VADER: Vector-Quantized Generative Adversarial Network for Motion Prediction
2023 IEEE/RSJ INTERNATIONAL CONFERENCE ON INTELLIGENT ROBOTS AND SYSTEMS, IROS(2023)
摘要
Human motion prediction is an essential component for enabling close-proximity human-robot collaboration. The task of accurately predicting human motion is non-trivial and is compounded by the variability of human motion and the presence of multiple humans in proximity. To address some of the open challenges in motion prediction, in this work, we propose VADER, a novel sequence learning algorithm that models past observed poses using a flexible discrete latent space. VADER introduces the concept of Vector Quantization for human motion prediction, enabling the learning of a discrete latent space without being restricted by any static prior. In addition, we propose a new objective function that uses the discriminator objective to penalize deviation of predicted motion from the ground-truth. Finally, to explicitly model interaction in multiple humans, we introduce a lightweight attention mechanism to condition per-agent prediction on the previous hidden states of all the agents. Our evaluation across three scenarios: single-agent, multi-agent, and human-robot collaboration shows that VADER outperformed all the state-of-the-art approaches, resulting in more feasible human poses that align better with the ground-truth. Finally, we conducted extensive ablation studies to emphasize the importance of the proposed modules.
更多查看译文
关键词
Action Recognition,Outlier Detection,View Transformation Model,3D Human Pose,Cross-View Recognition
AI 理解论文
溯源树
样例
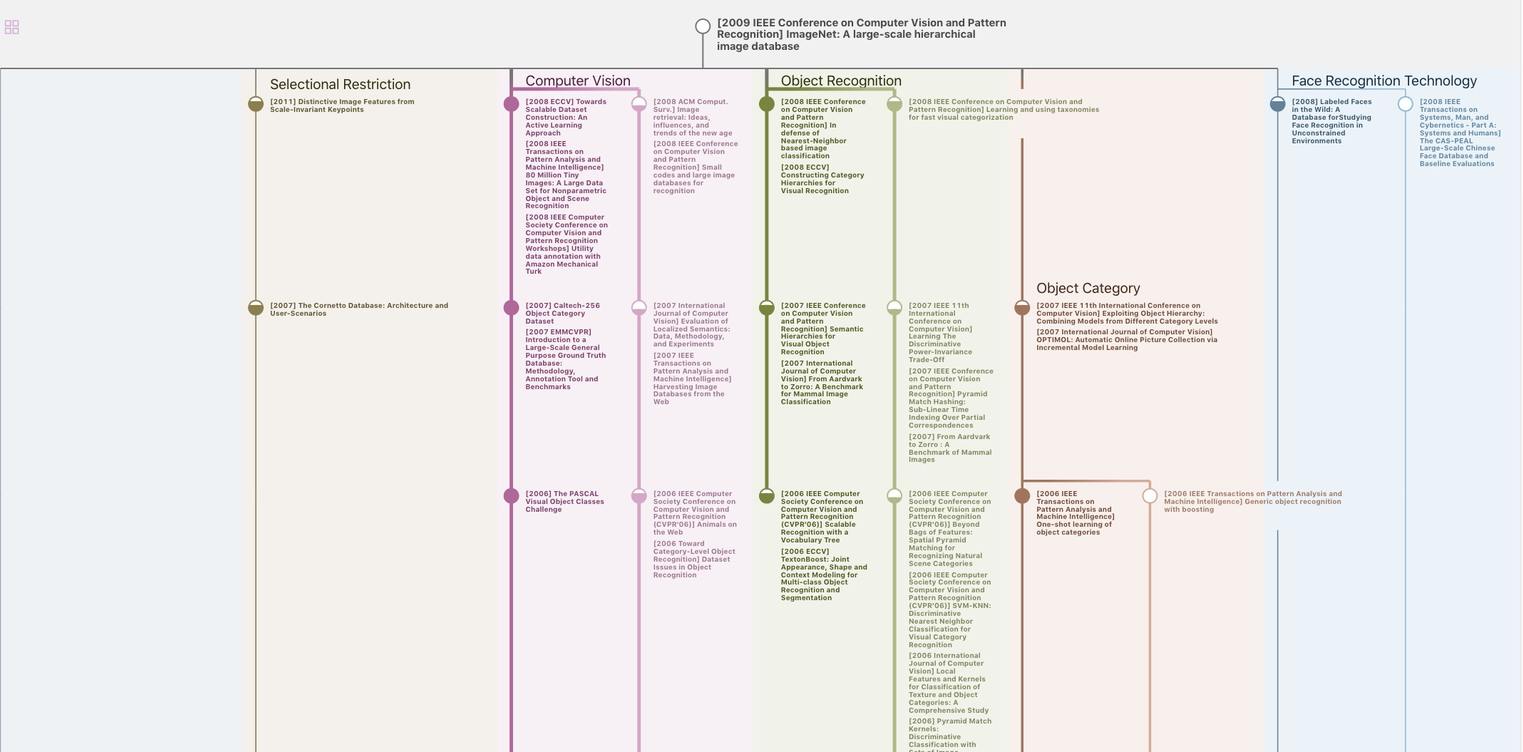
生成溯源树,研究论文发展脉络
Chat Paper
正在生成论文摘要