LAMIS-DMDB: A new full field digital mammography database for breast cancer AI-CAD researches
BIOMEDICAL SIGNAL PROCESSING AND CONTROL(2024)
摘要
The current state of machine learning (ML) and deep learning (DL) shows that they are powerful tools that can be used to glean knowledge from a huge amount of data. While a great deal of research in this area has centered around improving the accuracy and efficiency of training and inference algorithms, there has been relatively little attention paid to the equally important problem of monitoring the quality of data. To tackle this challenge, we have created a cutting-edge breast database capable of employing ML and DL algorithms to detect and classify breast cancer. It is noteworthy that compared to other digital mammogram databases (DMDB), the Laboratory of Mathematics, Informatics and System (LAMIS) images have better resolution and are in full-field digital mammography (FFDM) format. Furthermore, the images can be accessed according to their abnormality (normal, benign, malignant), lesions classification (mass, calcification, architectural distortion, multiple finding), American College of Radiology (ACR) density classification (ACR1, ACR2, ACR3, ACR4) and Breast Imaging Reporting and Data System (BI-RADS). Specifically, the database is designed to provide a variety of metadata as well as clinical data in simple comma separated values (CSV) format that can be really beneficial to the breast cancer research community and to the development of computer-aided diagnosis (CAD(x)) tools that rely on artificial intelligence (AI) algorithms, such as those based on DL models.
更多查看译文
关键词
Breast cancer,Datasets,Deep learning,Machine learning,Breast nodule classification
AI 理解论文
溯源树
样例
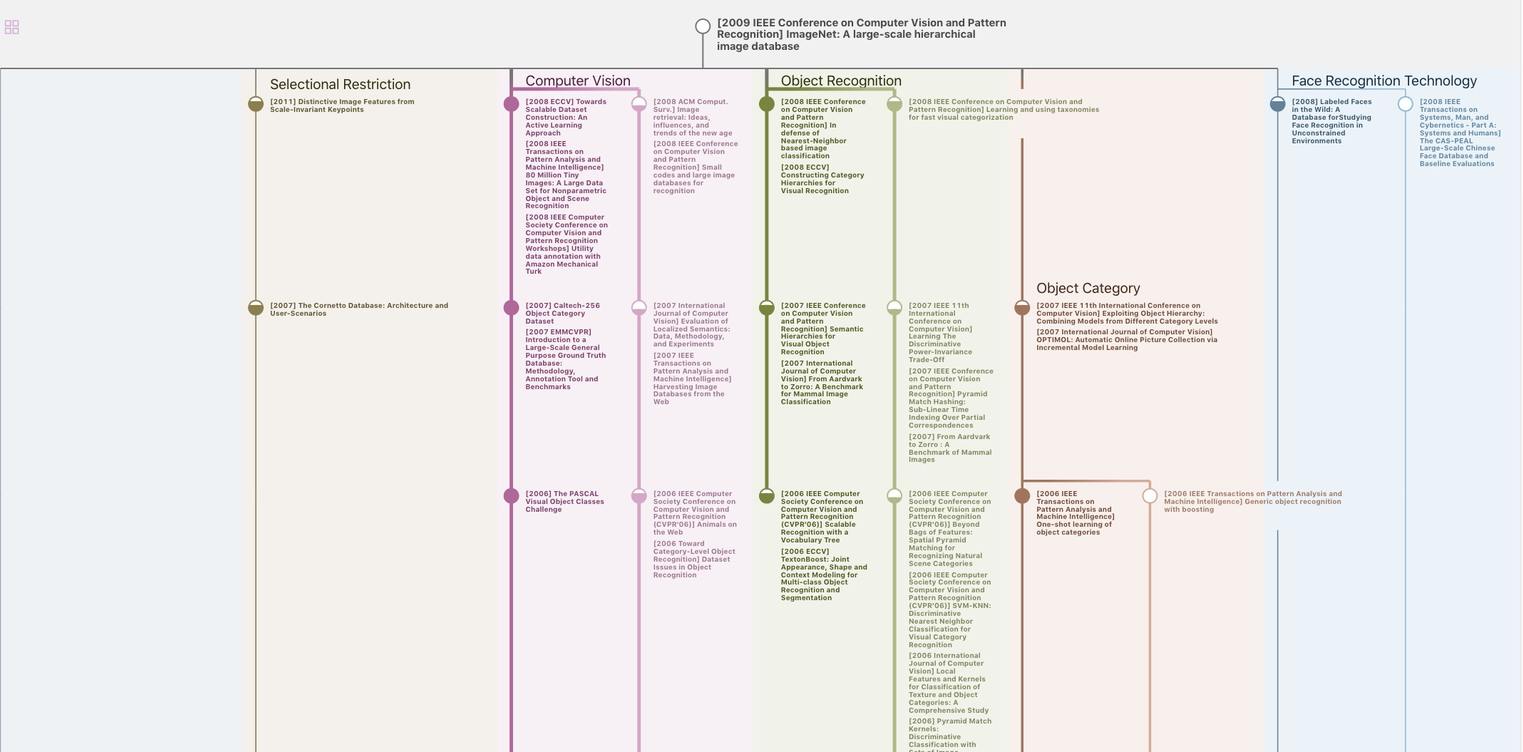
生成溯源树,研究论文发展脉络
Chat Paper
正在生成论文摘要