Privacy-Preserving Video Understanding via Transformer-based Federated Learning.
2023 IEEE Conference on Dependable and Secure Computing (DSC)(2023)
摘要
Video understanding has been an active area of research over the past several years, which is dominated by deep neural networks like image understanding and other computer vision tasks. In real-world implementations, even after pre-training on large datasets, state-of-the-art deep neural networks for video understanding tasks, such as anomaly detection and action recognition, can greatly benefit from diverse training data from multiple sources to adapt to the specific application. However, sharing videos collected by multiple sources with a central unit may not be feasible in practice due to privacy and communication constraints. Federated Learning (FL),which allows data parties to collaborate on machine learning models while preserving data privacy and reducing communication requirements, can be used to overcome these challenges. Despite significant progress on various computer vision tasks, FL for video understanding tasks has been largely unexplored. To this end, we propose a novel transformer-based approach for video anomaly detection and action recognition, and extensively benchmark the model performance in FL setting. Our results indicate that the proposed approach outperforms all existing state-of-the-art approaches under the centralized (non-FL) setting and performs competitively under the FL setting.
更多查看译文
关键词
privacy-preserving machine learning,federated learning,video anomaly detection,action recognition,video transformer
AI 理解论文
溯源树
样例
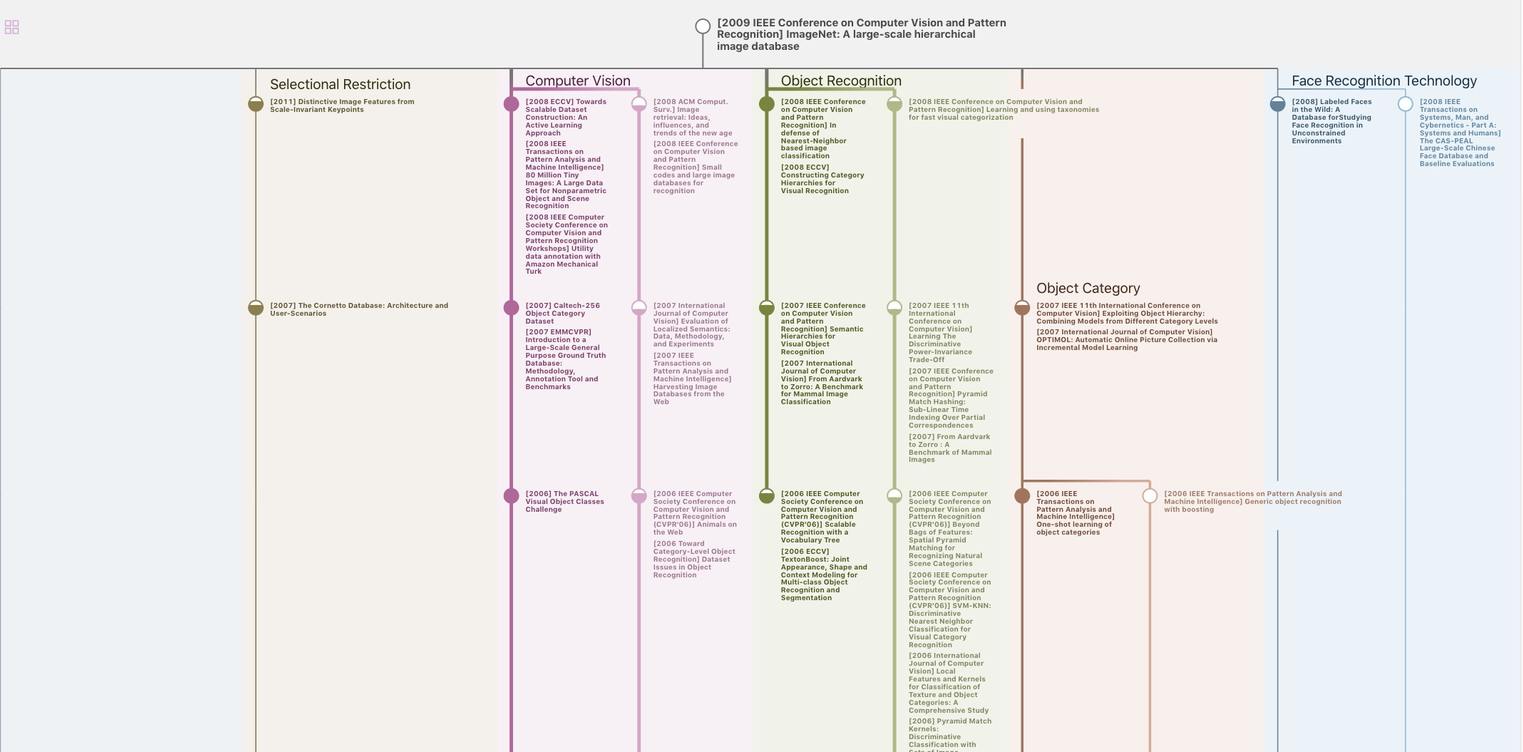
生成溯源树,研究论文发展脉络
Chat Paper
正在生成论文摘要