Representative Data Selection for Efficient Medical Incremental Learning
2023 45TH ANNUAL INTERNATIONAL CONFERENCE OF THE IEEE ENGINEERING IN MEDICINE & BIOLOGY SOCIETY, EMBC(2023)
摘要
To train a deep neural network relies on a large amount of annotated data. In special scenarios like industry defect detection and medical imaging, it is hard to collect sufficient labeled data all at once. Newly annotated data may arrive incrementally. In practice, we also prefer our target model to improve its capability gradually as new data comes in by quick re-training. This work tackles this problem from a data selection prospective by constraining ourselves to always retrain the target model with a fix amount of data after new data comes in. A variational autoencoder (VAE) and an adversarial network are combined for data selection, achieving fast model retraining. This enables the target model to continually learn from a small training set while not losing the information learned from previous iterations, thus incrementally adapting itself to new-coming data. We validate our framework on the LGG Segmentation dataset for the semantic segmentation task.
更多查看译文
关键词
Adversarial network,continual learning,data management,incremental learning,variational autoencoder
AI 理解论文
溯源树
样例
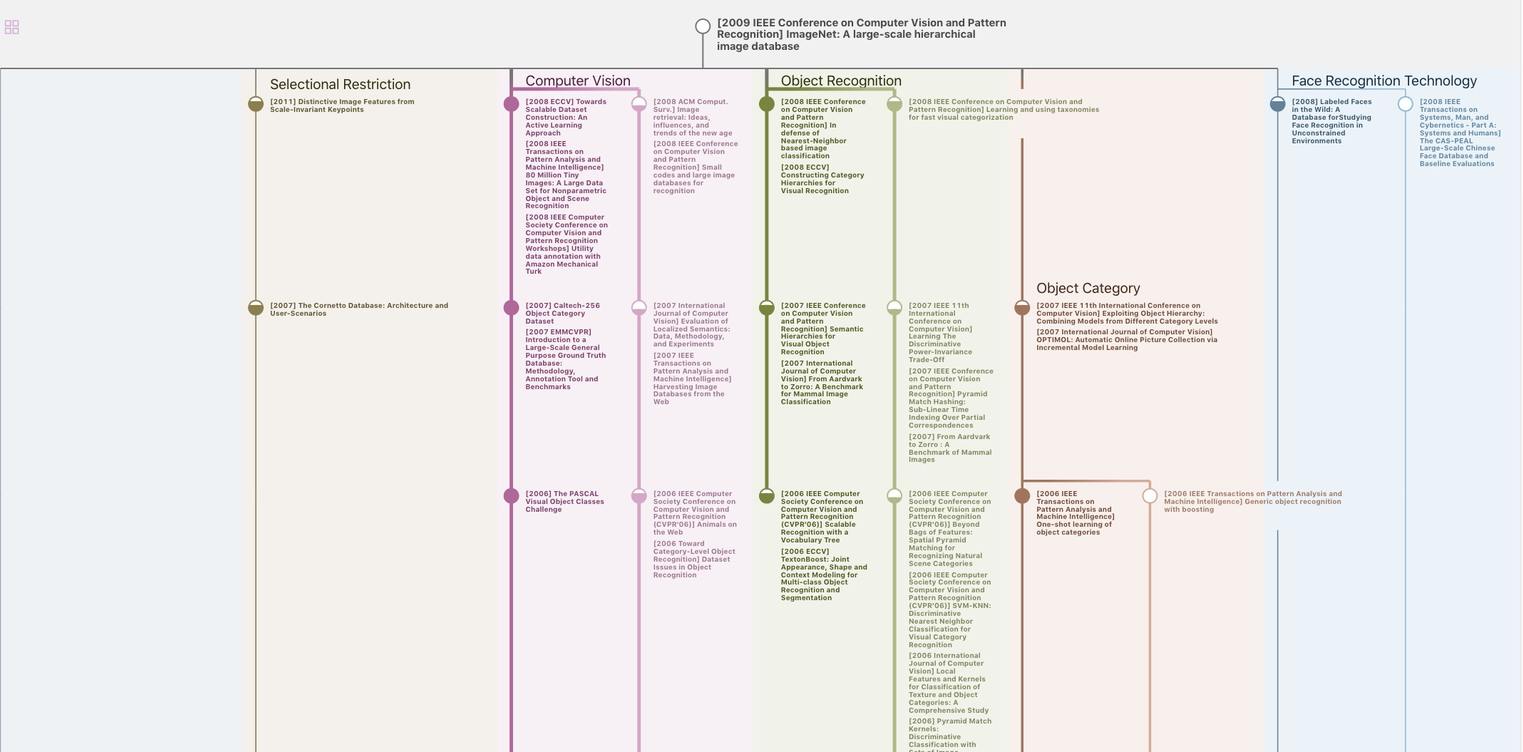
生成溯源树,研究论文发展脉络
Chat Paper
正在生成论文摘要