Graph neural network based robust anomaly detection at service level in SDN driven microservice system
COMPUTER NETWORKS(2024)
摘要
Software-Defined Network (SDN) has been widely employed in data center networks to host various software systems such as microservice systems. A microservice system is constructed by a microservice architecture composed of dozens of services running in different lightweight virtual machines (e.g., containers) and relying on overlay networks for connectivity. However, with the increase of the system scale and the network traffic volume, the network becomes more complex and common to behave anomalously. Therefore, it is error-prone to manually investigate anomalies. To tackle this problem, we propose a non-intrusive monitoring system to help network operators obtain deep insights from the network traffic generated by the running system. Based on the monitoring system, we propose an anomaly detection method to automatically detect network anomalies. The core idea is to learn behavior patterns of inter/intra-components by modeling spatial-temporal relationships amongst collected network metrics with the proposed model STCell-VAE. The pattern will be leveraged to reconstruct the input data. Subsequently, the reconstruction probability is used to determine anomalies. Additionally, STCell-VAE can assimilate network information from new components as the network evolves, eliminating the necessity for retraining. Experiments are conducted within a real networking system and four simulated large-scale networks. The results demonstrate that our system can detect anomalies with about 0.7 F1-score, which outperforms state-of-the-art methods.
更多查看译文
关键词
Software defined network,Microservice,Overlay network,Anomaly detection,Deep learning
AI 理解论文
溯源树
样例
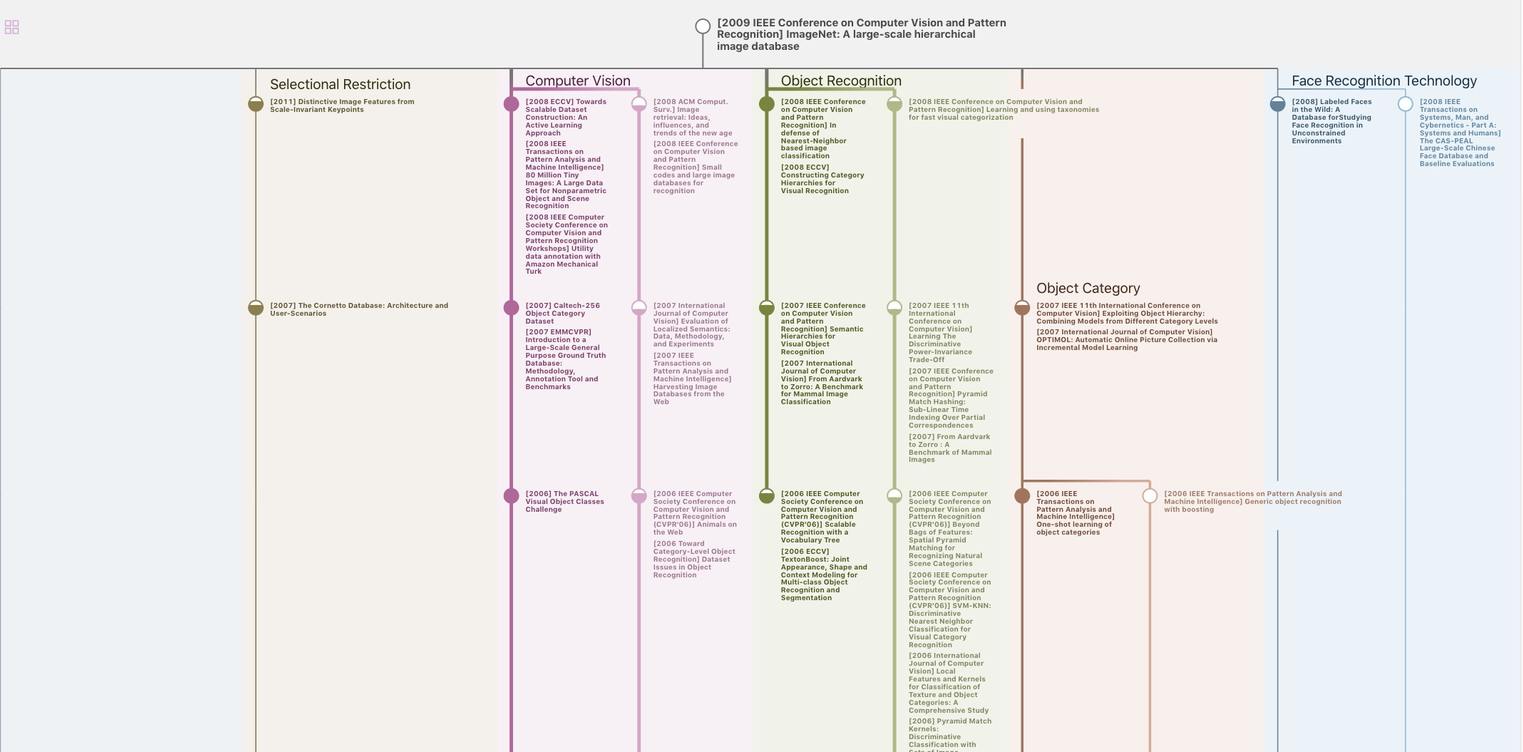
生成溯源树,研究论文发展脉络
Chat Paper
正在生成论文摘要