BGSNet: A cascaded framework of boundary guided semantic for COVID-19 infection segmentation
BIOMEDICAL SIGNAL PROCESSING AND CONTROL(2024)
摘要
Coronavirus disease 2019 (COVID-19) has spread globally in early 2020, leading to a new health crisis. Automatic segmentation of lung infections from computed tomography (CT) images provides an important basis for rapid diagnosis of COVID-19. This paper proposes a cascaded architecture of boundary guided semantic network (BGSNet) based on boundary supervision, multi-scale atrous convolution and dual attention mechanism. The BGSNet cascaded architecture includes a boundary supervision module (BSM), a multi-scale atrous convolution module (MACM), and a dual attention guidance module (DAGM). BSM provides boundary supervised features through explicit modeling to guide precise localization of target regions. MACM introduces atrous convolution with different dilation rates to obtain multi-scale receptive field, thus enhancing the segmentation ability of targets with different scales. DAGM combines channel and spatial attention to filter irrelevant information and enhance feature learning ability. The experimental results based on publicly available CO-Seg and CLSC datasets show that the BGSNet cascaded architecture achieves DSC of 0.806 and 0.677, respectively, which is superior to the advanced COVID-19 infection segmentation model. The effectiveness of the main components of BGSNet has been demonstrated through ablation experiments.
更多查看译文
关键词
COVID-19,CT images,Infection segmentation,Boundary guided semantic
AI 理解论文
溯源树
样例
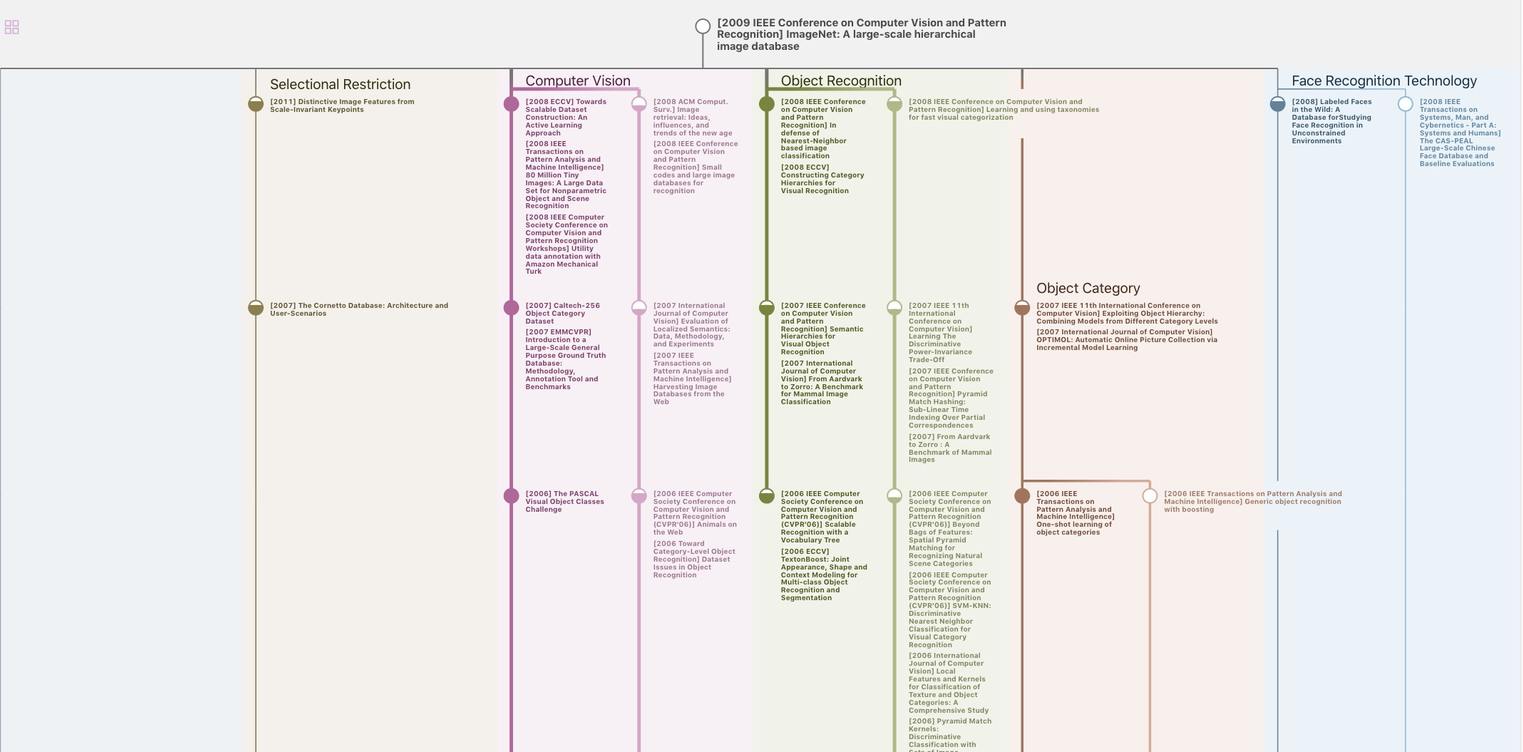
生成溯源树,研究论文发展脉络
Chat Paper
正在生成论文摘要