DFBU-Net: Double-branch flat bottom U-Net for efficient medical image segmentation
BIOMEDICAL SIGNAL PROCESSING AND CONTROL(2024)
摘要
In the field of medical image processing, segmenting tissues and organs in CT/MRI and other medical sequence images is a vital yet challenging task. Analyzing the MICCAI competition, we have identified two problems in current methods for medical image organ segmentation: (1) There is a bottleneck in organ segmentation, with marginal room for improvement, as algorithmic capabilities have already surpassed the task's inherent difficulty. (2) Most current research focuses on stacking and enhancing new modules for segmentation while overlooking the inherent characteristics of medical sequence images. To overcome these two problems, firstly, we have encapsulated the three characteristics of CT/MRI medical sequence image segmentation: semantic correctness, edge accuracy, and 3D structure. Secondly, we delved into the most information-rich downsampling stage in terms of detail and semantics. Subsequently, we designed a flat-bottom double-branch network (DFBU-Net) based on the U-Net architecture. The high-resolution flat bottom branch of this network maintained a 1/4 feature map size to ensure the preservation of rich detail information, while the low-resolution branch underwent progressive downsampling to capture more semantic information. To prevent information loss, cross-fusion was performed at each stage of the model's two branches. Finally, DFBU-Net was evaluated on the MICCAI FLARE2021 dataset (DSC:93.61%, NSD:85.01%). Particularly, in the challenging task of pancreatic segmentation, our model outperformed the first-place model by 0.72% in DSC and 2.92% in NSD. Furthermore, in the MICCAI PARSE2022 competition, DFBU-Net ranked ninth with a DICE score of 79.28%, demonstrating its excellent segmentation performance and generalization ability.
更多查看译文
关键词
U-Net,Medical image segmentation,Medical characteristic,Double-branch
AI 理解论文
溯源树
样例
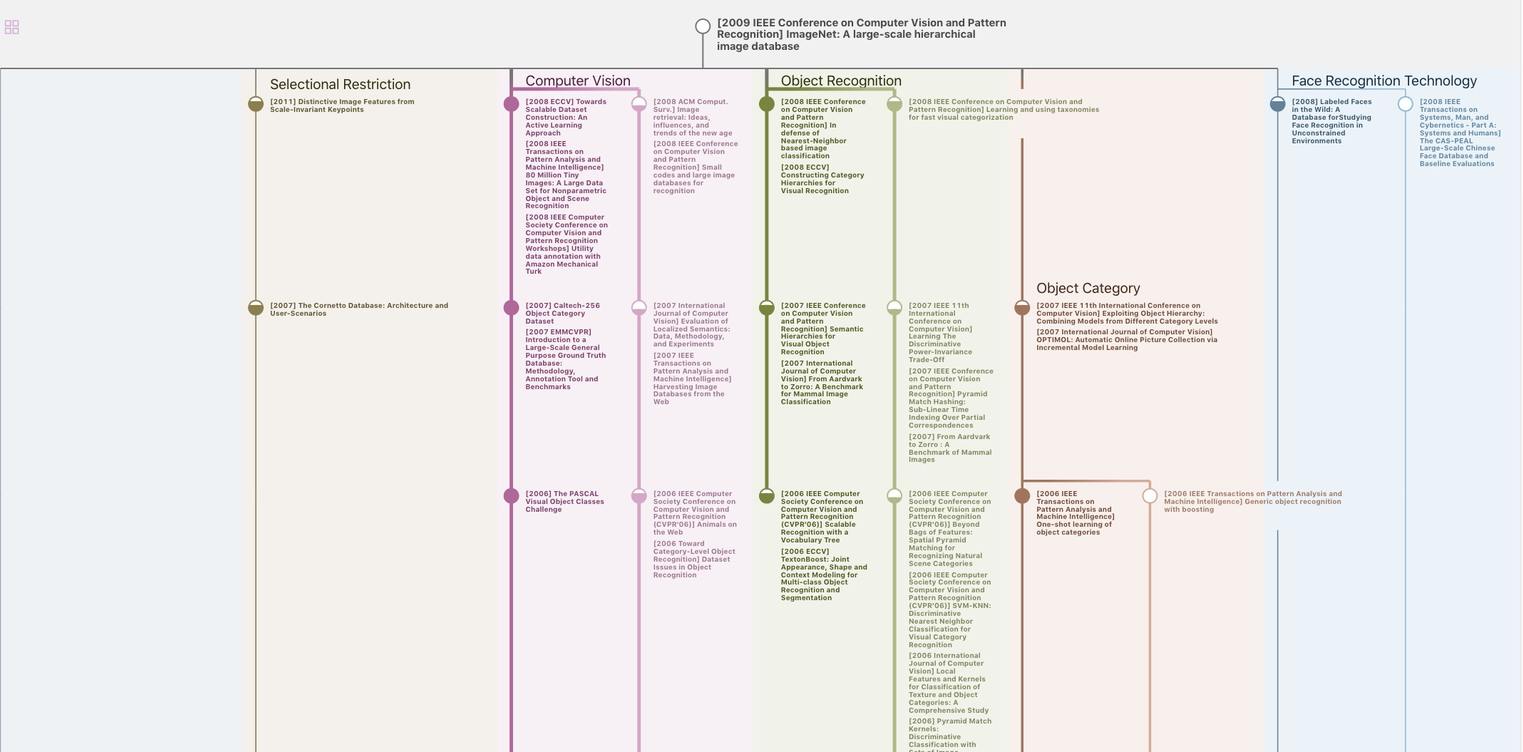
生成溯源树,研究论文发展脉络
Chat Paper
正在生成论文摘要