Mining actionable concepts in concept lattice using Interestingness Propagation
JOURNAL OF COMPUTATIONAL SCIENCE(2024)
摘要
Mining important conceptual patterns is an essential task for understanding the context and content of complex data in many scientific and engineering applications. While exact relevance indices in Formal Concept Analysis provide accurate importance evaluation that can be used for extracting interesting concepts, they often have expensive algorithmic complexity (e.g., at least quadratic in the lattice size, which is exponential with respect to the context size). This frequently results in a computational bottleneck, rendering these indices inappropriate for large, dense contexts or small contexts with large concepts. In this paper, we introduce I nterestingness P ropagation (IP), an efficient message passing strategy for identifying actionable concepts based on interesting and uninteresting information available from a very small portion of the concepts in lattices. From a conceptual perspective, IP leverages the lattice local and global conceptual structures to maintain local and global consistency in the interesting or uninteresting labeling information among similar neighborhood concepts. Experiments on synthetic and real-world datasets show that IP can accurately extract interesting concepts that are very competitive with ground truth ones computed using state-of-the-art exact interestingness indices, while being at least three times faster than certain indices like CR and one order of magnitude faster than others like stability.
更多查看译文
关键词
Pattern mining,Label propagation,Message passing,Interestingness measure,Semi-supervised learning
AI 理解论文
溯源树
样例
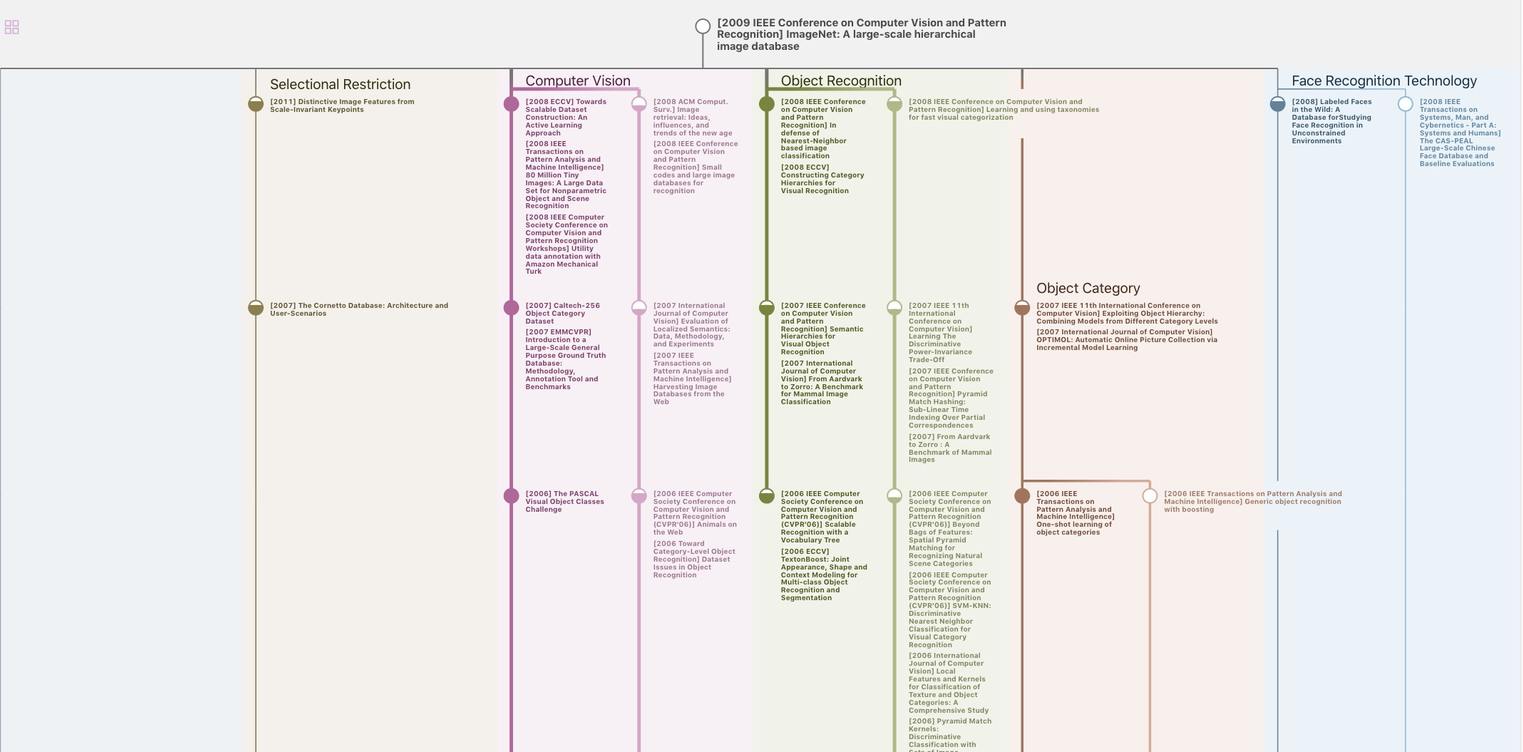
生成溯源树,研究论文发展脉络
Chat Paper
正在生成论文摘要