Diagnosing and grading gastric atrophy and intestinal metaplasia using semi-supervised deep learning on pathological images: development and validation study
Gastric Cancer(2023)
摘要
Patients with gastric atrophy and intestinal metaplasia (IM) were at risk for gastric cancer, necessitating an accurate risk assessment. We aimed to establish and validate a diagnostic approach for gastric biopsy specimens using deep learning and OLGA/OLGIM for individual gastric cancer risk classification. In this study, we prospectively enrolled 545 patients suspected of atrophic gastritis during endoscopy from 13 tertiary hospitals between December 22, 2017, to September 25, 2020, with a total of 2725 whole-slide images (WSIs). Patients were randomly divided into a training set (n = 349), an internal validation set (n = 87), and an external validation set (n = 109). Sixty patients from the external validation set were randomly selected and divided into two groups for an observer study, one with the assistance of algorithm results and the other without. We proposed a semi-supervised deep learning algorithm to diagnose and grade IM and atrophy, and we compared it with the assessments of 10 pathologists. The model’s performance was evaluated based on the area under the curve (AUC), sensitivity, specificity, and weighted kappa value. The algorithm, named GasMIL, was established and demonstrated encouraging performance in diagnosing IM (AUC 0.884, 95
更多查看译文
关键词
Atrophic gastritis,Semi-supervised deep learning,Diagnose,The operative link for gastric intestinal metaplasia assessment,The operative link for gastritis assessment
AI 理解论文
溯源树
样例
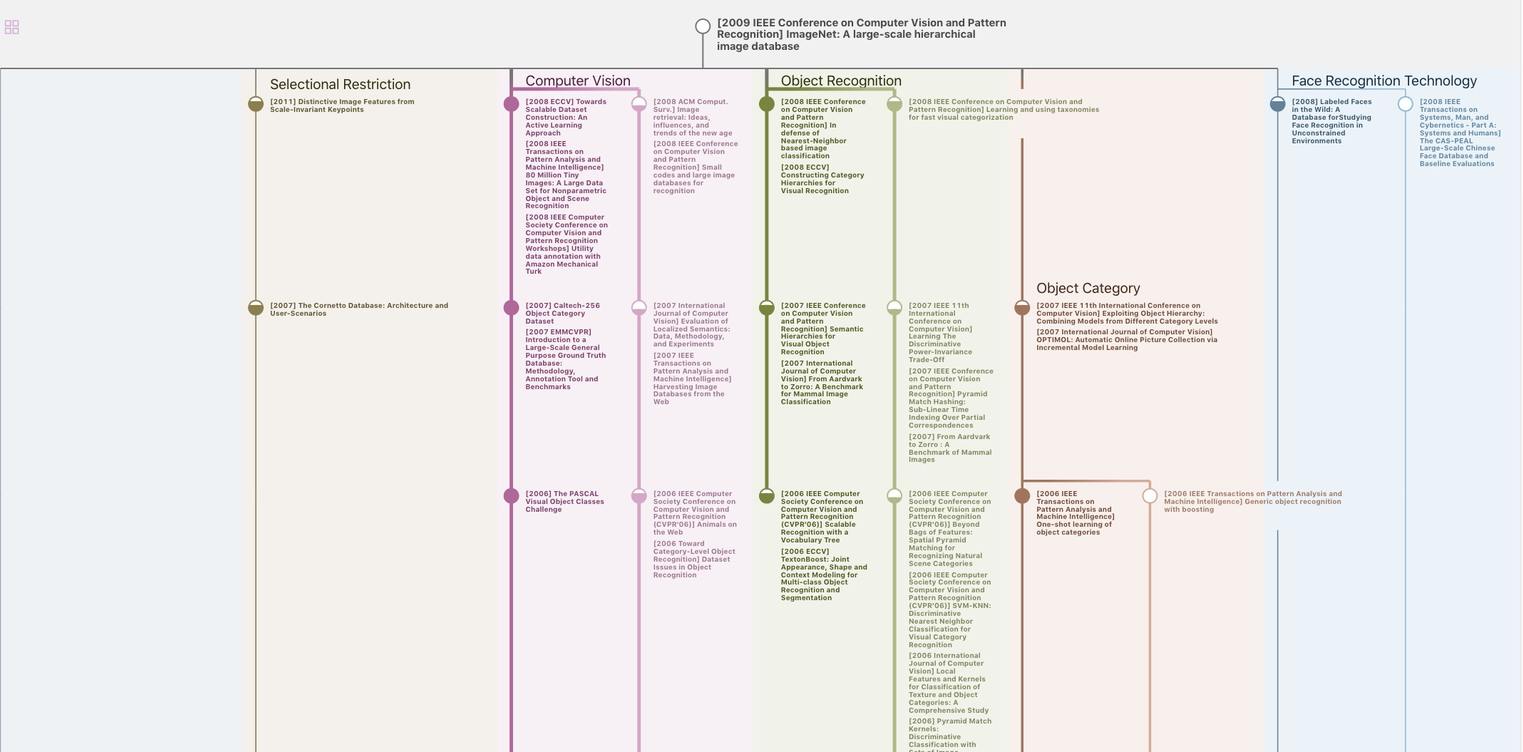
生成溯源树,研究论文发展脉络
Chat Paper
正在生成论文摘要