Uncertainty Quantification of Reservoir Performance Using Machine Learning Algorithms and Structured Expert Judgment
Energy(2024)
摘要
The increasing demand for fossil energy necessitates forecasting of reservoir performance and informed decision-making under various production scenarios. Although reservoir models are used to make such forecasts, neglecting geological uncertainties and history matching can limit the understanding of reservoir production behavior. While performing reservoir simulation on multiple models for uncertainty quantification is a direct approach, it is associated with significant time and computational costs and low convergence rates. This study proposes a method to address these limitations using Bayes' theorem integrated with Machine Learning (ML) algorithms, sampling methods (Markov Chain Monte Carlo and rejection), and Structured Expert Judgment (SEJ) based on the Cooke's Model (CM). The tuned ANN model outperforms traditional simulation methods in terms of computational cost when predicting posterior probability distributions, while also displaying high accuracy as confirmed by R2-score and k-fold cross-validation analyses. Furthermore, using trained ANN models in conjunction with representative samples drawn from posterior probability distributions enables the rigorous prediction of reservoir production parameters. Finally, the CM is implemented to generate a robust model by combining the statistical quantiles, including P10–P25–P50–P75–P90 estimations obtained from MCMC and rejection sampling. The applicability of this methodology is demonstrated for Teal South reservoir with eight uncertain parameters.
更多查看译文
关键词
Uncertainty quantification,Bayes' theorem,Machine learning,Structured expert judgment,Production forecasting
AI 理解论文
溯源树
样例
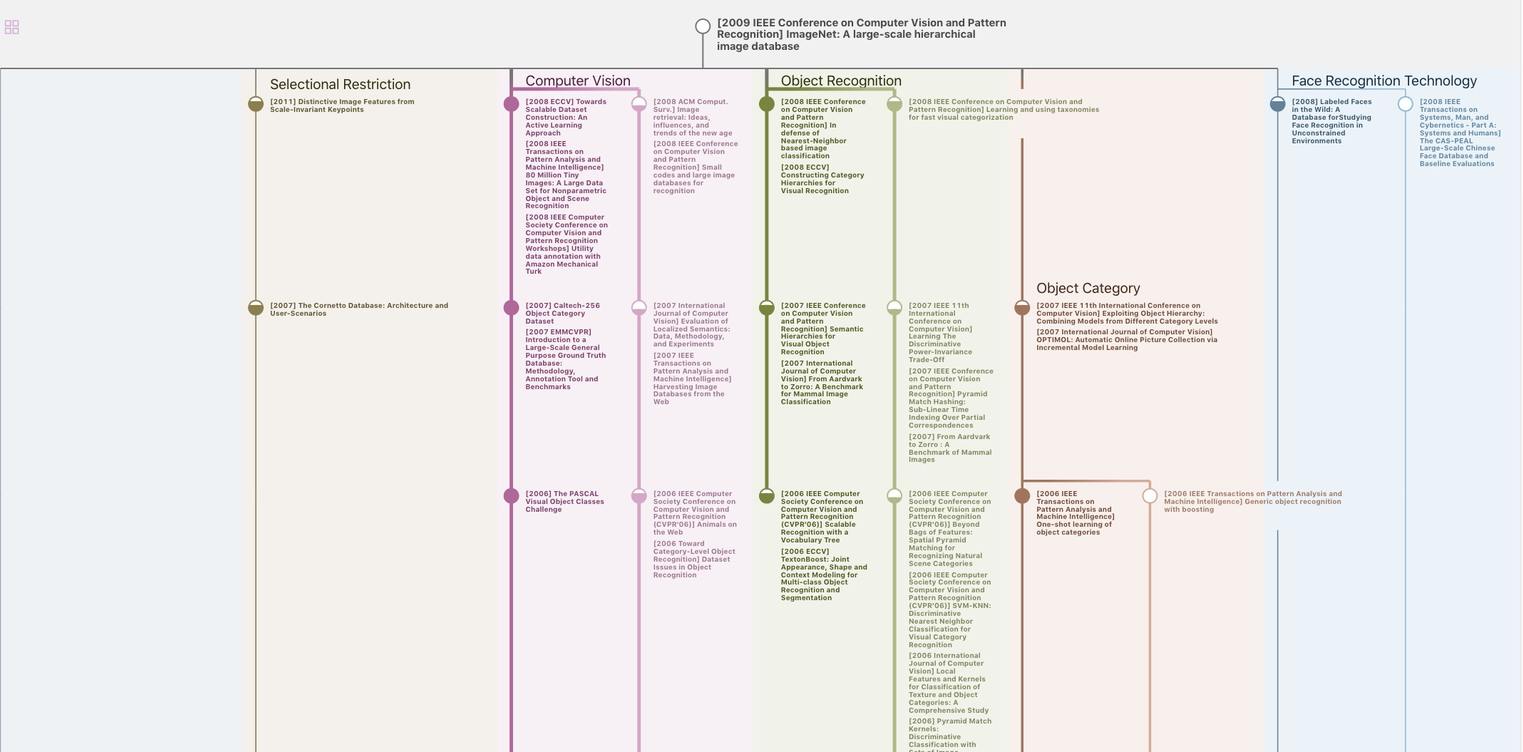
生成溯源树,研究论文发展脉络
Chat Paper
正在生成论文摘要