Optimization of number of wireless temperature sensors using clustering algorithm for deep learning algorithm-based Kimchi quality prediction
JOURNAL OF FOOD ENGINEERING(2024)
摘要
The production and storage of kimchi in a factory require monitoring temperature and humidity to maintain optimal quality. Recently, smart packaging devices such as wireless sensors have been used, but their installation and maintenance are costly and require additional resources. In this study, kimchi is packaged in polyethylene packages and placed in plastic baskets. Thirty baskets are stacked in the middle of the storage room. Wireless sensors placed inside the baskets collect temperature data alongside the kimchi for 60 days. The data is sent to a server through communication unit using 4G network. Two clustering algorithms, Competitive Learning Neural Network (CLNN) and k-means, are used to determine the optimal number and location of sensors. Deep learning algorithms, specifically Long Short-Term Memory (LSTM), predict the acidity and ripeness grade of the kimchi. The study found that the optimal number of temperature sensor groups for CLNN was 6 for acidity and 4 for ripeness grade classification. Increasing the number of sensors improved the kimchi acidity prediction, and the accuracy of ripeness classification increased with the number of sensors. The location of the wireless sensors was determined based on the heights of the stacked plastic baskets using the CLNN + LSTM model. This work pre-sents a process for optimizing sensor placement and evaluating kimchi quality during storage and fermentation using clustering and deep learning techniques to maintain optimal quality.
更多查看译文
关键词
Food fermentation,Kimchi ripeness quality,Optimization,Temperature wireless sensor,Clustering,Deep learning
AI 理解论文
溯源树
样例
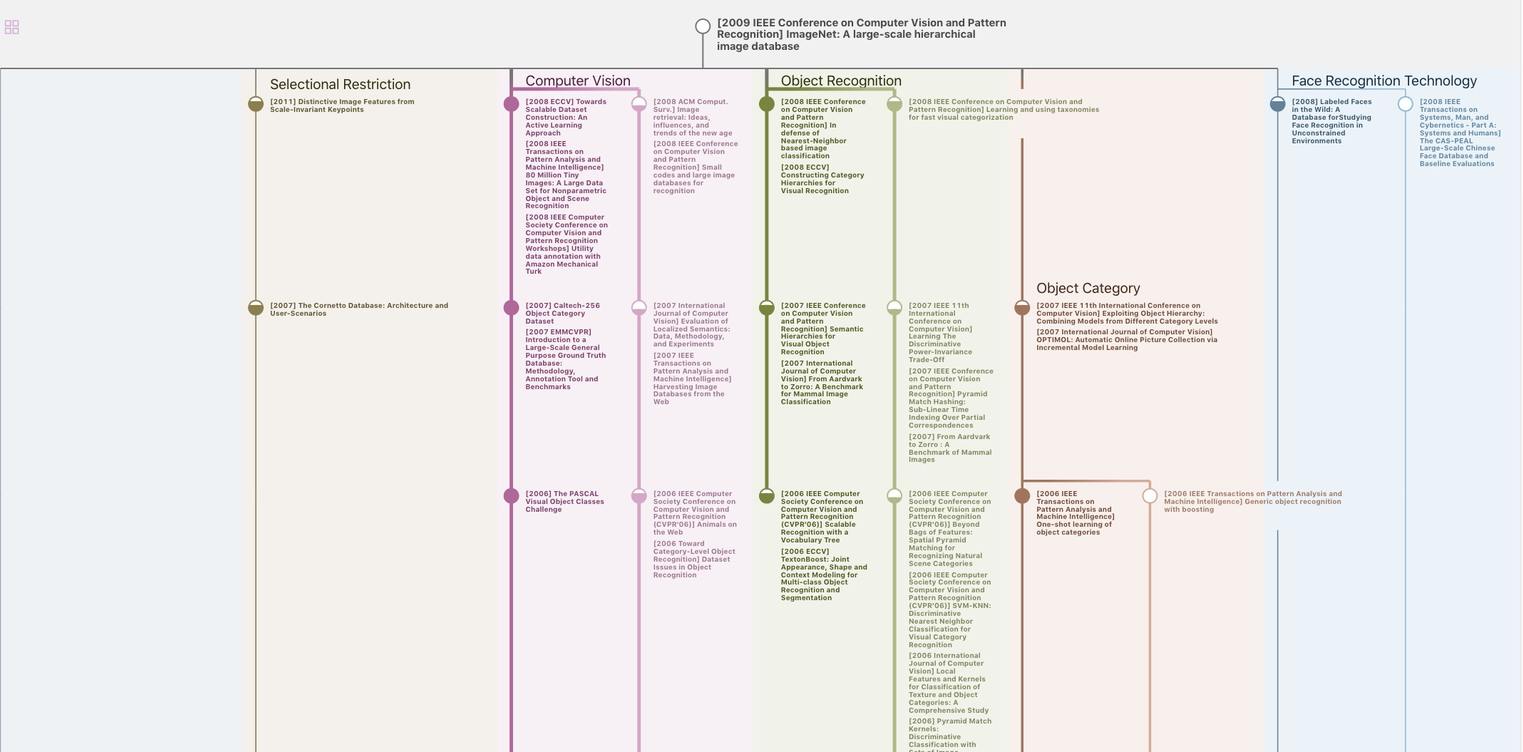
生成溯源树,研究论文发展脉络
Chat Paper
正在生成论文摘要