Early Diagnosis and Biomarkers of Alzheimer's Disease Based on Spatio-temporal Graph Convolution Network.
2023 45th Annual International Conference of the IEEE Engineering in Medicine & Biology Society (EMBC)(2023)
摘要
Functional magnetic resonance imaging (fMRI) could detect the dynamic activity of brain function and communication. Previous studies have found reduced brain functional connectivity in Alzheimer's disease (AD) patients. In this study, we proposed to process fMRI data by spatio-temporal graph convolution network (ST-GCN) to achieve an early differential diagnosis of AD and to extract image markers using gradient-weighted class activation mapping (Grad-CAM). The data used in this study were from the Alzheimer's Disease Neuroimaging Initiative (ADNI) database, Xuanwu Hospital, and Tongji Hospital. The study included 1105 normal controls and 790 patients with mild cognitive impairment (MCI). The grid search method of K-fold cross-validation was used to train the model. In addition, we used Grad-CAM to extract image markers and carried out visualization analysis. This model obtains better AD diagnosis power: accuracy = 0.92, sensitivity = 0.97, specificity = 0.89, and area under the curve=0.96. Salient brain regions extracted by Grad-CAM include the paracentral lobule, inferior occipital gyrus, middle frontal gyrus, superior temporal gyrus, cuneus, posterior cingulate gyrus, and superior parietal gyrus. Our proposed ST-GAN model will help to explore objective markers that can be used for the early diagnosis of AD.Clinical relevance- Our proposed model shows great potential for enhancing the understanding of the pathology of AD by detecting functional connectivity interruptions.
更多查看译文
AI 理解论文
溯源树
样例
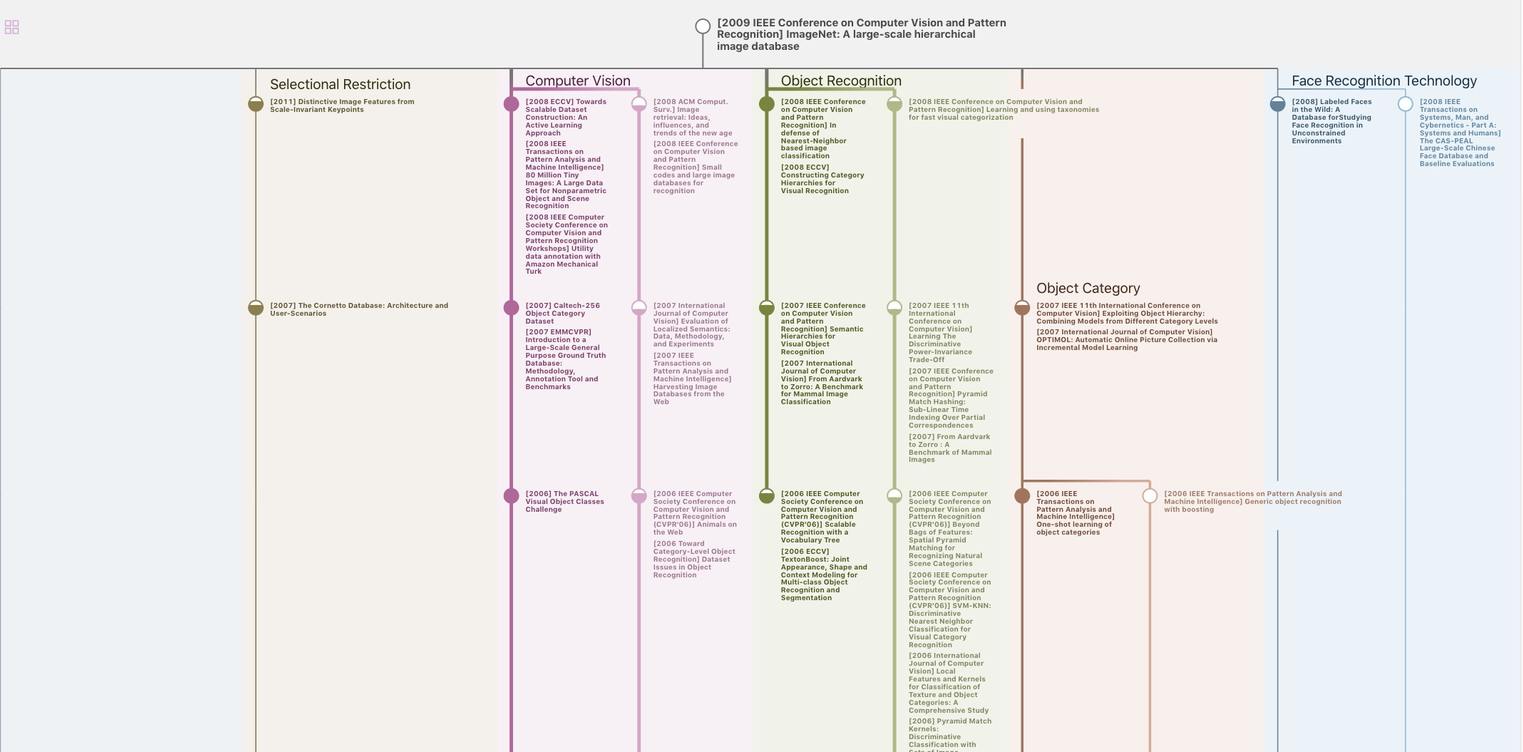
生成溯源树,研究论文发展脉络
Chat Paper
正在生成论文摘要