YOLO-Q: Drone Aerial Target Detection
2023 IEEE 4th International Conference on Pattern Recognition and Machine Learning (PRML)(2023)
摘要
Currently, with the widespread use of UAVs in various fields, there is a significant challenge in detecting aerial targets using drones. The YOLO series of object detection has made significant advancements in both speed and accuracy. However, many state-of-the-art methods are not suitable for drone images due to the unique perspective and large number of small targets. Increasing the detection layer or input picture size can improve accuracy, but this also increases computational cost and reduces detection speed. This paper proposes YOLOQ for aerial object detection, utilizing new S-FPN, SPPF+, and S-SIoU modules. Extensive experiments demonstrate that YOLOQ achieves advanced performance on generic drone dataset with only 5.1MB weight files, two million parameters, 13.5GFLOPs of computational complexity, and 192FPS speed.
更多查看译文
关键词
Computer vision,Object detection,Small targets,Drone aerial photography
AI 理解论文
溯源树
样例
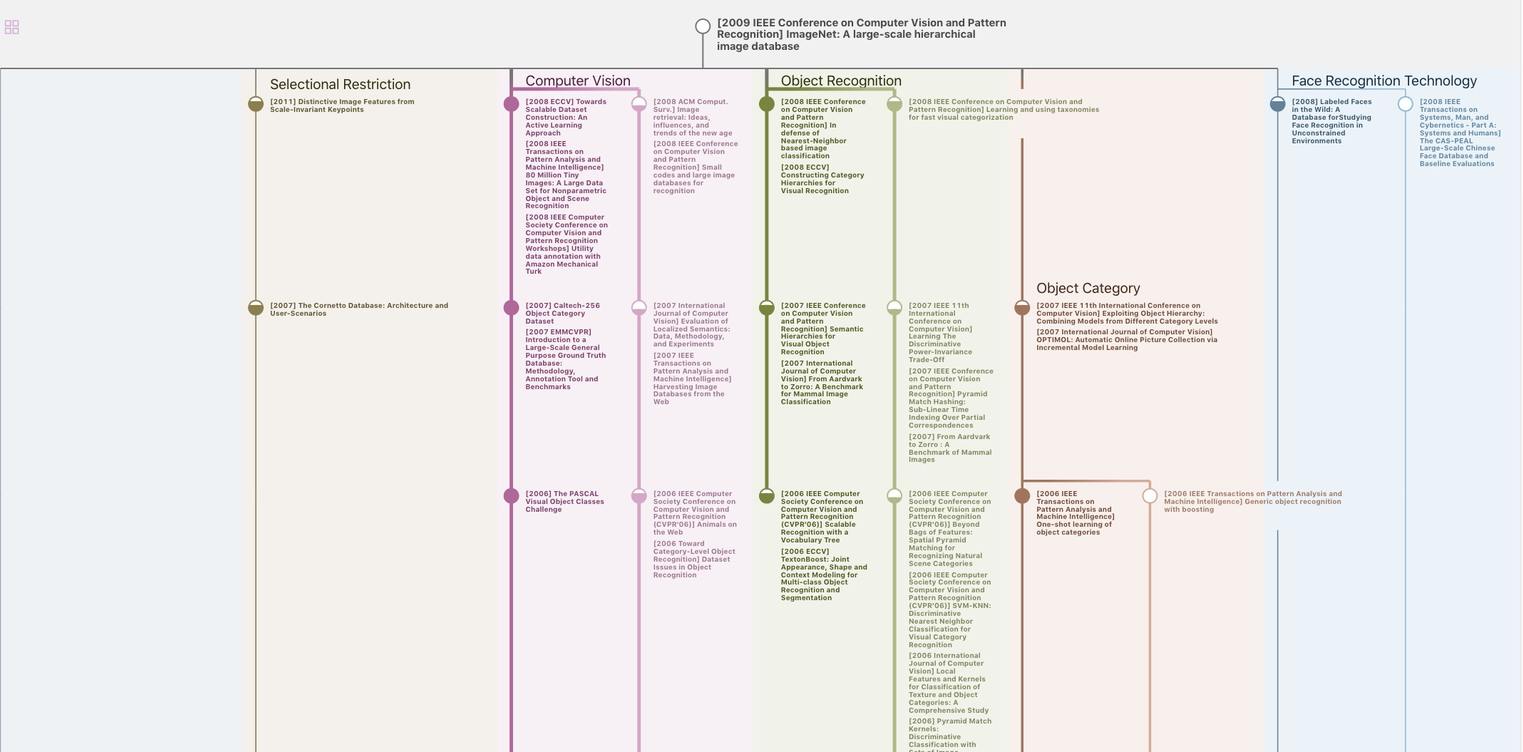
生成溯源树,研究论文发展脉络
Chat Paper
正在生成论文摘要