Benchmarking the Full-Order Model Optimization Based Imitation in the Humanoid Robot Reinforcement Learning Walk
2023 21ST INTERNATIONAL CONFERENCE ON ADVANCED ROBOTICS, ICAR(2023)
摘要
When a gait of a bipedal robot is developed using deep reinforcement learning, reference trajectories may or may not be used. Each approach has its advantages and disadvantages, and the choice of method is up to the control developer. This paper investigates the effect of reference trajectories on locomotion learning and the resulting gaits. We implemented three gaits of a full-order anthropomorphic robot model with different reward imitation ratios, provided sim-to-sim control policy transfer, and compared the gaits in terms of robustness and energy efficiency. In addition, we conducted a qualitative analysis of the gaits by interviewing people, since our task was to create an appealing and natural gait for a humanoid robot. According to the results of the experiments, the most successful approach was the one in which the average value of rewards for imitation and adherence to command velocity per episode remained balanced throughout the training. The gait obtained with this method retains naturalness (median of 3.6 according to the user study) compared to the gait trained with imitation only (median of 4.0), while remaining robust close to the gait trained without reference trajectories.
更多查看译文
关键词
Walking,Imitation,Humanoid Robot,Full-order Model,Energy Efficiency,Deep Reinforcement Learning,Reference Trajectory,Robot Model,Velocity Commands,Degrees Of Freedom,Learning Process,Center Of Mass,Actuator,Flat Surface,Current Position,Transportation Costs,Angular Velocity,Joint Position,Proportional-integral-derivative,Reward Function,Linear Velocity,Motor Position,Motor Torque,Input Clock,Number Of Motors,Deep Reinforcement Learning Approach,Bipedal Locomotion,Target Velocity,Reduced-order Model,Asteraceae
AI 理解论文
溯源树
样例
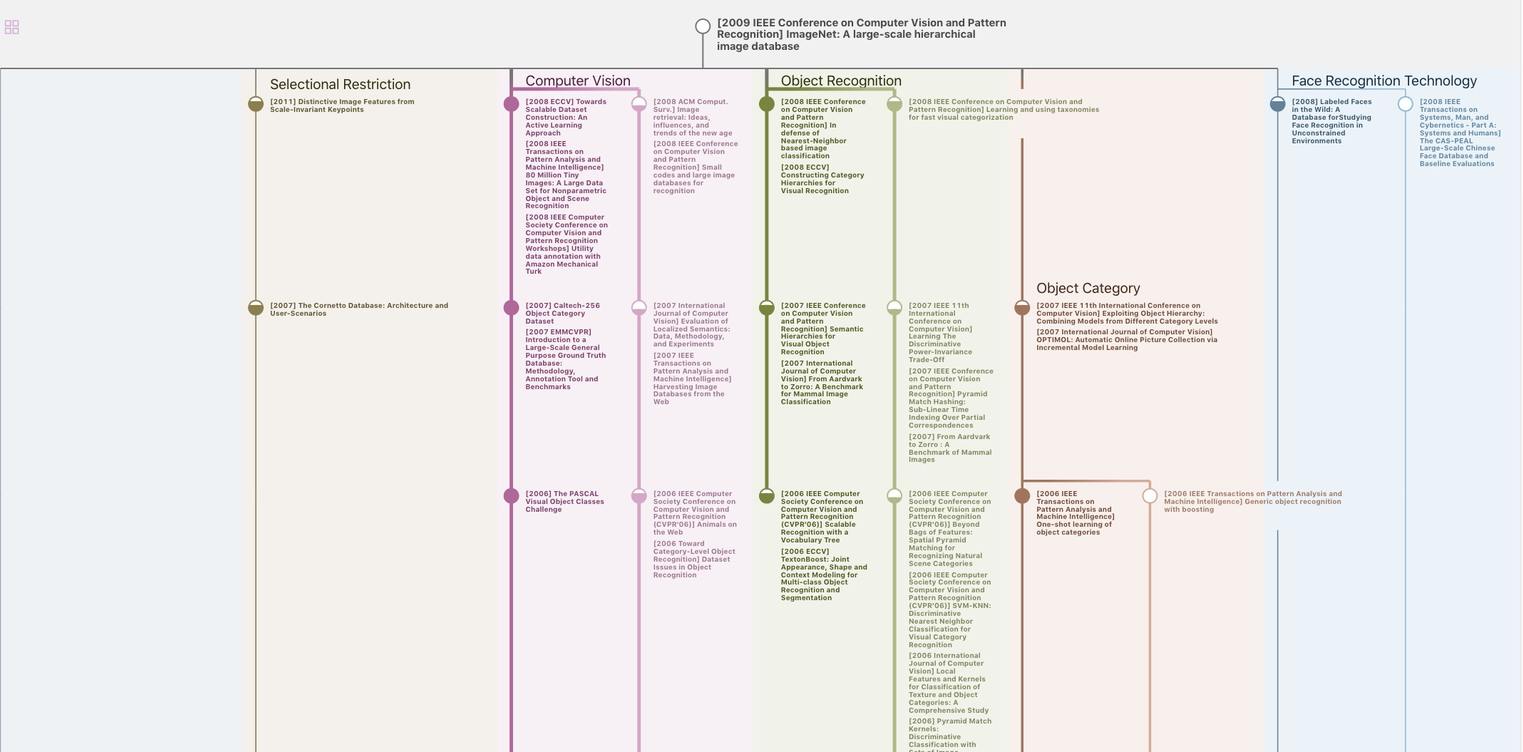
生成溯源树,研究论文发展脉络
Chat Paper
正在生成论文摘要