Predicting Failure Process of Precast Deck Joints Using Physics-Guided LSTM Model
Structures(2024)
摘要
The performance of precast deck joints is critical to the structural response and service life of precast segmental bridges, while the design of joints is faced with problems of high structural complexity and a large number of influencing factors. Meanwhile, current methods for evaluating joint performance are costly and time-consuming. In this research, a novel method for failure process prediction of deck joints is proposed. Based on the bending tests, finite element analysis (FEA) of the joints was performed and verified. Parametric analysis was then conducted to obtain an extensive failure process database. Further, a physics-guided long short-term memory (PG-LSTM) neural network was constructed for accurate sequence-to-sequence prediction of the failure process. Finally, the prediction results of the model were validated by supplementary tests, which showed good agreement. The method proposed in this study combines conventional methods with machine learning techniques to predict the failure process of deck joints and consequently obtain vital indexes such as initial stiffness, ultimate deflection, failure mode, and ultimate bearing capacity, thus offering an effective tool for the design of joints. It can also be extended to the prediction of other structural behaviors, opening up fresh perspectives for the advancement of intelligent design.
更多查看译文
关键词
Precast deck joint,Failure process prediction,Long short-term memory (LSTM),Physics-guided machine learning,Experimental study,Finite element analysis (FEA)
AI 理解论文
溯源树
样例
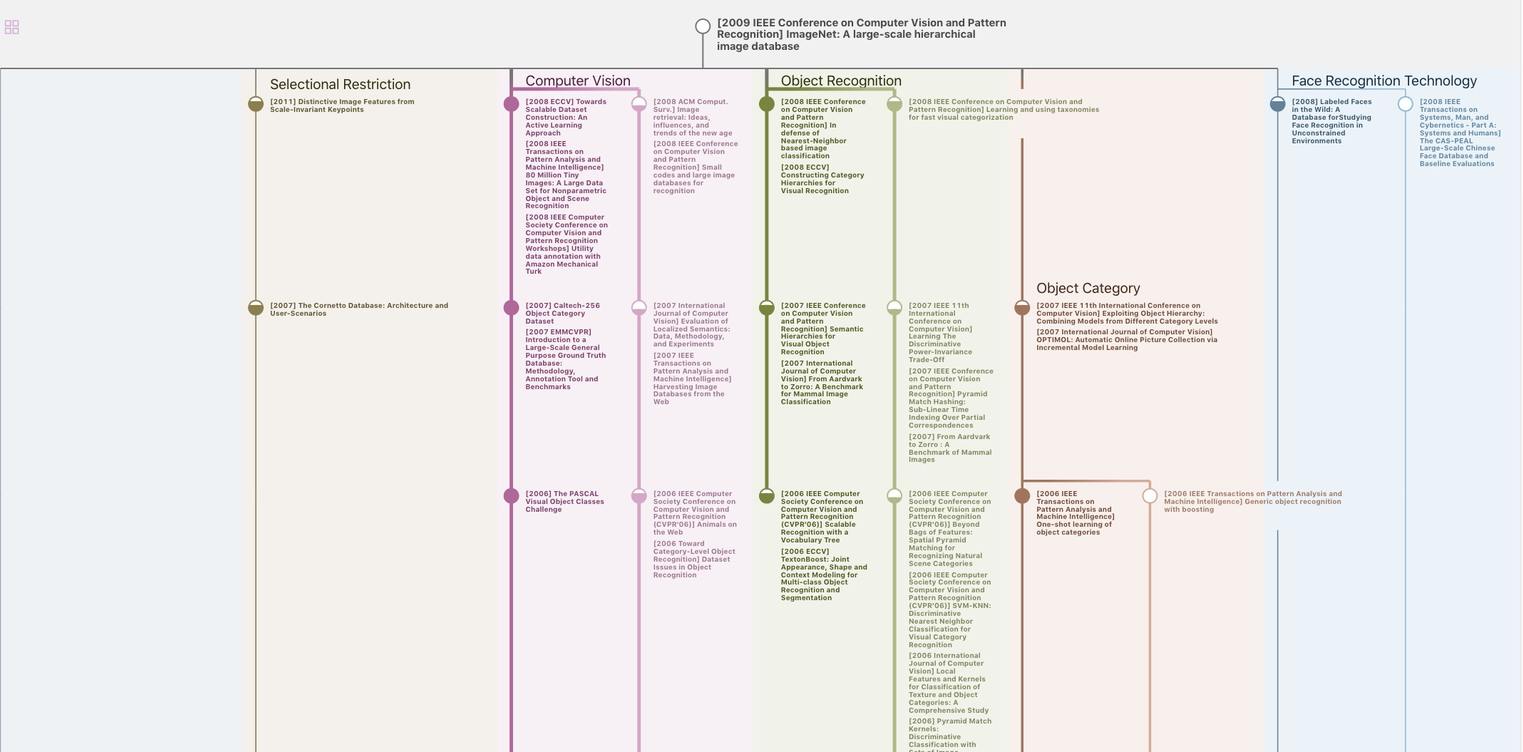
生成溯源树,研究论文发展脉络
Chat Paper
正在生成论文摘要