FeatDANet: Feature-level Domain Adaptation Network for Semantic Segmentation
2023 IEEE/RSJ INTERNATIONAL CONFERENCE ON INTELLIGENT ROBOTS AND SYSTEMS, IROS(2023)
摘要
Unsupervised domain adaptation (UDA) is proposed to better adapt the network trained on labeled synthetic data to unlabeled real-world data for addressing the annotation cost. However, most of these methods pay more attention to domain distributions in input and output stages while ignoring the important differences in semantic expressions and local details in middle feature stages. Therefore, a novel UDA network named FeatDANet is presented to align feature-level domain distributions at each encoder layer. Specifically, two attention-based modules abbreviated as IFAM and DFLM are designed and implemented by mixing queries and keys between domains for advisable domain adaptation. The former realizes Inter-domain Features Alignment by transferring feature style, and the latter achieves Domain-invariant Features Learning robustly for the domain shift. Furthermore, FeatDANet is constructed as a self-training network with three weight-sharing branches, and an improved pseudo-labels learning strategy is suggested by identifying more confident pseudo-labels and maximizing the use of pseudo-labels. It increases the participation of unlabeled data and also ensures stability in training. Extensive experiments show that FeatDANet achieves state-of-the-art performances on the tasks of GTA -> Cityscapes and Synthia -> Cityscapes.
更多查看译文
AI 理解论文
溯源树
样例
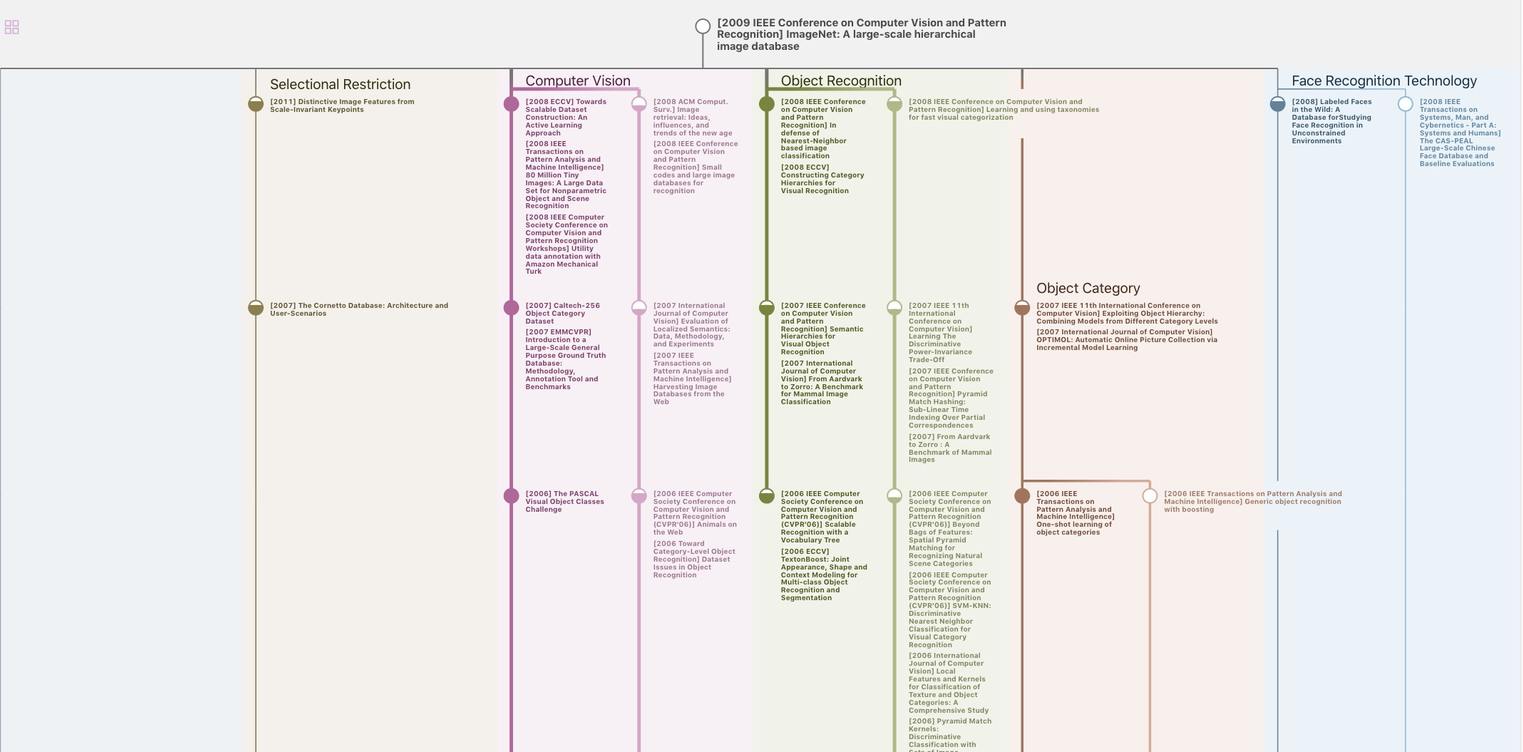
生成溯源树,研究论文发展脉络
Chat Paper
正在生成论文摘要