Enhancing Trajectory Prediction through Self-Supervised Waypoint Noise Prediction
CoRR(2023)
摘要
Trajectory prediction is an important task that involves modeling the
indeterminate nature of traffic actors to forecast future trajectories given
the observed trajectory sequences. However, current methods confine themselves
to presumed data manifolds, assuming that trajectories strictly adhere to these
manifolds, resulting in overly simplified predictions. To this end, we propose
a novel approach called SSWNP (Self-Supervised Waypoint Noise Prediction). In
our approach, we first create clean and noise-augmented views of past observed
trajectories across the spatial domain of waypoints. We then compel the
trajectory prediction model to maintain spatial consistency between predictions
from these two views, in addition to the trajectory prediction task.
Introducing the noise-augmented view mitigates the model's reliance on a narrow
interpretation of the data manifold, enabling it to learn more plausible and
diverse representations. We also predict the noise present in the two views of
past observed trajectories as an auxiliary self-supervised task, enhancing the
model's understanding of the underlying representation and future predictions.
Empirical evidence demonstrates that the incorporation of SSWNP into the model
learning process significantly improves performance, even in noisy
environments, when compared to baseline methods. Our approach can complement
existing trajectory prediction methods. To showcase the effectiveness of our
approach, we conducted extensive experiments on three datasets: NBA Sports VU,
ETH-UCY, and TrajNet++, with experimental results highlighting the substantial
improvement achieved in trajectory prediction tasks.
更多查看译文
AI 理解论文
溯源树
样例
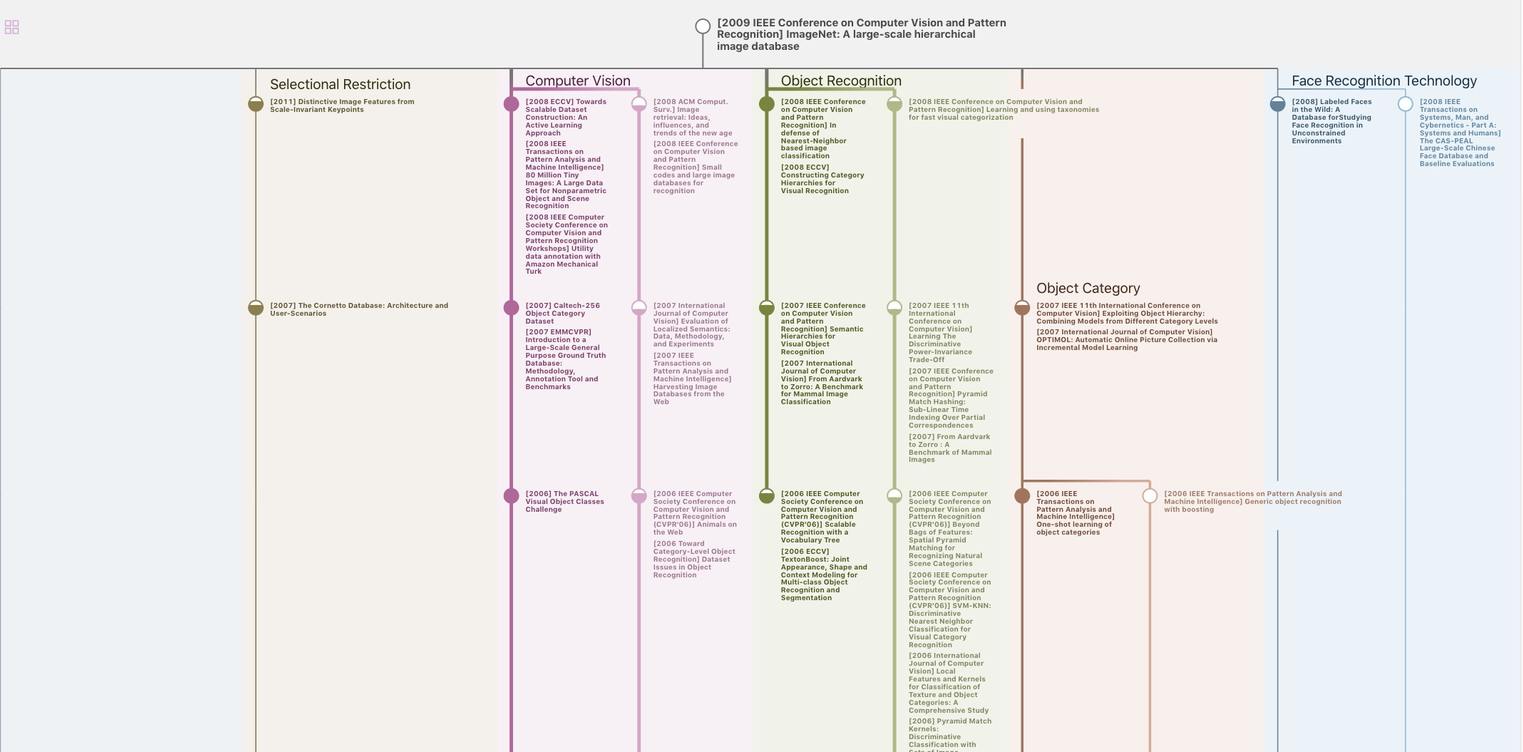
生成溯源树,研究论文发展脉络
Chat Paper
正在生成论文摘要