Adaptive Nonlinear Dimensionality Reduction with a Local Metric.
Symposium on Information and Communication Technology(2023)
摘要
Understanding the structure of multidimensional patterns, especially in unsupervised cases, is of fundamental importance in data mining, pattern recognition and machine learning. Several algorithms have been proposed to analyze the structure of high dimensional data based on the notion of manifold learning. These algorithms have been used to extract the intrinsic characteristics of different types of high dimensional data by performing nonlinear dimensionality reduction. Most of these algorithms rely on the Euclidean metric and manually choosing a neighborhood size. They cannot recover the intrinsic geometry of data manifold automatically and accurately for some data sets. In this paper, we propose an adaptive version of ISOMAP that integrates the advantages of local and global manifold learning algorithms. Faster convergence rate of this algorithm is obtained by replacing the Euclidean metric with the arc length metric as the sum of second-order approximation to the geodesic distance. Our experiments on synthetic data as well as real world images demonstrate that our proposed algorithm can achieve better performance than that of ISOMAP and solve some harder data sets that are impossible for existing global methods.
更多查看译文
AI 理解论文
溯源树
样例
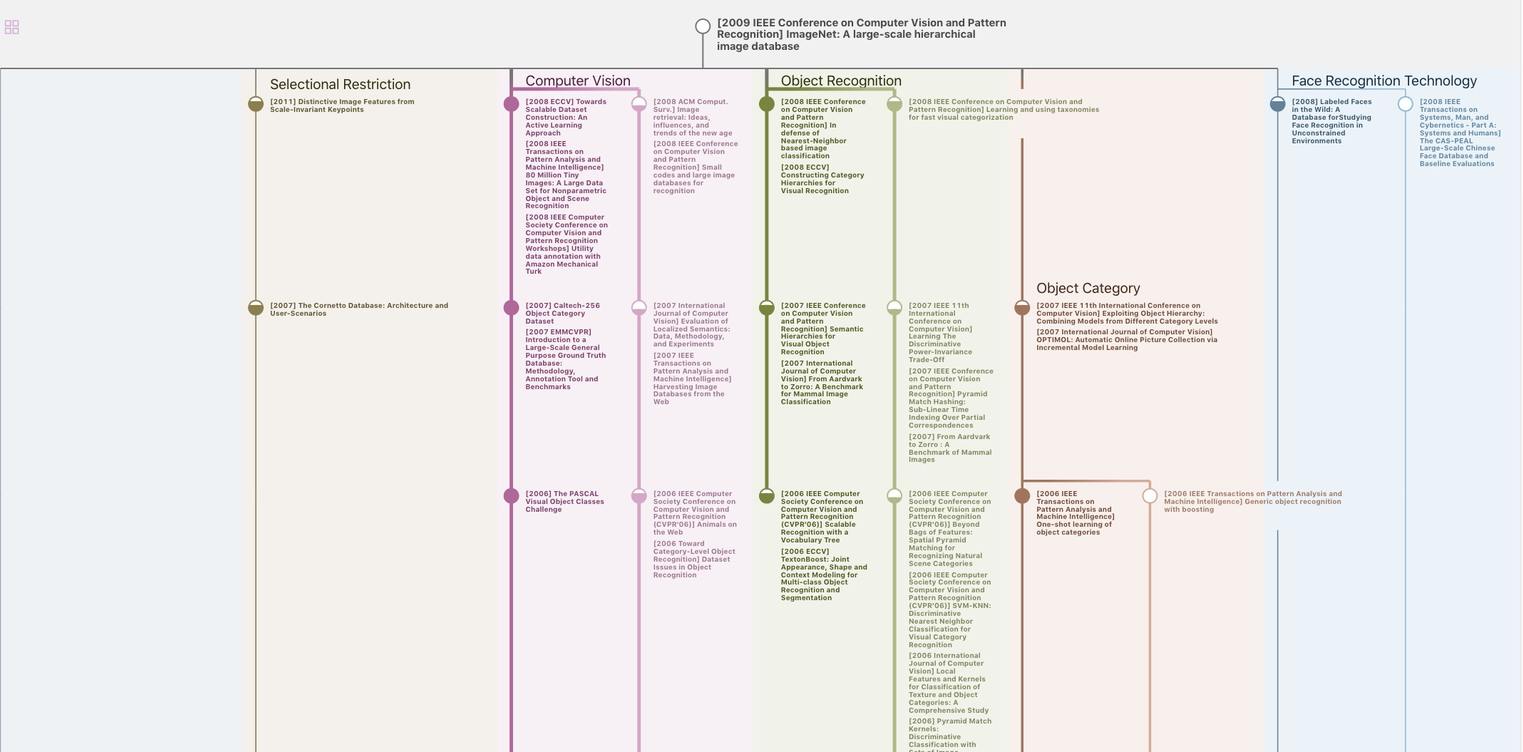
生成溯源树,研究论文发展脉络
Chat Paper
正在生成论文摘要