Towards Neuromorphic Compression based Neural Sensing for Next-Generation Wireless Implantable Brain Machine Interface
arxiv(2023)
摘要
This work introduces a neuromorphic compression based neural sensing
architecture with address-event representation inspired readout protocol for
massively parallel, next-gen wireless iBMI. The architectural trade-offs and
implications of the proposed method are quantitatively analyzed in terms of
compression ratio and spike information preservation. For the latter, we use
metrics such as root-mean-square error and correlation coefficient between the
original and recovered signal to assess the effect of neuromorphic compression
on spike shape. Furthermore, we use accuracy, sensitivity, and false detection
rate to understand the effect of compression on downstream iBMI tasks,
specifically, spike detection. We demonstrate that a data compression ratio of
$50-100$ can be achieved, $5-18\times$ more than prior work, by selective
transmission of event pulses corresponding to neural spikes. A correlation
coefficient of $\approx0.9$ and spike detection accuracy of over $90\%$ for the
worst-case analysis involving $10K$-channel simulated recording and typical
analysis using $100$ or $384$-channel real neural recordings. We also analyze
the collision handling capability and scalability of the proposed pipeline.
更多查看译文
AI 理解论文
溯源树
样例
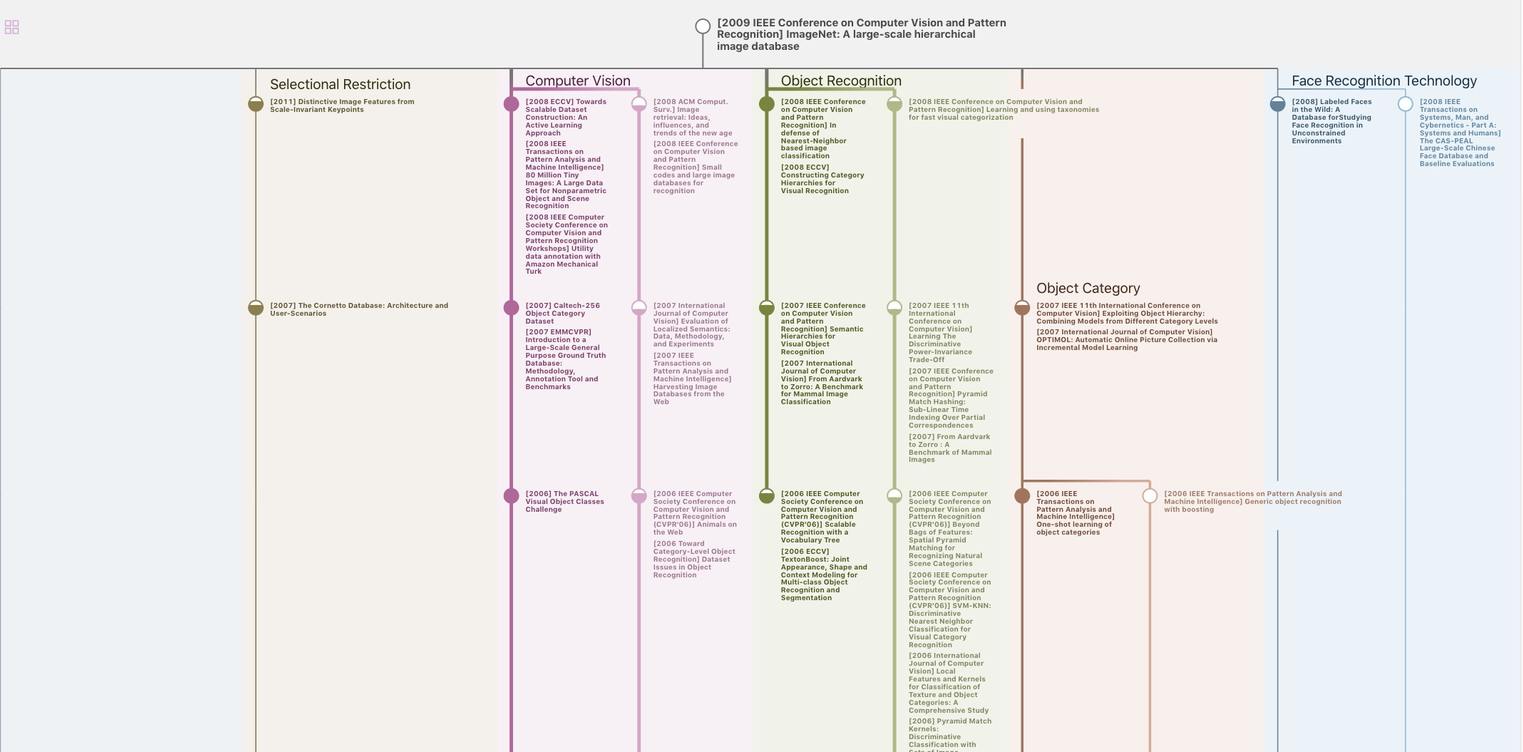
生成溯源树,研究论文发展脉络
Chat Paper
正在生成论文摘要