Improving Biomedical Claim Detection using Prompt Learning Approaches
2023 IEEE 4th International Conference on Pattern Recognition and Machine Learning (PRML)(2023)
摘要
Biomedical claim detection is an effective method to uncover negative effects arising from the treatment of disease and detect misinformation about medications from online platforms. Due to the power of pre-trained language models (PLMs), such as BERT, RoBERTa and T5, fine-tuned PLMs perform exceptionally well in biomedical claim detection. However, a gap exists in the text classification task between objective forms used in pre-training and fine-tuning for PLMs methods, preventing these models from taking full advantage of the information for biomedical claim detection. Motivated by the prompt learning approach, we propose a method, in which the classification task is transformed into a masked language modeling task that fully utilizes the mask learning capability of PLMs for better prediction of biomedical claim detection. In our method, a template with a mask representing the label is first constructed, and the mask is then filled and mapped to the corresponding label. We use three PLMs as backbone models, i.e., BERT, RoBERTa, and T5, with both hard and mixed templates which are fully and partially predefined templates. Experimental results using the BioClaim dataset demonstrate the superiority of the prompt learning methods over the BERT and RoBERTa classification baselines. Furthermore, the T5 model with mixed template consistently outperforms the rest of experimented models and achieves state-of-the-art performance with an increase of 5.3% on F1-score compared to previous research on this dataset.
更多查看译文
关键词
Claim detection,Pre-trained language models,Prompt learning,Natural language processing
AI 理解论文
溯源树
样例
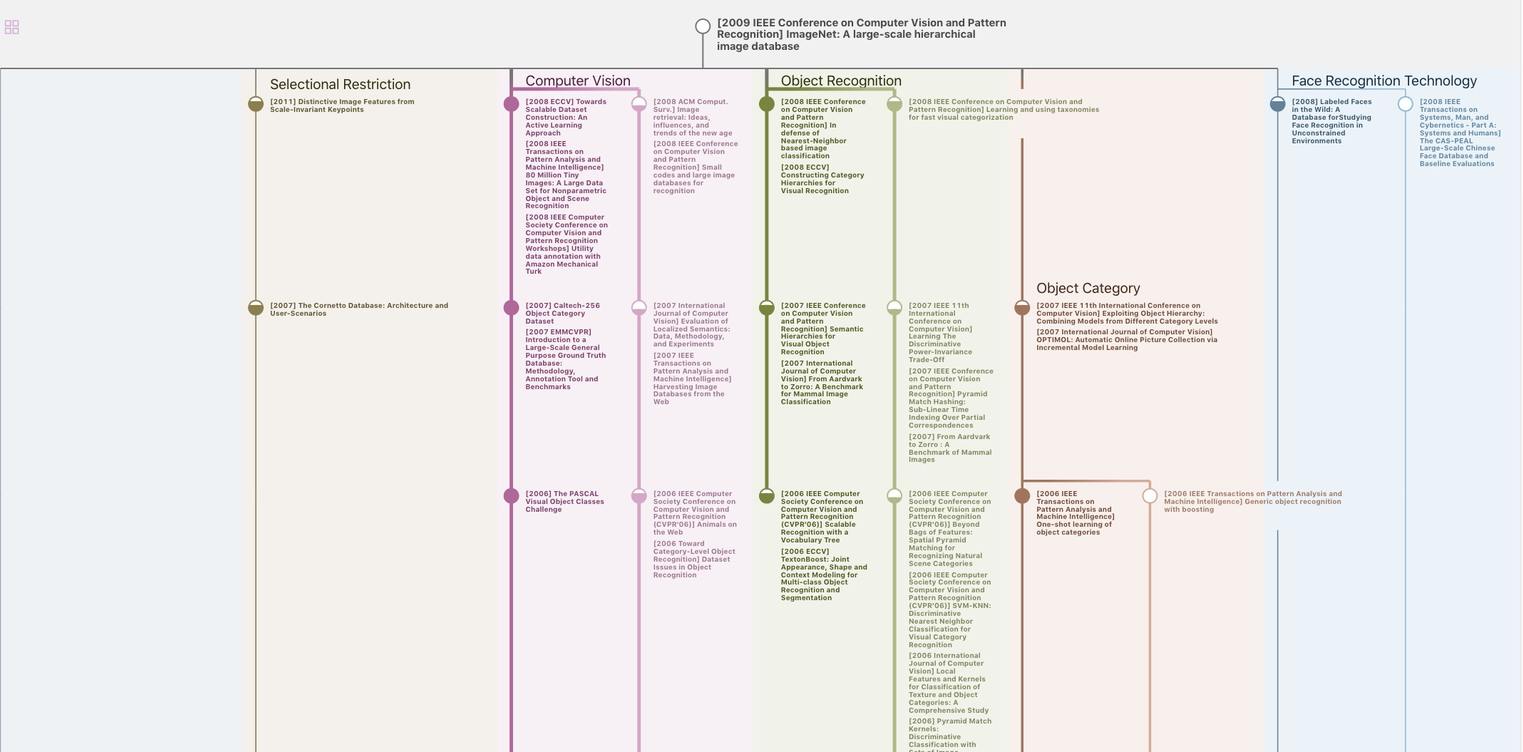
生成溯源树,研究论文发展脉络
Chat Paper
正在生成论文摘要