Intrinsic and extrinsic techniques for quantification uncertainty of an interpretable GRU deep learning model used to predict atmospheric total suspended particulates (TSP) in Zabol, Iran during the dusty period of 120-days wind
ENVIRONMENTAL POLLUTION(2024)
摘要
Total suspended particulates (TSP), as a key pollutant, is a serious threat for air quality, climate, ecosystems and human health. Therefore, measurements, prediction and forecasting of TSP concentrations are necessary to mitigate their negative effects. This study applies the gated recurrent unit (GRU) deep learning model to predict TSP concentrations in Zabol, Iran, during the dust period of the 120-day wind (3 June - 4 October 2014). Three uncertainty quantification (UQ) techniques consisting of the blackbox metamodel, heteroscedastic regression and infinitesimal jackknife were applied to quantify the uncertainty associated with GRU model. Permutation feature importance measure (PFIM), based on the game theory, was employed for the interpretability of the predictive model's outputs. A total of 80 TSP samples were collected and were randomly divided as training (70%) and validation (30%) datasets, while eight variables were used in the TSP prediction model. Our findings showed that GRU performed very well for TSP prediction (with r and Nash Sutcliffe coefficient (NSC) values above 0.99 for both datasets, and RMSE of 57 mu g m(-3) and 73 mu g m(-3) for training and validation datasets, respectively). Among the three UQ techniques, the infinitesimal jackknife was the most accurate one, while all the observed and predicted TSP values fell within the continence limitation estimated by the model. PFIM plots showed that wind speed and air humidity were the most and least important variables, respectively, impacting the predictive model's outputs. This is the first attempt of using an interpretable DL model for TSP prediction modelling, recommending that future research should involve aspects of uncertainty and interpretability of the predictive models. Overall, UQ and interpretability techniques have a key role in reducing the impact of uncertainties during optimization and decision making, resulting in better understanding of sophisticated mechanisms related to the predictive model.
更多查看译文
关键词
Deep learning,GRU model,TSP interpretable model,Game theory,Uncertainty quantification,Zabol
AI 理解论文
溯源树
样例
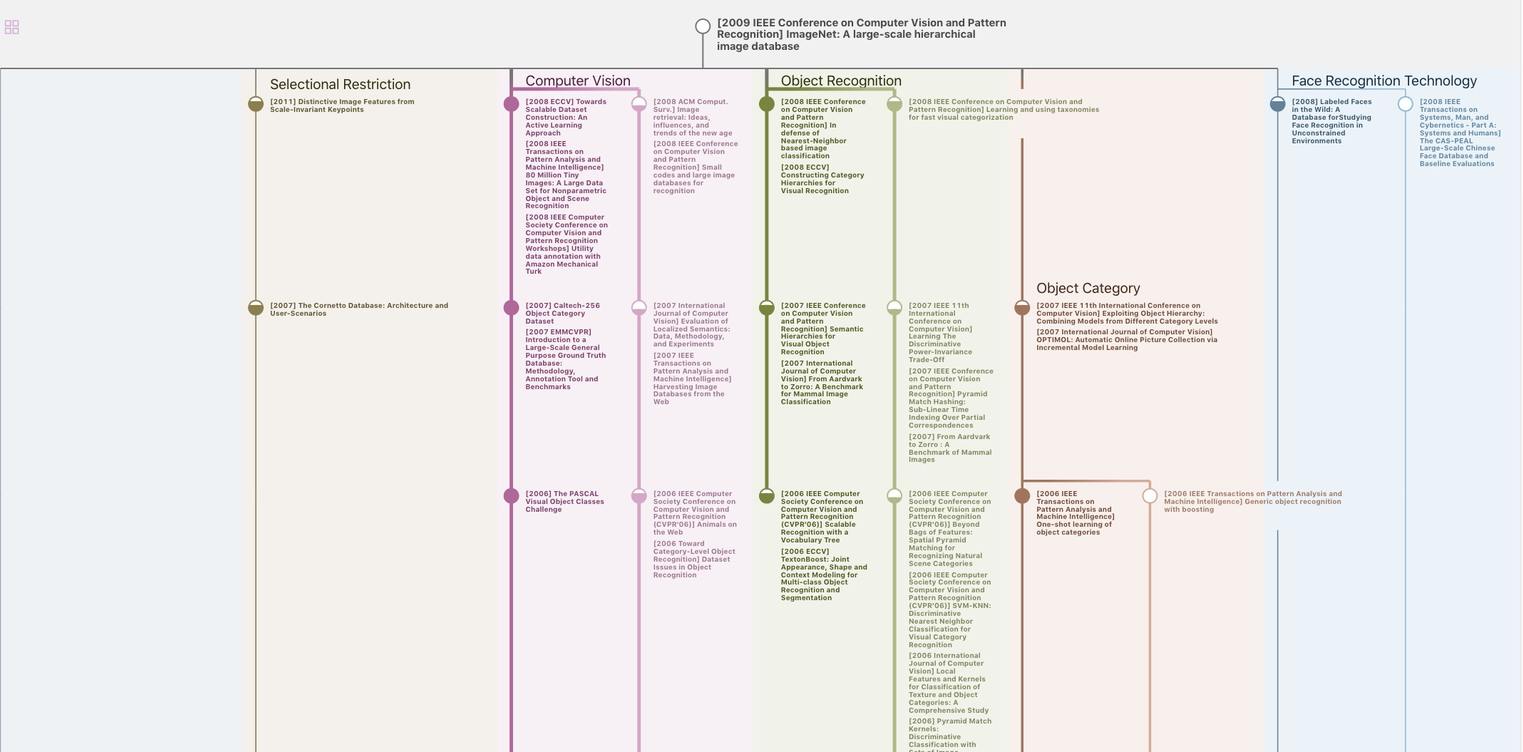
生成溯源树,研究论文发展脉络
Chat Paper
正在生成论文摘要