Unsupervised feature selection by learning exponential weights
PATTERN RECOGNITION(2024)
摘要
Unsupervised feature selection has gained considerable attention for extracting valuable features from unla-beled datasets. Existing approaches typically rely on sparse mapping matrices to preserve local neighborhood structures. However, this strategy favors large-weight features, potentially overlooking smaller yet valuable ones and distorting data distribution and feature structure. Besides, some methods focus on local structure information, failing to explore global information. To address these limitations, we introduce an exponential weighting mechanism to induce a rational feature distribution and explore data structure in the feature subspace. Specifically, we propose a unified framework incorporating local structure learning and exponen-tially weighted sparse regression for optimal feature combinations, preserving global and local information. Experimental results demonstrate the superiority of our approach over existing unsupervised feature selection methods.
更多查看译文
关键词
Unsupervised feature selection,Sparse regression,Local structure learning,Global information preservation
AI 理解论文
溯源树
样例
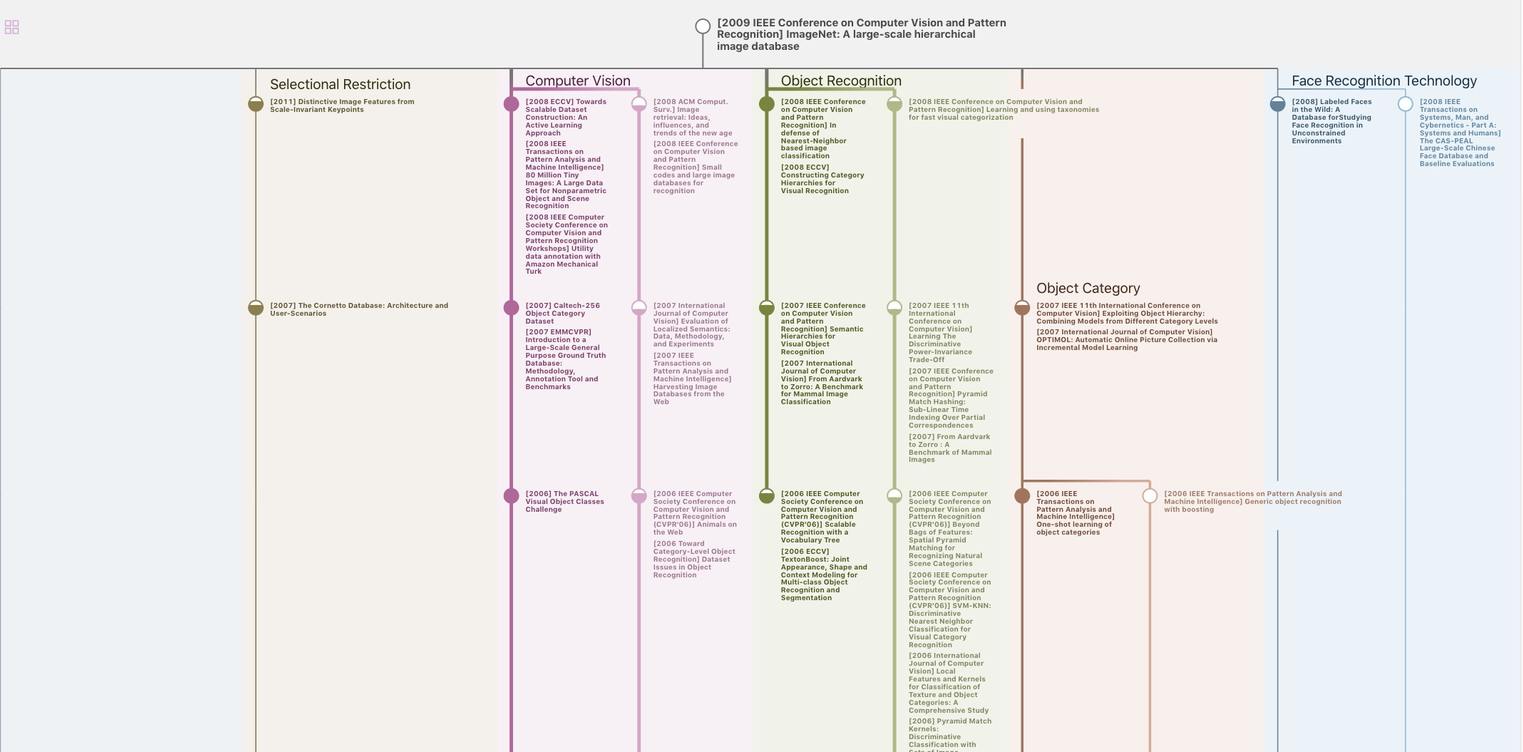
生成溯源树,研究论文发展脉络
Chat Paper
正在生成论文摘要