Adaptive-weighted deep multi-view clustering with uniform scale representation
NEURAL NETWORKS(2024)
摘要
Multi-view clustering has attracted growing attention owing to its powerful capacity of multi-source information integration. Although numerous advanced methods have been proposed in past decades, most of them generally fail to distinguish the unequal importance of multiple views to the clustering task and overlook the scale uniformity of learned latent representation among different views, resulting in blurry physical meaning and suboptimal model performance. To address these issues, in this paper, we propose a joint learning framework, termed Adaptive-weighted deep Multi-view Clustering with Uniform scale representation (AMCU). Specifically, to achieve more reasonable multi-view fusion, we introduce an adaptive weighting strategy, which imposes simplex constraints on heterogeneous views for measuring their varying degrees of contribution to consensus prediction. Such a simple yet effective strategy shows its clear physical meaning for the multi view clustering task. Furthermore, a novel regularizer is incorporated to learn multiple latent representations sharing approximately the same scale, so that the objective for calculating clustering loss cannot be sensitive to the views and thus the entire model training process can be guaranteed to be more stable as well. Through comprehensive experiments on eight popular real-world datasets, we demonstrate that our proposal performs better than several state-of-the-art single-view and multi-view competitors.
更多查看译文
关键词
Multi-view clustering,Deep clustering,Adaptive-weighted learning,Uniform scale representation
AI 理解论文
溯源树
样例
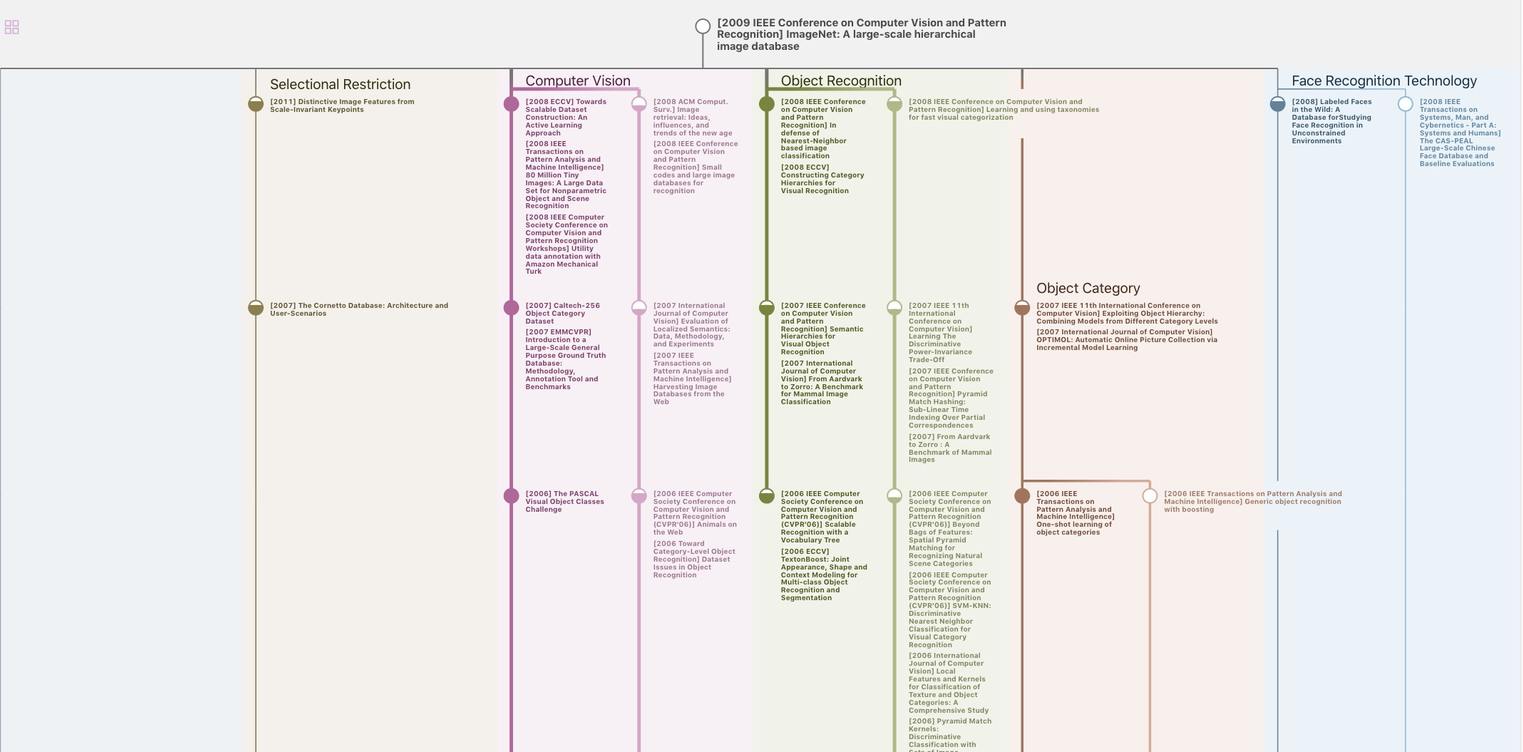
生成溯源树,研究论文发展脉络
Chat Paper
正在生成论文摘要