Self-aware Collaborative Edge Inference with Embedded Devices for Task-oriented IIoT
2023 IEEE 98TH VEHICULAR TECHNOLOGY CONFERENCE, VTC2023-FALL(2023)
摘要
The computing and communication resources of embedded devices are constrained and heterogeneous, resulting in a low quality-of-experience for compute -intensive applications in task -oriented industrial Internet of Things (IIoT), such as edge inference. To address these challenges, we first propose a model partitioning-based self-aware collaborative edge inference framework. Furthermore, the throughput -aware collaborative inference algorithm is designed for typical IIoT scenario, stacking tasks. Via jointly optimizing the partition layer and collaborative device selection, the optimal inference efficiency, maximum inference throughput, can be obtained. Finally, the performance of our proposal is demonstrated by extensive simulations and tests based on 10 Raspberry Pi 4Bs and popular models. Specifically, with the proposed algorithm, our platform reaches up to 14.77x throughput speed up for stacking tasks, which indicates that the the proposed design can improve the inference efficiency.
更多查看译文
AI 理解论文
溯源树
样例
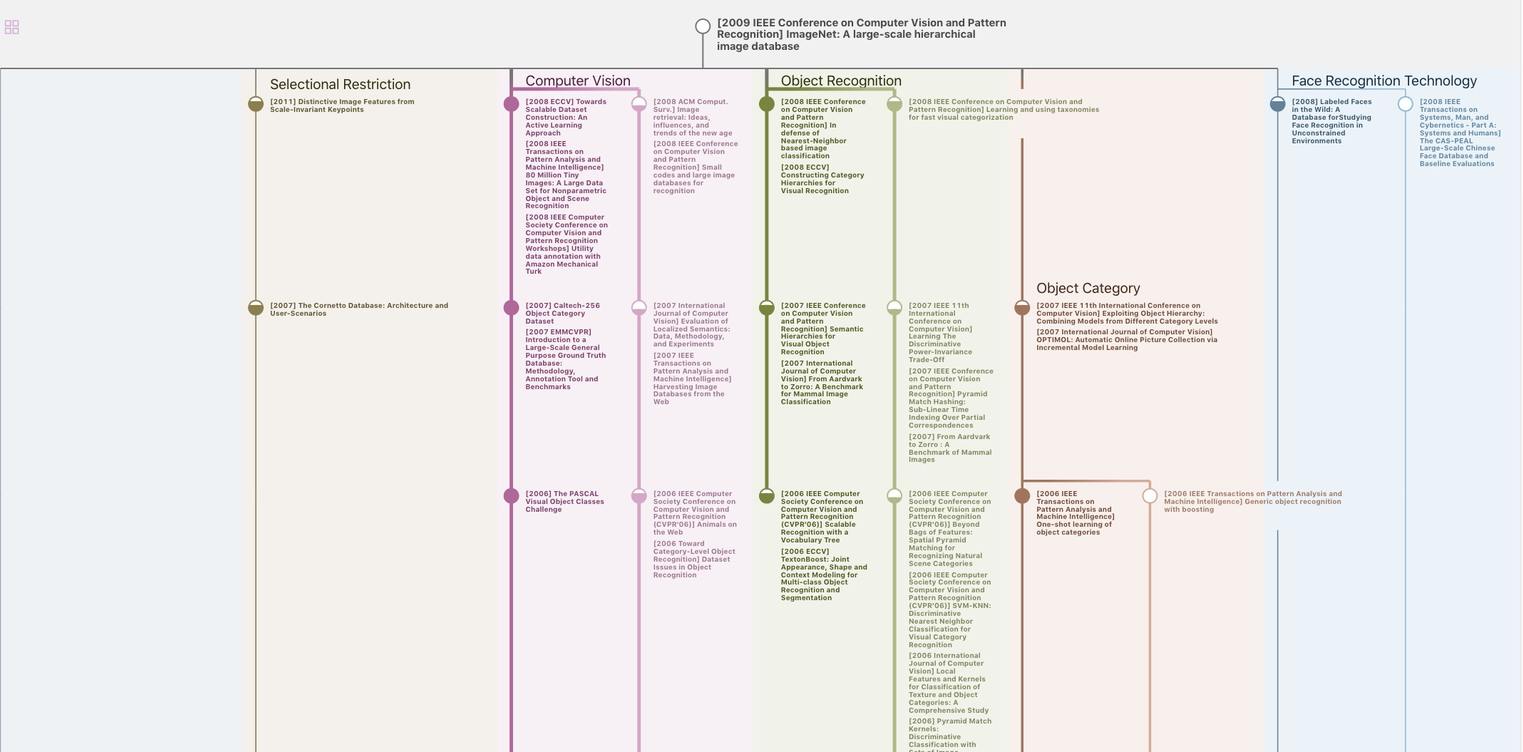
生成溯源树,研究论文发展脉络
Chat Paper
正在生成论文摘要