Super-resolution reconstruction of structured illumination microscopy using deep-learning and sparse deconvolution
OPTICS AND LASERS IN ENGINEERING(2024)
摘要
Structured illumination microscopy (SIM) is one of the essential super-resolution microscopic imaging tech-niques, featuring fast imaging speed, low exposure dose, and having no special requirements for fluorescent probes. In this paper, we propose a super-resolution SIM reconstruction approach, which integrates an end-to -end multi-scale neural network (entitled Scale Richardson-Lucy Network, SRLN). SRLN fits the SIM imaging process through Richardson-Lucy deconvolution incorporated in the neural network. After being trained with sufficient data pairs, among which the ground-truth images were refined with sparse deconvolution, the SRLN network can yield super-resolution images with a spatial resolution of similar to 70 nm from raw SIM intensity images. The feasibility and generalization capability of SRLN were experimentally demonstrated by reconstructing SR images for different biological structures, of which the raw images were acquired with different devices. The proposed network will be valuable for super-resolution imaging in the biology and medicine fields.
更多查看译文
关键词
Structured illumination microscopy,Deep learning,Neural network,Super-resolution,Image reconstruction
AI 理解论文
溯源树
样例
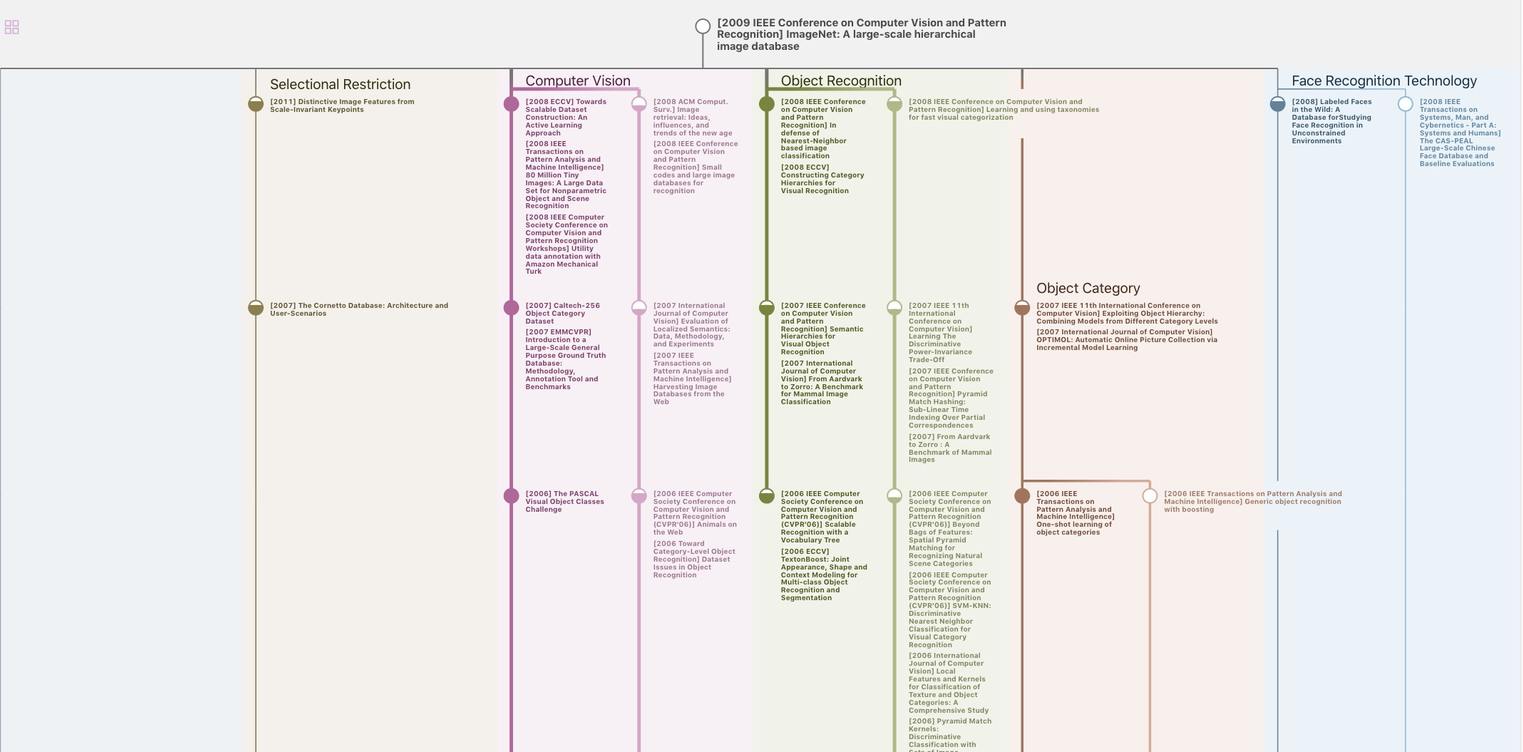
生成溯源树,研究论文发展脉络
Chat Paper
正在生成论文摘要