GraphSAGE with contrastive encoder for efficient fault diagnosis in industrial IoT systems
ICT EXPRESS(2023)
摘要
Imbalanced datasets are common in industrial internet of things (IIoT) systems due to challenges in acquiring faulty labels. Augmentation and graph-based methods have been proposed to improve classification accuracy of deep learning-based systems. However, the conventional approaches can be limited by training complexity and inefficient memory usage. In this paper, GraphSAGE with contrastive encoder (GCE) is proposed to improve classification accuracy and memory utilization efficiency. From the simulation results, it is confirmed that the GCE can improve classification accuracy by up to 23% compared to conventional approaches. (c) 2023 The Authors. Published by Elsevier B.V. on behalf of The Korean Institute of Communications and Information Sciences. This is an open access article under the CC BY-NC-ND license (http://creativecommons.org/licenses/by-nc-nd/4.0/).
更多查看译文
关键词
Imbalanced dataset,Fault diagnosis,Graph neural network,Contrastive learning
AI 理解论文
溯源树
样例
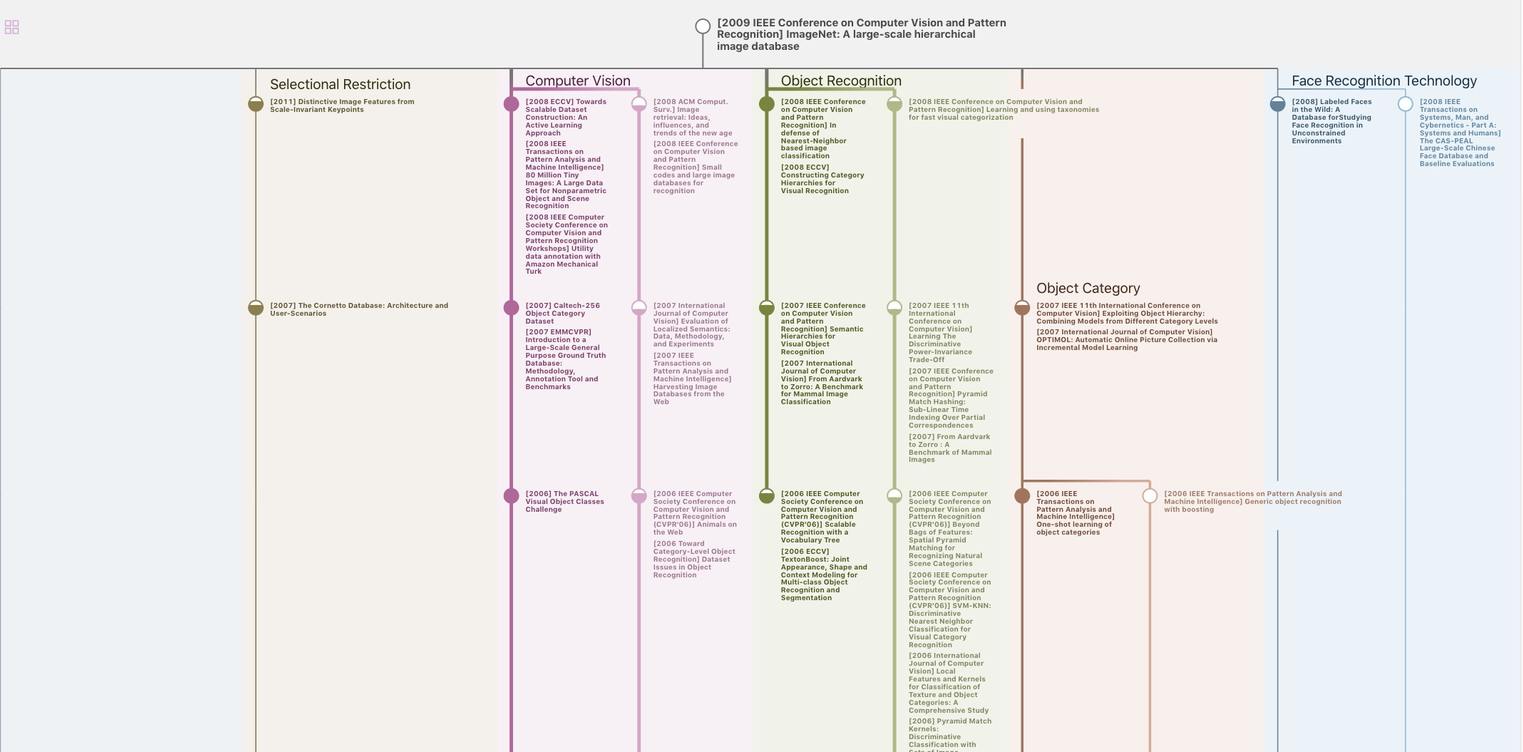
生成溯源树,研究论文发展脉络
Chat Paper
正在生成论文摘要