Feature Learning Networks for Floor Sensor-based Gait Recognition
2023 45TH ANNUAL INTERNATIONAL CONFERENCE OF THE IEEE ENGINEERING IN MEDICINE & BIOLOGY SOCIETY, EMBC(2023)
摘要
Deep learning (DL) has become a powerful tool in many image classification applications but often requires large training sets to achieve high accuracy. For applications where the available data are limited, this can become a severely limiting factor in model performance. To address this limitation, feature learning network approaches that integrate traditional feature extraction methods with DL frameworks have been proposed. In this study, the performances of traditional methods: discrete wavelet transform (DWT), discrete cosine transform (DCT), independent component analysis (ICA), and principal component analysis (PCA); and their corresponding feature networks based on a convolutional neural network (CNN) framework: ScatNet (wavelet scattering network), DCTNet, ICANet, and PCANet, were investigated for use in pressure-based footstep recognition when the limited sample size is available for person authentication. The results show that the feature learning networks (90.6% accuracy) achieved significantly better performance on average than the conventional feature extraction methods (79.7% accuracy) (p < 0.05). Among the different feature networks, PCANet provided the best verification performance, with an accuracy of 92.2%. Feature learning networks are simple and effective approaches that can be a promising solution for applications like floor-based gait recognition in a security access scenario (such as workspace environment and border control) when small amounts of data are available for training models to differentiate between a larger group of users.
更多查看译文
AI 理解论文
溯源树
样例
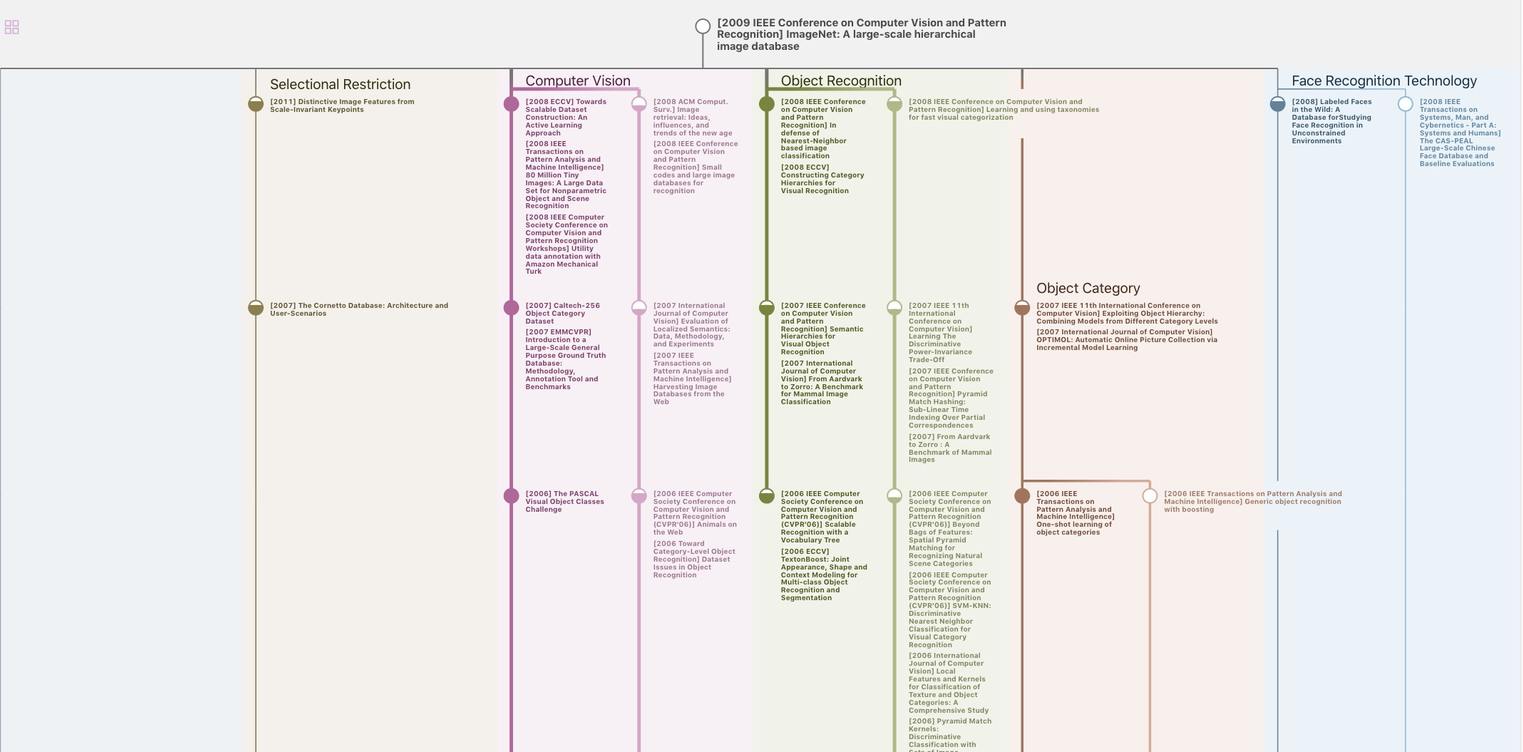
生成溯源树,研究论文发展脉络
Chat Paper
正在生成论文摘要