An Autonomous Multi Agent Q-Learning Approach for Resource Allocation in D2D-Enabled Heterogeneous Networks
2023 31st International Conference on Electrical Engineering (ICEE)(2023)
摘要
In today's heterogeneous networks (HetNets), Device to Device (D2D) communications is a vital technology that enables the user equipment to communicate on a point-to-point basis without needing for network infrastructure. This paper considers a three-tier D2D-enabled HetNets with one macro cell, several D2D users, and several femto cells with femto cellular users scattered over each cell. Then, a new method named Autonomous Multi Agent Q-learning (AMAQL) is presented for optimal transmission power level selection and sub-channel allocation. Each user's equipment (UE) is assumed to be provided with an intelligent agent. This agent can make decisions about resource management independently, without any requirements for network infrastructure. The simulation results show that the proposed AMAQL method convergences properly and achieves better system performance than other existing strategies.
更多查看译文
关键词
Heterogeneous Network,Device to Device (D2D) Communications,Resource Allocation,Q-learning
AI 理解论文
溯源树
样例
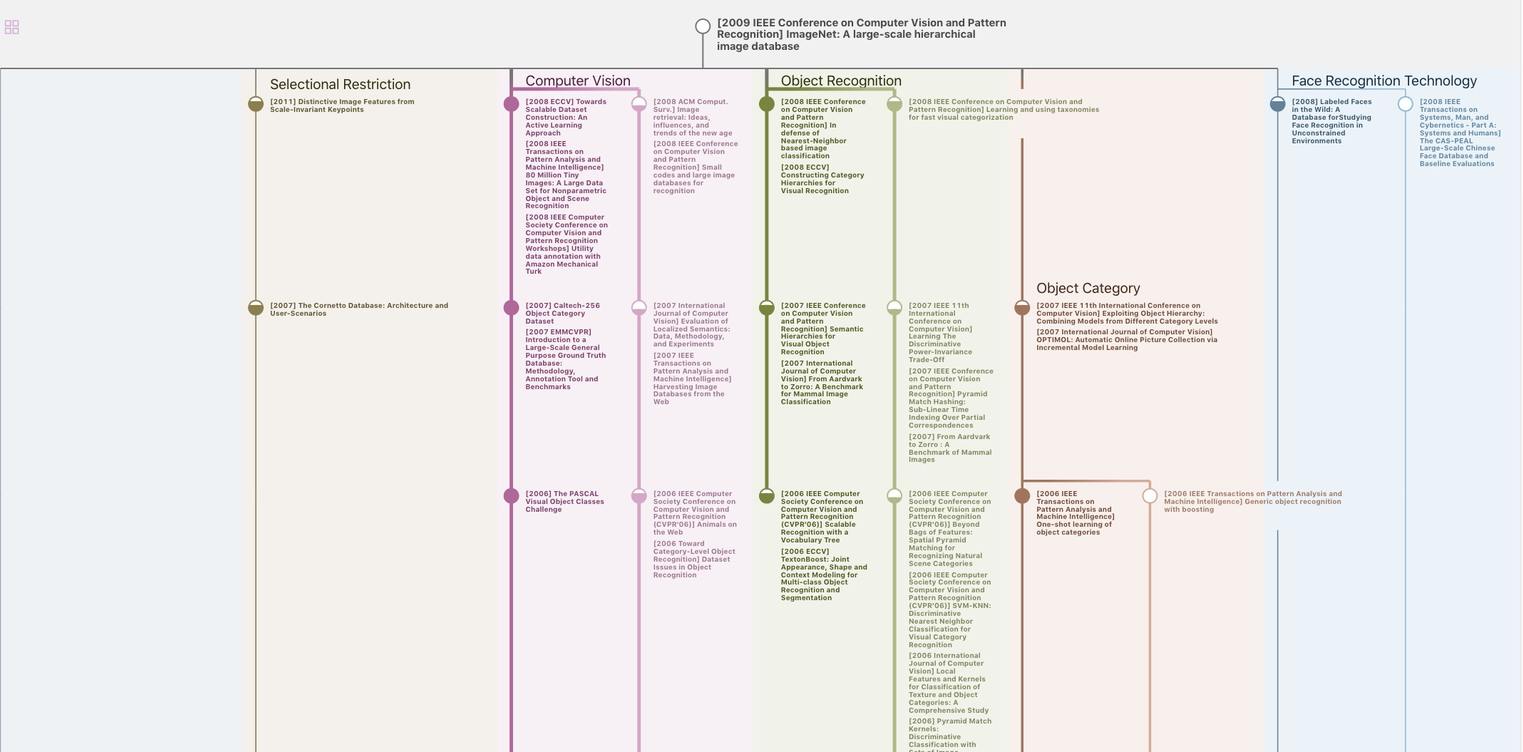
生成溯源树,研究论文发展脉络
Chat Paper
正在生成论文摘要