LogoStyleFool: Vitiating Video Recognition Systems Via Logo Style Transfer
arXiv (Cornell University)(2023)
摘要
Video recognition systems are vulnerable to adversarial examples. Recentstudies show that style transfer-based and patch-based unrestrictedperturbations can effectively improve attack efficiency. These attacks,however, face two main challenges: 1) Adding large stylized perturbations toall pixels reduces the naturalness of the video and such perturbations can beeasily detected. 2) Patch-based video attacks are not extensible to targetedattacks due to the limited search space of reinforcement learning that has beenwidely used in video attacks recently. In this paper, we focus on the videoblack-box setting and propose a novel attack framework named LogoStyleFool byadding a stylized logo to the clean video. We separate the attack into threestages: style reference selection, reinforcement-learning-based logo styletransfer, and perturbation optimization. We solve the first challenge byscaling down the perturbation range to a regional logo, while the secondchallenge is addressed by complementing an optimization stage afterreinforcement learning. Experimental results substantiate the overallsuperiority of LogoStyleFool over three state-of-the-art patch-based attacks interms of attack performance and semantic preservation. Meanwhile, LogoStyleFoolstill maintains its performance against two existing patch-based defensemethods. We believe that our research is beneficial in increasing the attentionof the security community to such subregional style transfer attacks.
更多查看译文
关键词
Video Analysis,Detection,Security Analysis
AI 理解论文
溯源树
样例
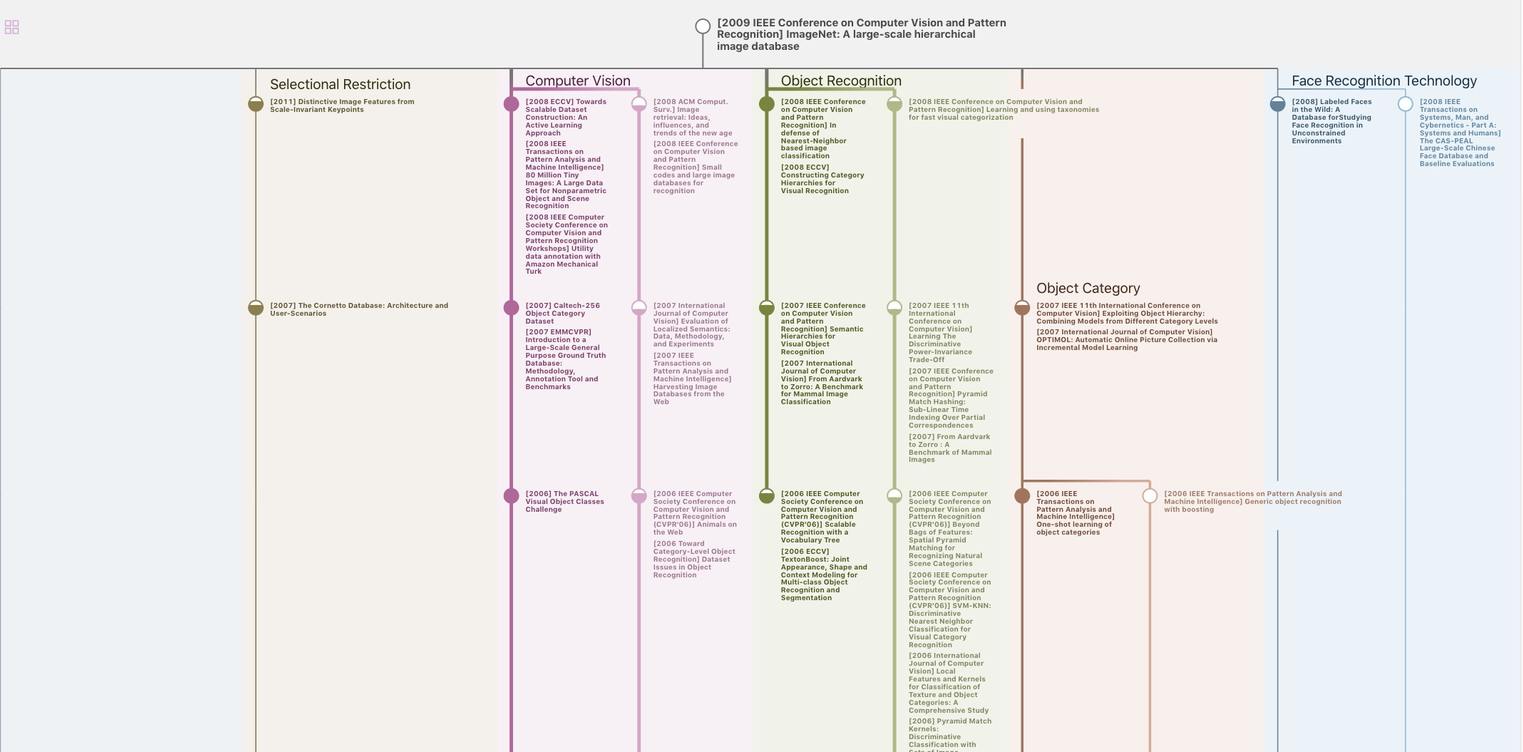
生成溯源树,研究论文发展脉络
Chat Paper
正在生成论文摘要