Evaluation Metrics of Object Detection for Quantitative System-Level Analysis of Safety-Critical Autonomous Systems
2023 IEEE/RSJ INTERNATIONAL CONFERENCE ON INTELLIGENT ROBOTS AND SYSTEMS (IROS)(2023)
摘要
This paper proposes two metrics for evaluating learned object detection models: the proposition-labeled and distance-parametrized confusion matrices. These metrics are leveraged to quantitatively analyze the system with respect to its system-level formal specifications via probabilistic model checking. In particular, we derive transition probabilities from these confusion matrices to compute the probability that the closed-loop system satisfies its system-level specifications expressed in temporal logic. Instead of using object class labels, the proposition-labeled confusion matrix uses atomic propositions relevant to the high-level control strategy. Furthermore, unlike the traditional confusion matrix, the proposed distance-parametrized confusion matrix accounts for variations in detection performance with respect to the distance between the ego and the object. Empirically, these evaluation metrics, chosen by considering system-level specifications and control module design, result in less conservative system-level evaluations than those from traditional confusion matrices. We demonstrate this framework on a car-pedestrian example by computing the satisfaction probabilities for safety requirements formalized in Linear Temporal Logic.
更多查看译文
AI 理解论文
溯源树
样例
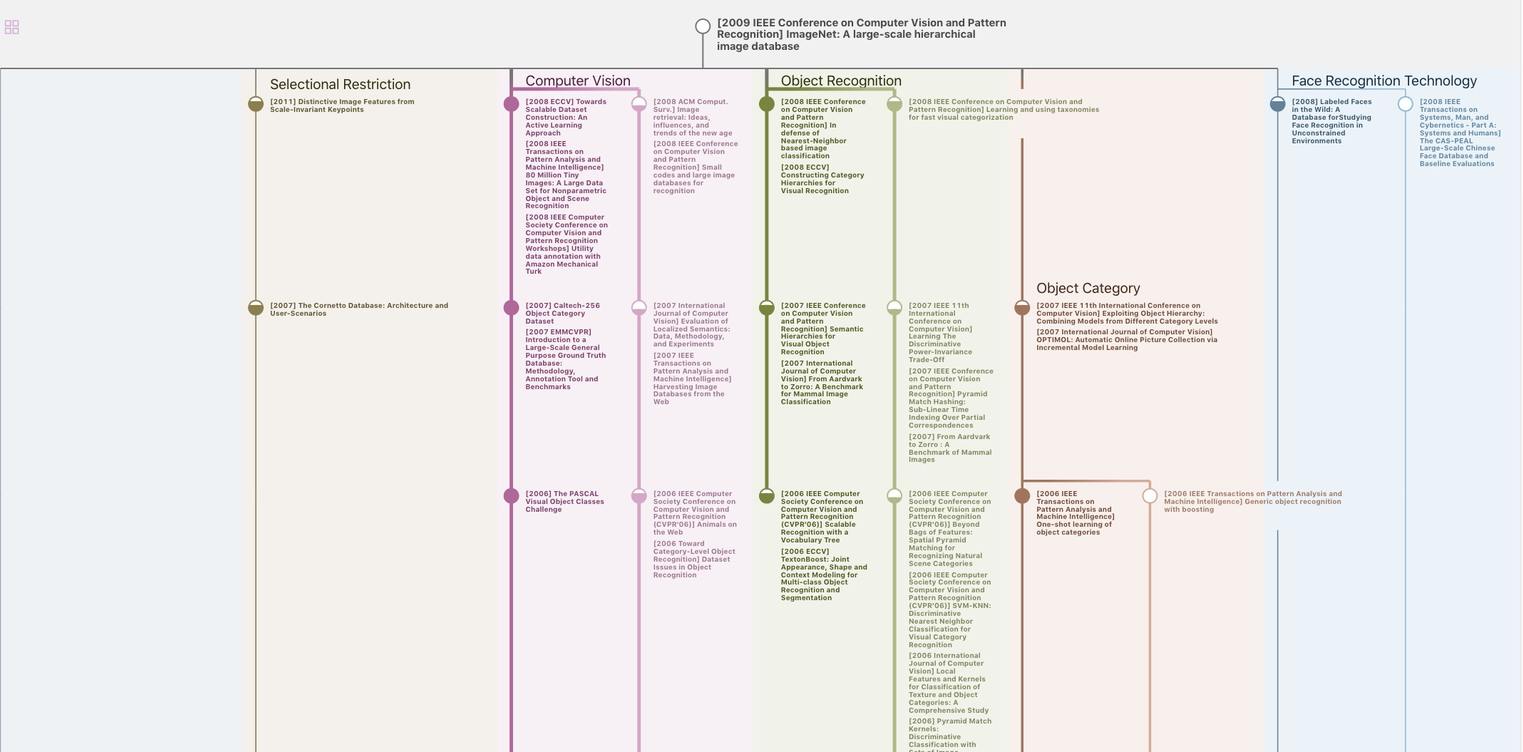
生成溯源树,研究论文发展脉络
Chat Paper
正在生成论文摘要