Hybrid machine learning models for aboveground biomass estimations
ECOLOGICAL INFORMATICS(2024)
摘要
Forest biomass provides a quantitative assessment for carbon stock marketing on a national or regional scale. Some countries have committed to net zero carbon emissions, so proper biomass estimations are essential. This study investigates the uses of machine learning (LightGBM, XGBoost), in which hyperparameters were tuned by Bayesian-based Optimisers and a novel Tasmanian Devil Optimisation algorithm for estimates of aboveground biomass (AGB) using Sentinel 1A, Landsat images, and ground survey data. A province in the northern part of Vietnam was selected as a case study since the change in land cover has been considered crucial. The models were optimized/trained and validated using statistical indicators, namely, root mean square error (RMSE), coefficient of determination (R2), and mean absolute error (MAE). The trained models were further explained using SHAP values to understand better how they perform and the contribution of each feature to the overall estimates. The results showed that the three indicators of the proposed model were statistically better than those of the reference methods. Specifically, the hybrid model ended up at RMSE -13.87, MAE - 10.62, and R2 - 0.79 for the estimation of AGB. Based on the experience, such hybrid integration can be recommended as an alternative solution for biomass estimation. In a broader context, the fast growth of machine learning and optimization algorithms has created new scientifically sound solutions for a better analysis of forest cover.
更多查看译文
关键词
Aboveground biomass,Gradient boosting,Bayesian optimisations,Meta-heuristic optimisation
AI 理解论文
溯源树
样例
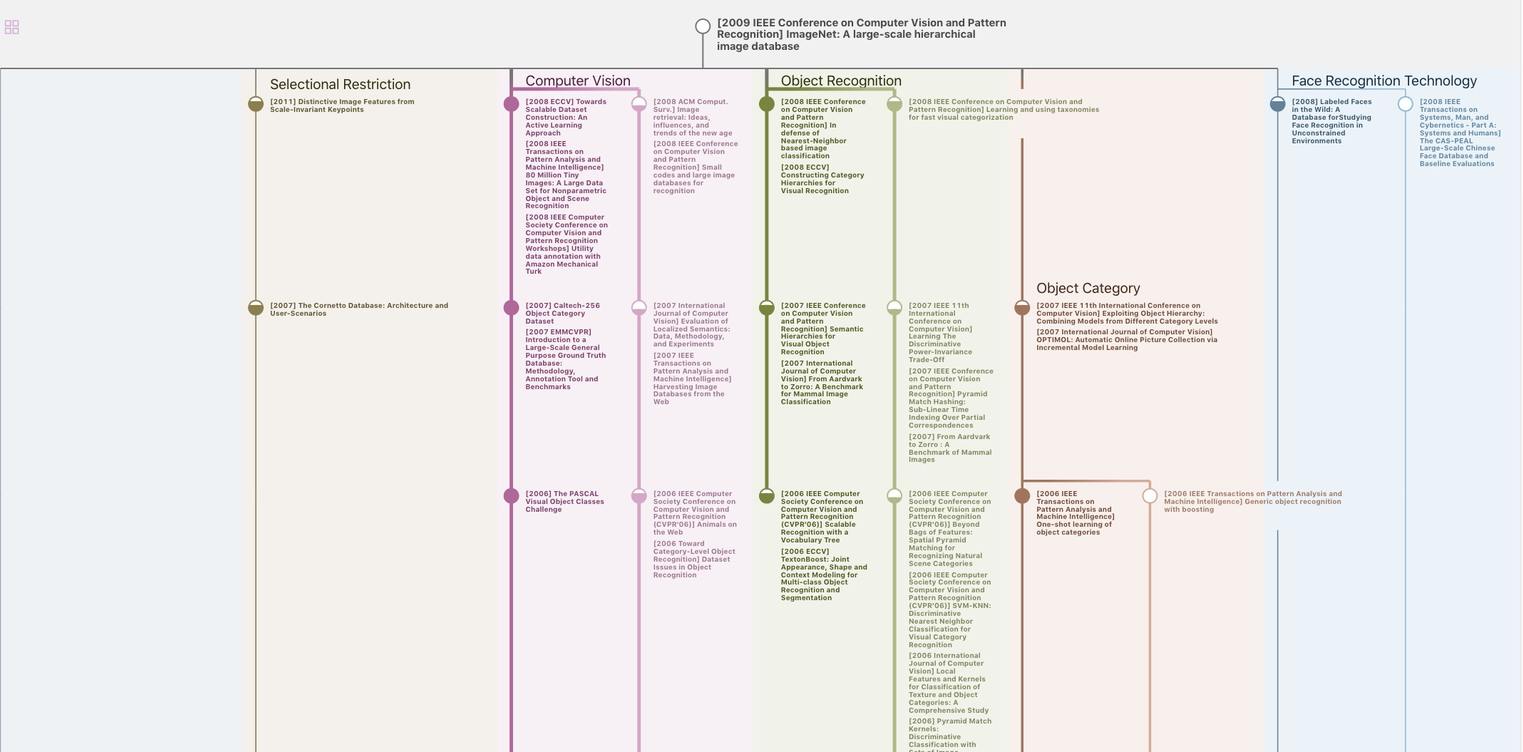
生成溯源树,研究论文发展脉络
Chat Paper
正在生成论文摘要