Microstructural feature-driven machine learning for predicting mechanical tensile strength of laser powder bed fusion (L-PBF) additively manufactured Ti6Al4V alloy
ENGINEERING FRACTURE MECHANICS(2024)
摘要
The rapid solidification inherent in laser powder bed fusion (L-PBF) additive manufacturing (AM) introduces segregation phenomena and formation of non-equilibrium phases in duplex titanium alloy components, thereby impeding their suitability for high-reliability engineering applications. Consequently, heat treatment becomes indispensable for optimizing both the microstructure and mechanical properties to meet application requirements. This study aims to investigate the in-fluence of varied annealing temperatures on the evolution of L-PBF-built Ti6Al4V alloy micro-structure, subsequently elucidating their impact on tensile properties by analyzing of L-PBF process parameters, building orientations, and annealing temperatures. The findings reveal that annealing at 850 degrees C for 2 h facilitates the transformation of brittle martensite into a ductile lamellar (alpha + beta) microstructure, thereby conferring excellent tensile properties upon the L-PBF-built Ti6Al4V alloy. Furthermore, to accurately predict the tensile strengths of the Ti6Al4V, we take into account the L-PBF process parameters and the as-built microstructures in a compre-hensive manner, extracting the pertinent microstructural features. A machine learning (ML)-based model is built to facilitate accurate predictions. Accurate and reliable predictions are demonstrated by this model when applied to Ti6Al4V. This data-driven approach establishes a novel avenue for AM material property prediction and process parameter optimization.
更多查看译文
关键词
Laser powder bed fusion,Additive manufacturing,Annealing,Machine learning,Tensile properties,Ti6Al4V
AI 理解论文
溯源树
样例
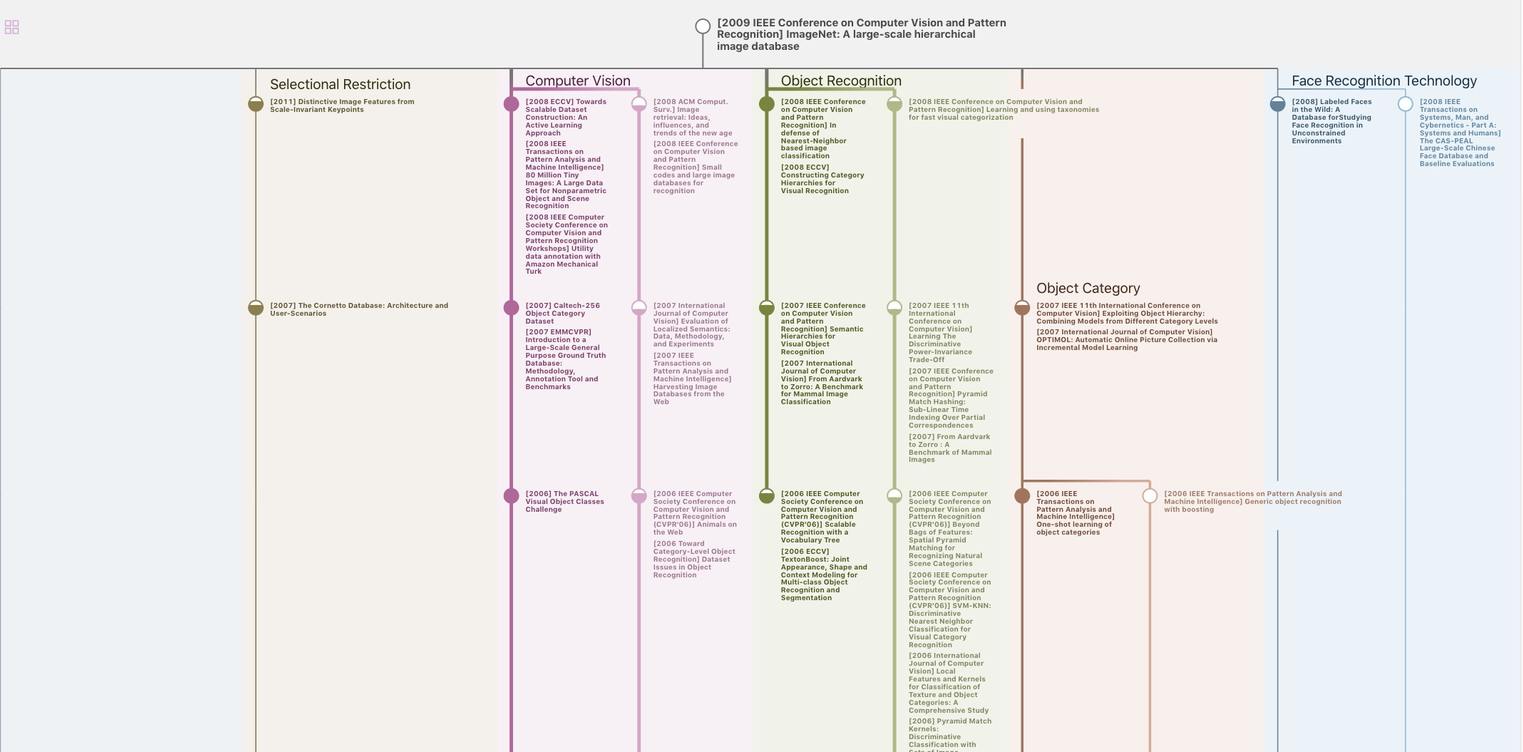
生成溯源树,研究论文发展脉络
Chat Paper
正在生成论文摘要