A bi-objective low-carbon economic scheduling method for cogeneration system considering carbon capture and demand response
EXPERT SYSTEMS WITH APPLICATIONS(2024)
摘要
Carbon capture and storage (CCS), energy storage (ES), and demand response (DR) mechanisms are introduced into a cogeneration system to enhance their ability to absorb wind energy, reduce carbon emissions, and improve operational efficiency. First, a bi-objective low-carbon economic scheduling model of a cogeneration system considering CCS, ES, and DR was developed. In this model, the ES and CCS remove the coupling between power generation and heating. The DR mechanism, which is based on the time-of-use electricity price and heating comfort, further enhanced the flexibility of the system. In addition, an improved bare-bones multi-objective particle swarm optimisation (IBBMOPSO) was designed to directly obtain the Pareto front of the low-carbon economy scheduling model. The particle position update mode was improved to balance global and local search capabilities in various search stages. The Taguchi method was used to calibrate the algorithm parameters. The inverse generational distance (IGD), hypervolume (HV), and maximum spread (MS) were used to evaluate the distribution and convergence performance of the algorithm. The improved technique for order preference by similarity to an ideal solution (TOPSIS) method was utilised to obtain the optimal compromise solution. Finally, the proposed method was tested on a cogeneration system in Northeast China. According to the comparison results, the average economic cost of the cogeneration system considering CCS, ES, and DR was reduced by approximately 1.13%, and carbon emissions were reduced by 6.79%. The IBBMOPSO is more competitive than the NSGA-II, MOWDO, MOMA, MOPSO, and BBMOPSO in low-carbon economic scheduling for the cogeneration system.
更多查看译文
关键词
Cogeneration system,Carbon capture,Demand response,Improved bare-bones multi-objective particle,swarm optimisation,Low-carbon economic scheduling
AI 理解论文
溯源树
样例
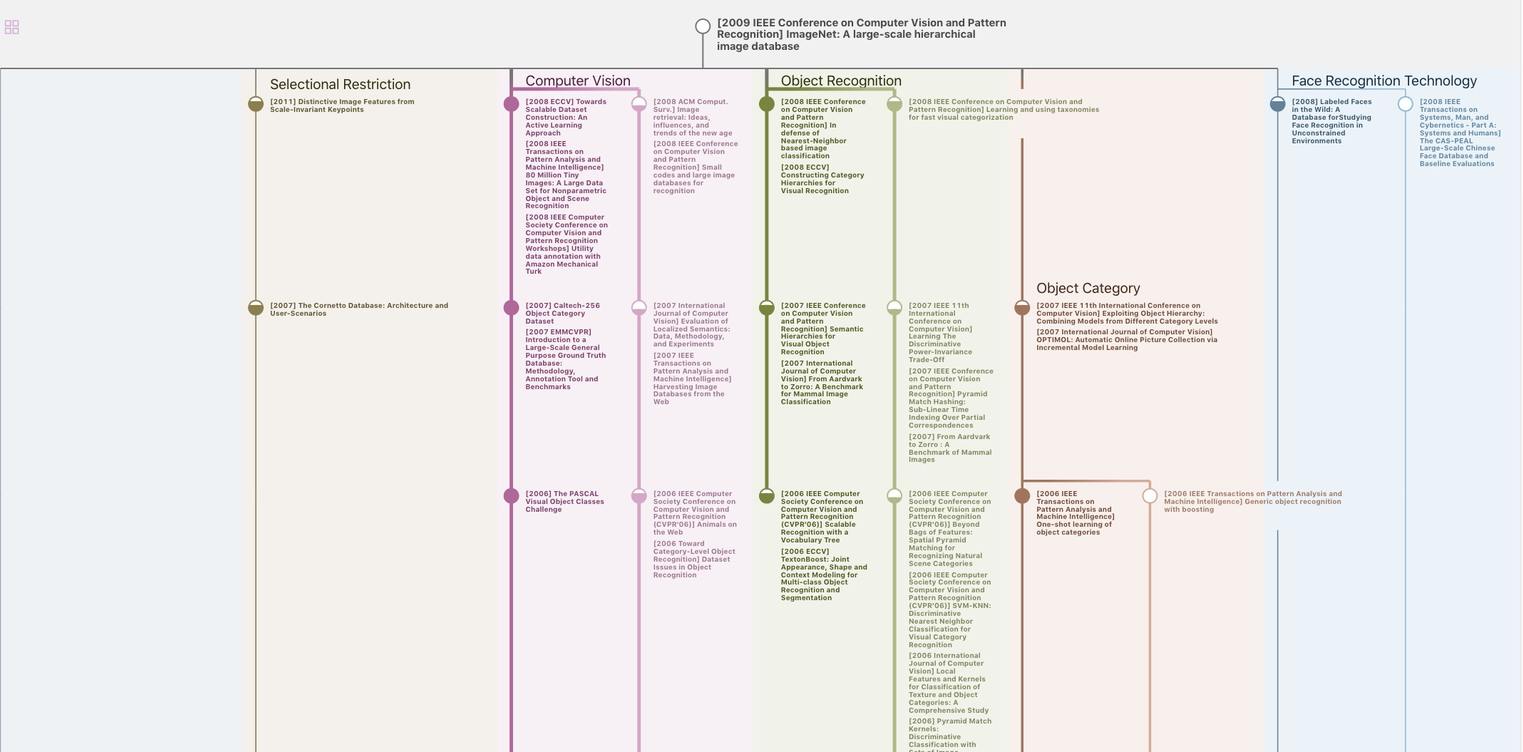
生成溯源树,研究论文发展脉络
Chat Paper
正在生成论文摘要