Leveraging Question Answering for Domain-Agnostic Information Extraction
PROGRESS IN PATTERN RECOGNITION, IMAGE ANALYSIS, COMPUTER VISION, AND APPLICATIONS, CIARP 2023, PT I(2024)
摘要
Transformers gave a considerable boost to Natural Language Processing, but their application to specific scenarios still poses some practical issues. We present an approach for extracting information from technical documents on different domains, with minimal effort. It leverages on generic models for Question Answering and on questions formulated with target properties in mind. These are made to specific sections where the answer, then used as the value for the property, should reside. We further describe how this approach was applied to documents of two very different domains: toxicology and finance. For both, results extracted from a sample of documents were assessed by domain experts, who also provided feedback on the benefits of this approach. F-Scores of 0.73 and 0.90, respectively in the toxicological and financial domain, confirm the potential and flexibility of the approach suggesting that, while it cannot yet be fully automated and replace human work, it can support expert decisions, thus reducing time and manual effort.
更多查看译文
关键词
Information Extraction,Question Answering,Transformers,Toxicology Analysis,Exchange-Traded Fund Information
AI 理解论文
溯源树
样例
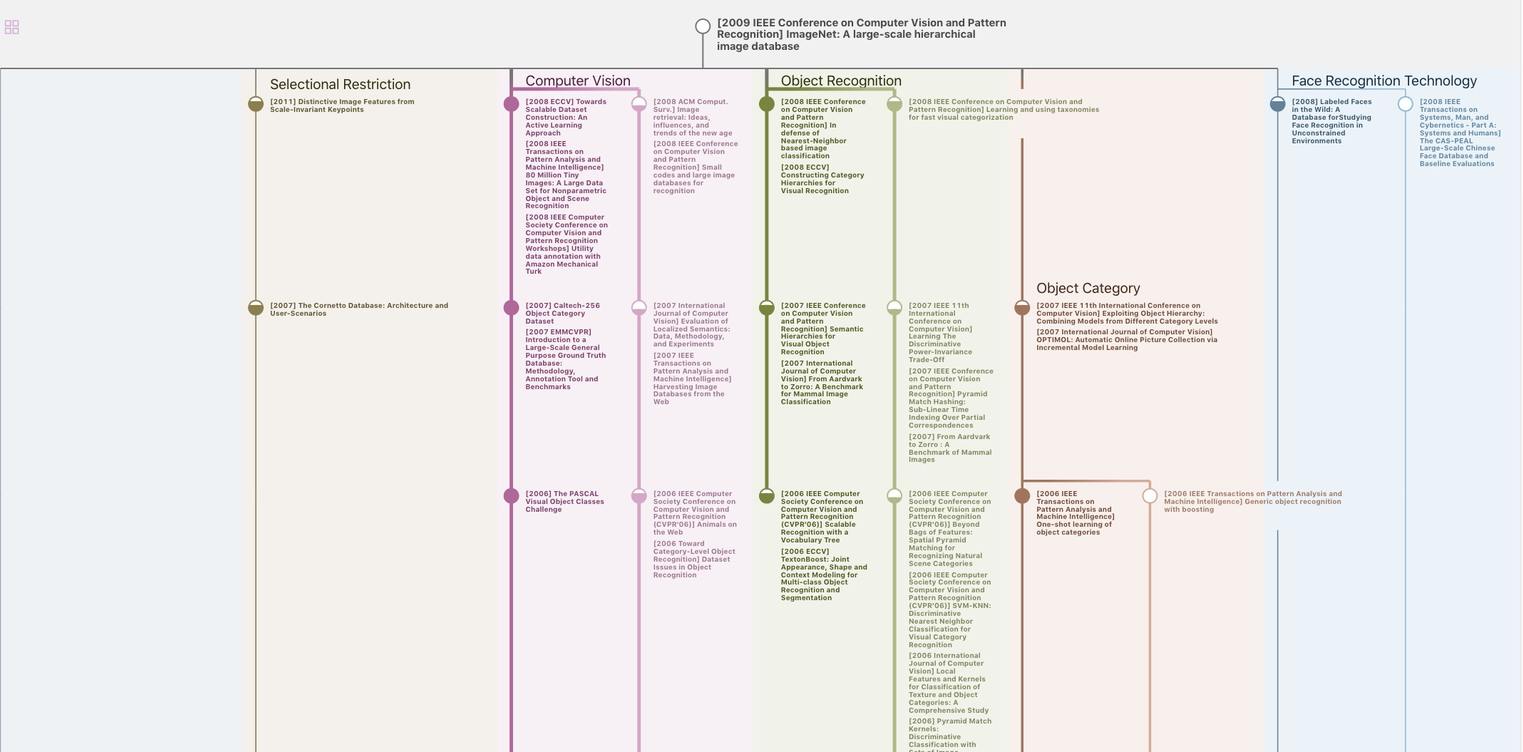
生成溯源树,研究论文发展脉络
Chat Paper
正在生成论文摘要