Bounded exponential loss function based AdaBoost ensemble of OCSVMs
PATTERN RECOGNITION(2024)
摘要
As a commonly used ensemble method, AdaBoost has drawn much consideration in the field of machine learning. However, AdaBoost is highly sensitive to outliers. The performance of AdaBoost may be greatly deteriorated when the training samples are polluted by outliers. For binary and multi-class classifications, there have emerged many approaches to improving the robustness of AdaBoost against outliers. Unfortunately, there are too few researches on enhancing the robustness of AdaBoost against outliers in the case of one class classification. In this study, the exponential loss function of AdaBoost is replaced by a more robust one to improve the anti-outlier ability of the conventional AdaBoost based ensemble of one-class support vector machines (OCSVMs). Furthermore, based on the redesigned loss function, the update formulae for the weights of base classifiers and the probability distribution of training samples are reformulated towards the AdaBoost ensemble of OCSVMs. The empirical error upper bound is derived from the theoretical viewpoint. Experimental outcomes upon the artificial and benchmark data sets show that the presented ensemble approach is more robust against outliers than its related methods.
更多查看译文
关键词
One-class classification,AdaBoost,Exponential loss function,One-class support vector machine,Outliers
AI 理解论文
溯源树
样例
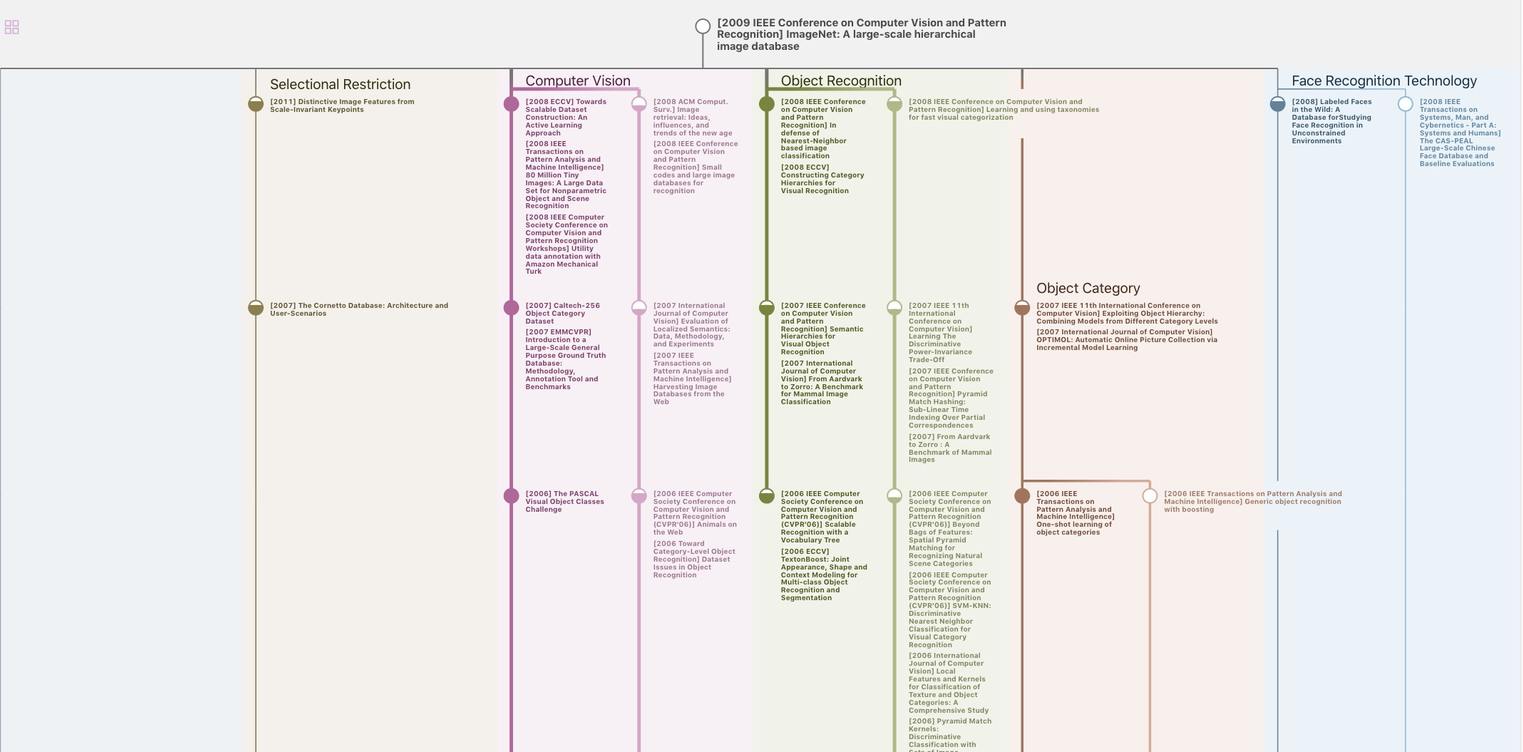
生成溯源树,研究论文发展脉络
Chat Paper
正在生成论文摘要