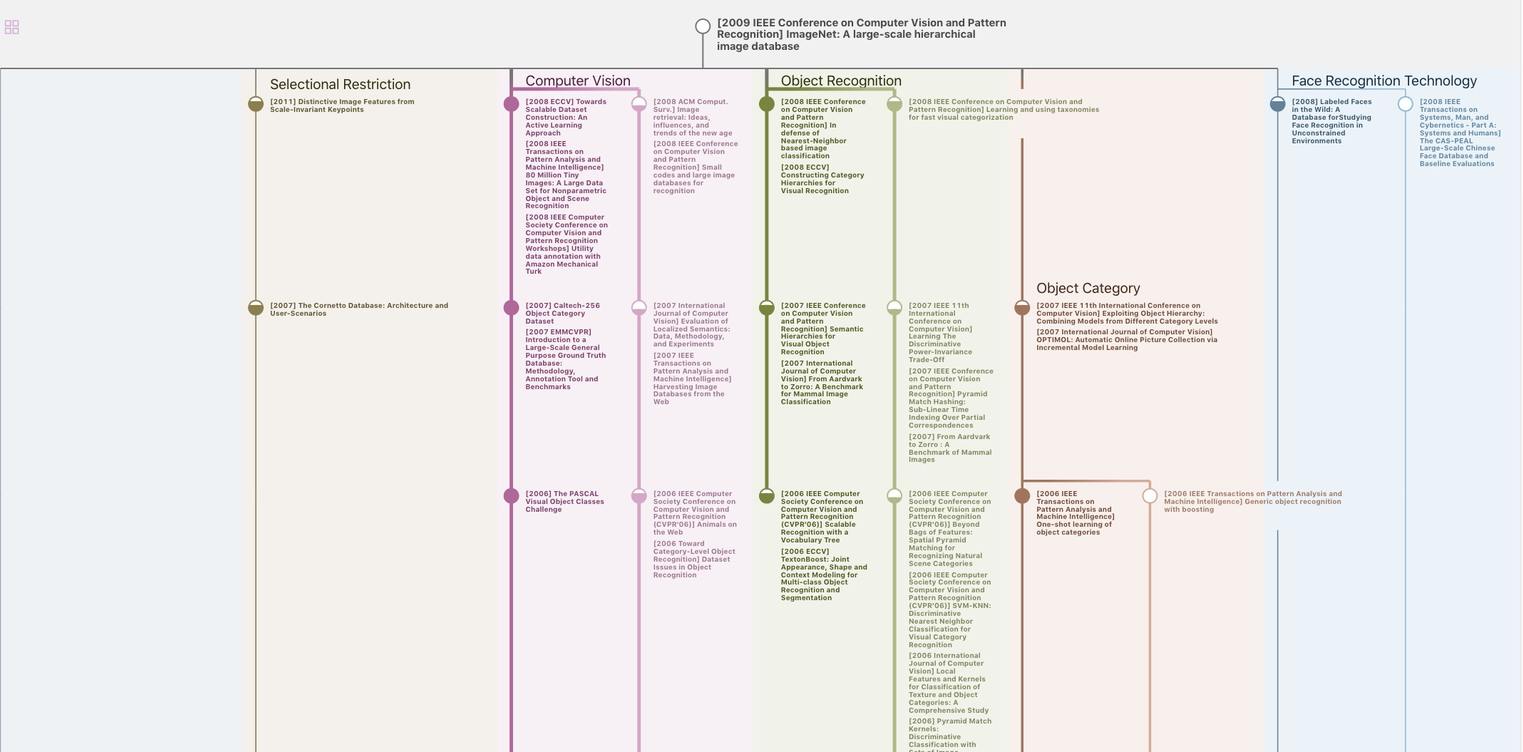
生成溯源树,研究论文发展脉络
Chat Paper
正在生成论文摘要