Exploring Learning-based Control Policy for Fish-like Robots in Altered Background Flows
2023 IEEE/RSJ INTERNATIONAL CONFERENCE ON INTELLIGENT ROBOTS AND SYSTEMS, IROS(2023)
摘要
The study of motion control for the fish-like robots in complex fluid fields is of great importance in improving the performance of underwater vehicles, due to its strong maneuverability, propulsion efficiency, and deceptive visual appearance. In this article, a novel learning-based control framework is first proposed to autonomously explore efficient control policies that are capable of performing motion control tasks in non-quiescent and unknown background flows. First, we utilize a high-fidelity simulation system, named FishGym, to generate various uniform flows. Next, a DRL-based algorithm is incorporated with the FishGym to train the fish-like robot to control its motion to optimally complete a delicately designed task (Approaching Target and Stay) in both quiescent and uniform flow. Then, the obtained control policy together with an online estimator is directly applied to a Path-Following Task. The proposed framework well balances the simulation accuracy and the computational efficiency, which is of crucial importance for effective coupling with the learning algorithm. The simulation results indicate that, via the proposed learning framework, the robot successfully acquired a swimming strategy that can be used to adapt to different background flows and tasks. Furthermore, we also observe some adaptation behavior of the robot, such as rheotaxis, that is similar to the fish in nature, which gains us more insight into the mechanism underlying the adaptation behavior of fish in a complex environment.
更多查看译文
AI 理解论文
溯源树
样例
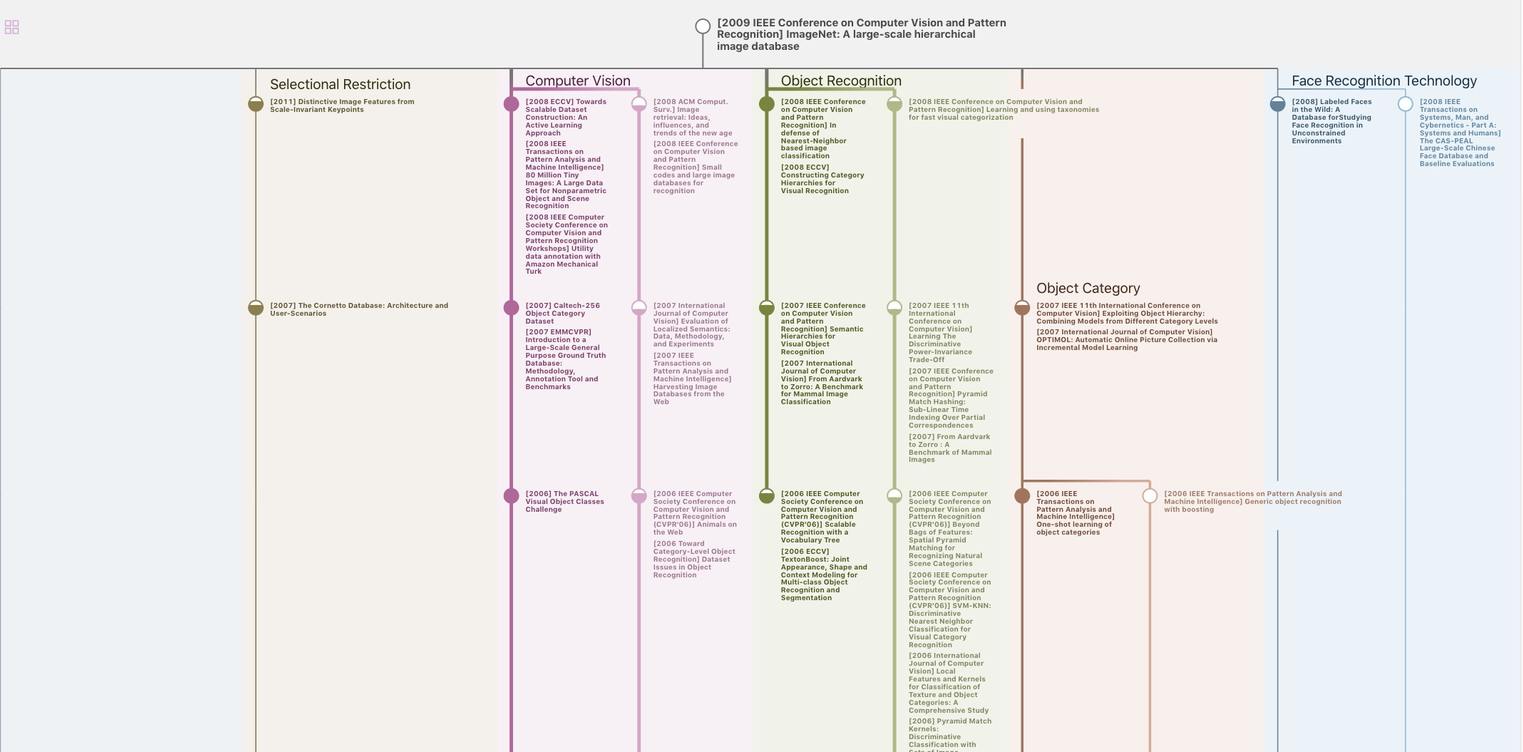
生成溯源树,研究论文发展脉络
Chat Paper
正在生成论文摘要