Machine Learning-Assisted Analysis of Advanced STDP for Neuromorphic Computing using MRAM
2023 IEEE Nanotechnology Materials and Devices Conference (NMDC)(2023)
摘要
A spiking neural network (SNN) comprises spiking neurons that mimic the information transfer in biological neurons using a series of time-dependent spikes. The spikes from such neurons are sparse in time and space, and event-driven. This facilitates the development of low-power neuromorphic hardware when coupled with bioplausible local spike-timing-dependent plasticity (STDP) learning algorithm that can encode temporal information to solve complicated time-dependent pattern recognition problems. The SNN hardware implementation using novel memristive (spintronic) devices is an active area of research to achieve area and power efficiency. Spintronic devices pave for hardware-efficient implementation of complex neuromorphic algorithms such as STDP for in-situ learning. This work presents the implementation of STDP algorithms using spin-based synaptic devices. Moreover, using an unsupervised learning scheme, the paper presents an SNN for digit recognition that is based on the mechanism with increased biological plausibility with 3 different STDP learning rules. The results show the testing accuracy of the triplet-based STDP rule is 18.34%, 10.72%, 7.15%, and 3.53% higher than the pair-based STDP rule with 100, 250, 400, and 1600 excitatory neurons respectively. The energy with the presented device is reported as 300 fJ.
更多查看译文
关键词
Image classification,memristive devices,neuromorphic computing,spiking neural network,spintronic devices
AI 理解论文
溯源树
样例
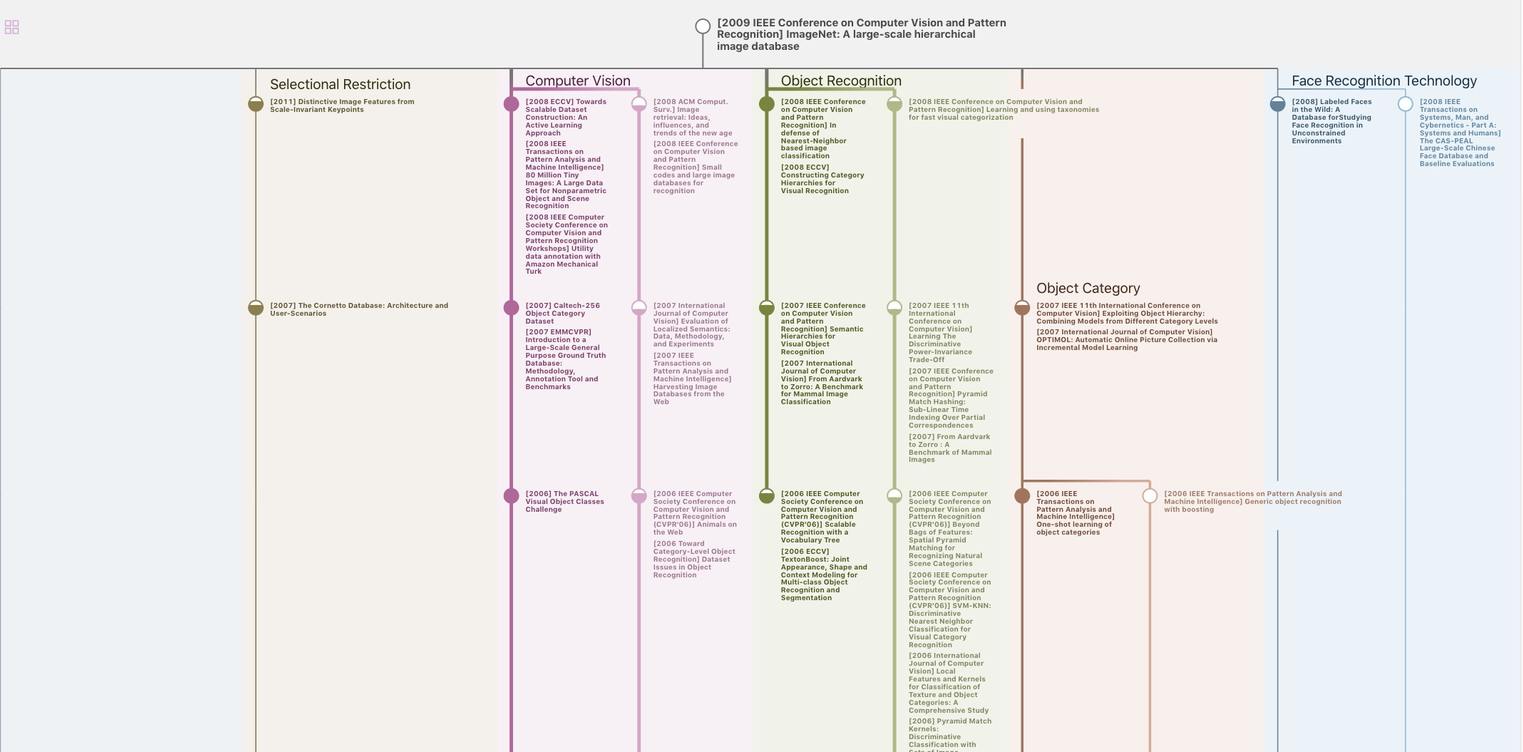
生成溯源树,研究论文发展脉络
Chat Paper
正在生成论文摘要