A novel self-supervised graph model based on counterfactual learning for diversified recommendation
INFORMATION SYSTEMS(2024)
摘要
Consumers' needs present a trend of diversification, which causes the emergence of diversified recommendation systems. However, existing diversified recommendation research mostly focuses on objective function construction rather than on the root cause that limits diversity-namely, imbalanced data distribution. This study considers how to balance data distribution to improve recommendation diversity. We propose a novel selfsupervised graph model based on counterfactual learning (SSG-CL) for diversified recommendation. SSG-CL first distinguishes the dominant and disadvantageous categories for each user based on long-tail theory. It then introduces counterfactual learning to construct an auxiliary view with relatively balanced distribution among the dominant and disadvantageous categories. Next, we conduct contrastive learning between the user-item interaction graph and the auxiliary view as the self-supervised auxiliary task that aims to improve recommendation diversity. Finally, SSG-CL leverages a multitask training strategy to jointly optimize the main accuracy-oriented recommendation task and the self-supervised auxiliary task. Finally, we conduct experimental studies on real-world datasets, and the results indicate good SSG-CL performance in terms of accuracy and diversity.
更多查看译文
关键词
Recommender model,Diversified recommendation,Imbalanced preference distribution,Counterfactual learning,Self -supervised learning
AI 理解论文
溯源树
样例
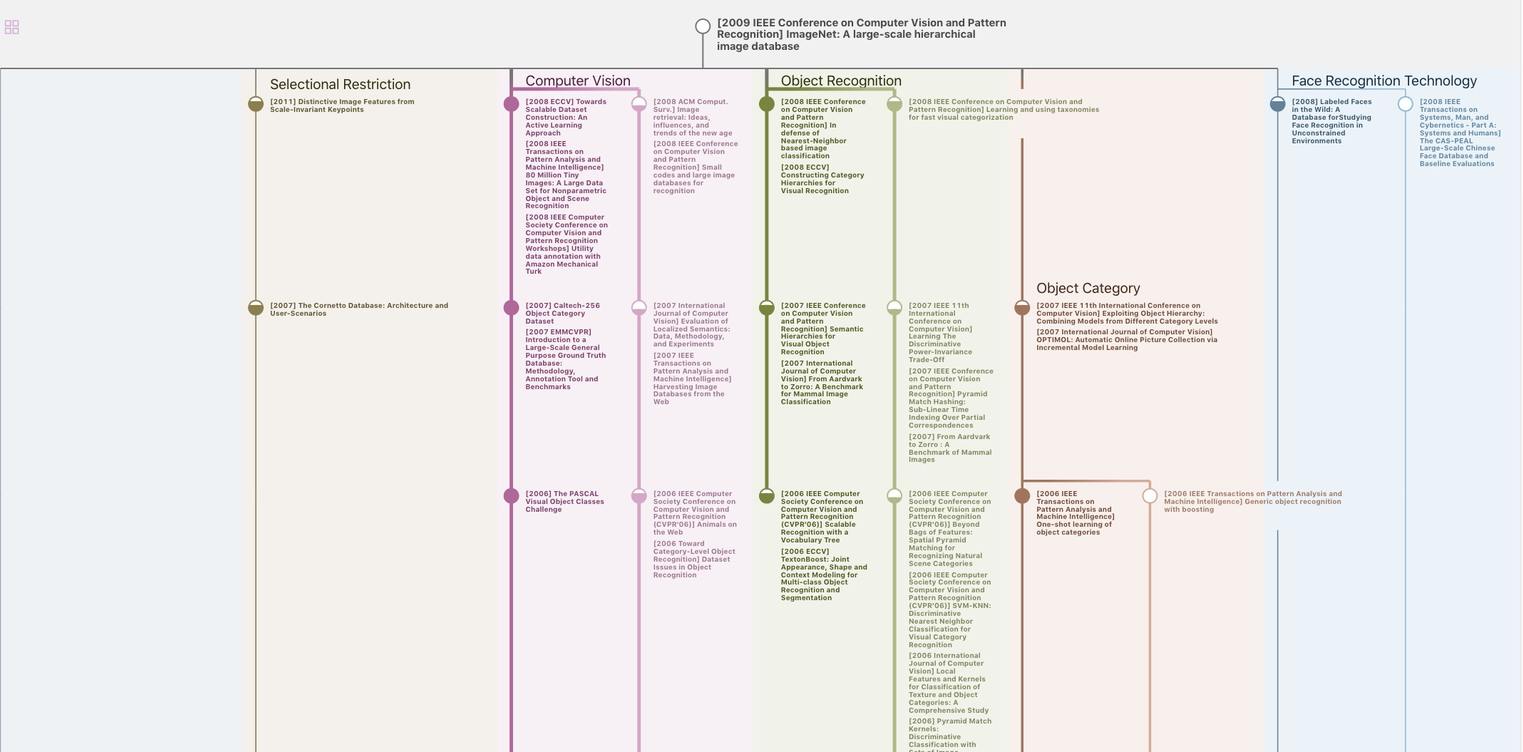
生成溯源树,研究论文发展脉络
Chat Paper
正在生成论文摘要