Latent Space Navigation for Face Privacy: A Case Study on the MNIST Dataset
ADVANCES IN VISUAL COMPUTING, ISVC 2023, PT I(2023)
摘要
Preserving privacy in facial recognition systems while maintaining high accuracy is a challenging problem. In this research, we propose a novel method for achieving image privacy with latent space navigation and synthetic data generation. Our approach aims to generate synthetic samples that are ambiguous to recognize for humans while still being correctly classified by the classifier. To demonstrate the effectiveness of our method, we conduct experiments on the MNIST dataset, chosen for its interpretability and low dimensionality. We create latent spaces with different dimensions (10-D, 30-D, and 50-D) through an encoder-decoder architecture, enabling controlled sampling close to class boundaries. Our optimization technique ensures privacy protection by producing diverse and confusing images that the MNIST digit classifier can correctly identify. The results of our study serve as a foundation for future research in privacy-preserving facial recognition systems, offering a promising direction to safeguard user privacy without compromising classifier accuracy.
更多查看译文
关键词
Latent space walk,Face perturbation,Autoencoder
AI 理解论文
溯源树
样例
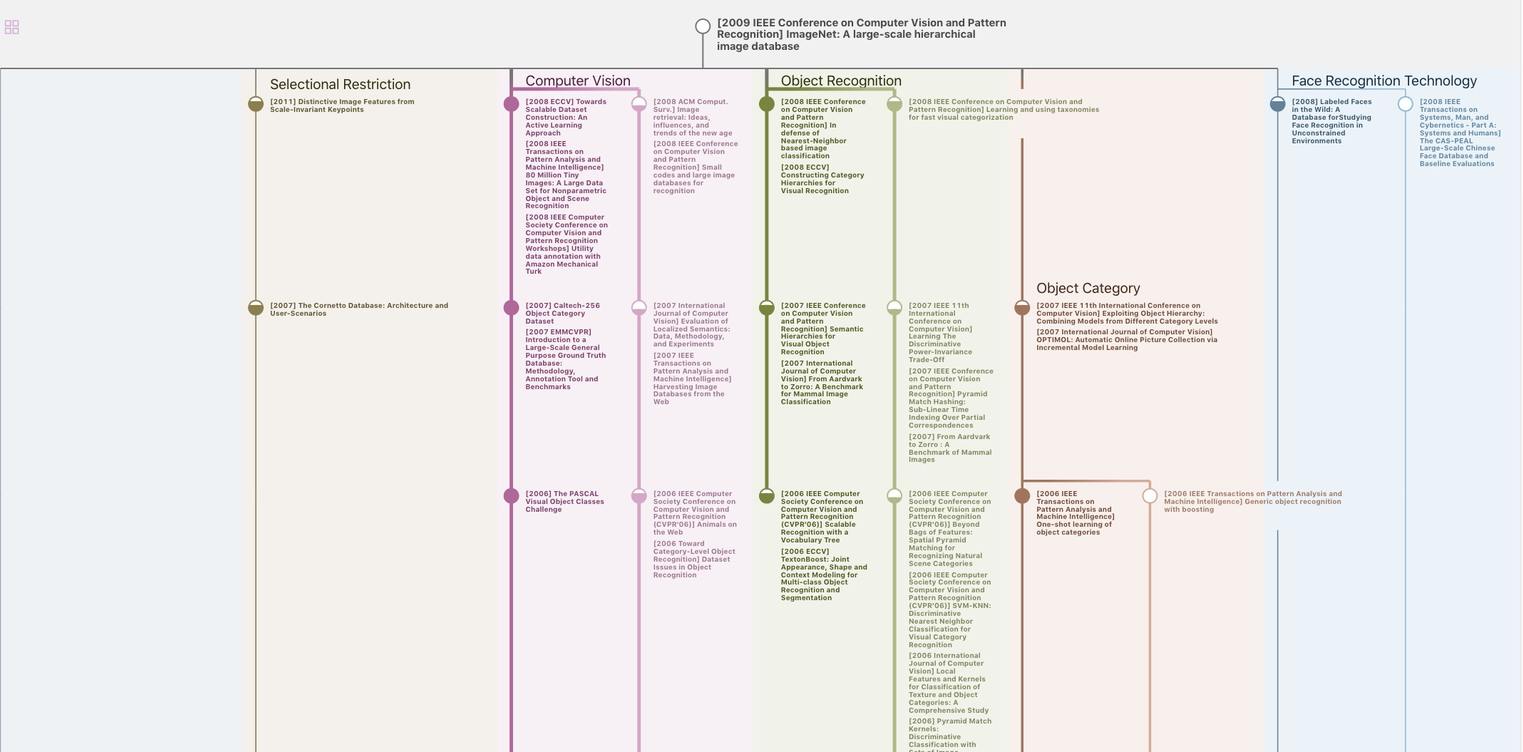
生成溯源树,研究论文发展脉络
Chat Paper
正在生成论文摘要