Single-pixel Imaging Classification Method Based on Improved Lenet-5 Network
2023 IEEE 4th International Conference on Pattern Recognition and Machine Learning (PRML)(2023)
摘要
Single-pixel imaging, as a new imaging technique, requires a large amount of data processing when reconstructing images. If the classification is based on the reconstructed images of single-pixel imaging, a large amount of data redundancy will be generated. In view of this defect, the “image-free classification” method can significantly reduce the data throughput in the step of image reconstruction in single-pixel imaging, and directly send the value of the single-pixel detector before image recovery into the convolutional neural network for classification. However, there is still some room for improvement in the classification accuracy and time of MNIST dataset. This paper combines Lenet-5 network with “image-free classification”, innovatively adjusts Lenet-5 network to a structure suitable for “image-free classification”, completes the classification of MNIST and Fashion-MNIST dataset using “image-free classification”, and adds residual structure to improve accuracy. Using the structural illumination trained by the improved neural network to project the target can increase the test accuracy from 89% to 99%, reduce the number of projection times of digital micromirror devices from 15 to 6, and reduce the total time of testing and training from 2 hours to 4.7 minutes, which indicates that the improved convolutional neural network reduces the classification time and improves the classification accuracy.
更多查看译文
关键词
single-pixel imaging,classification algorithm,image-free classification,residual structure,Lenet-5 Network
AI 理解论文
溯源树
样例
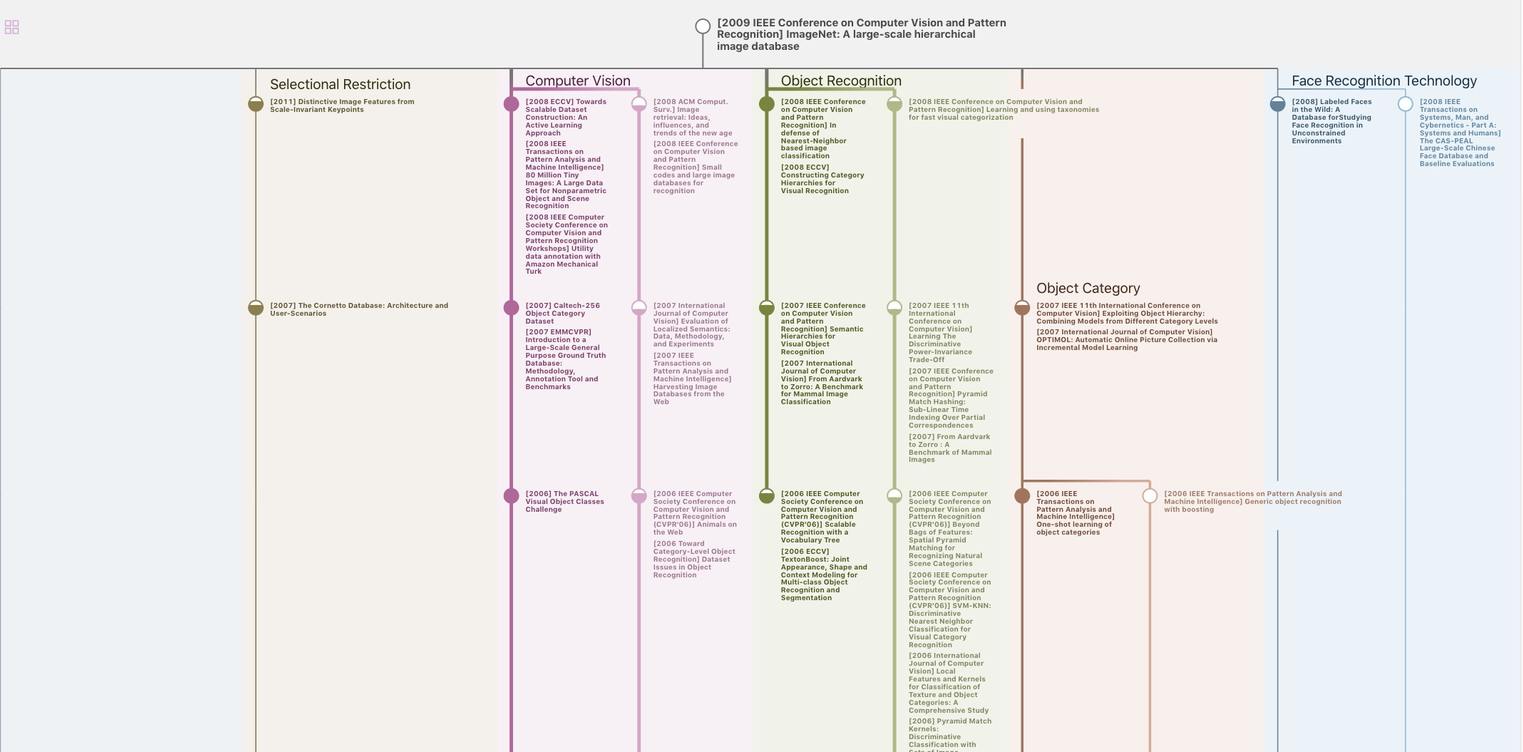
生成溯源树,研究论文发展脉络
Chat Paper
正在生成论文摘要