Generalised 3D Medical Image Registration with Learned Shape Encodings
MEDICAL IMAGE UNDERSTANDING AND ANALYSIS, MIUA 2023(2024)
摘要
Due to the high variability in medical images, task-specific solutions have often prevailed in the field of Deep Learning Image Registration (DLIR). Contrary to classical approaches, these are hardly transferable to other tasks or datasets. To overcome these limitations we propose to exploit easy-to-acquire anatomical segmentations, which provide strong semantic features as a common representation across different datasets and image modalities. We, therefore, present a new module for medical image registration based on generalised label encodings that can be applied to arbitrary label maps. We demonstrate the generalisability of our approach by employing the module trained on skeletal and pulmonary vascular structures to the registration of abdominal segmentations, yielding an average increase in Dice scores of 24% and compare their encoding qualities to classical Euclidean Distance Maps. Furthermore, we leverage the modular design to assess the generalisability of convolutional and vision-transformer based registration architectures. Source code and trained models are released on GitHub.
更多查看译文
关键词
Gerneralisability,Image Registration,Semantic Segmentation
AI 理解论文
溯源树
样例
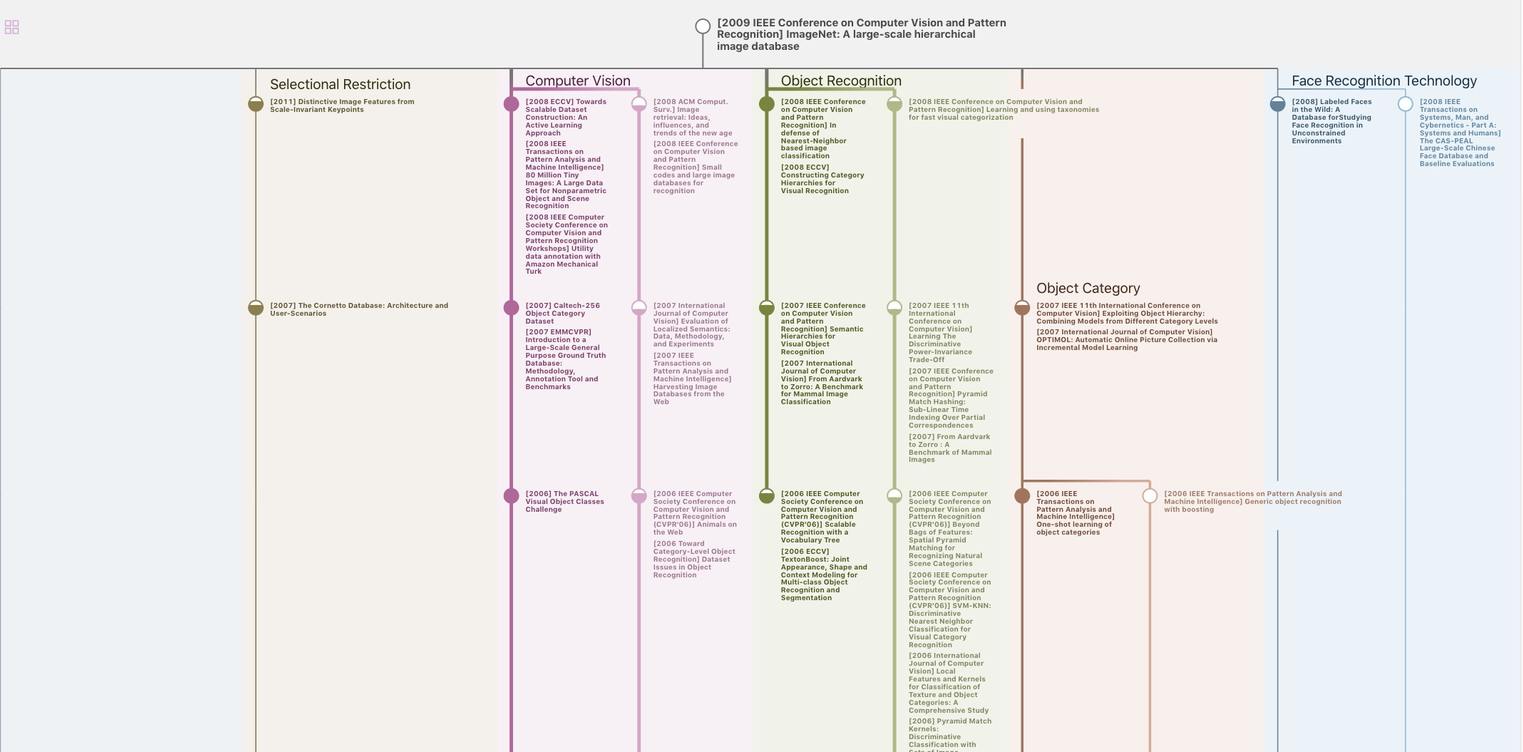
生成溯源树,研究论文发展脉络
Chat Paper
正在生成论文摘要