Semantic Segmentation based on Multiple Granularity Learning
2023 IEEE/RSJ INTERNATIONAL CONFERENCE ON INTELLIGENT ROBOTS AND SYSTEMS (IROS)(2023)
摘要
Accurate and robust coarse semantic segmentation plays a key role in the pursuit of autonomous driving. We present an algorithm that regularizes the representation space of Semantic Segmentation by Multiple Granularity Learning (SSMGL). This approach explores multiple levels of semantic knowledge in an unified framework, where the fine-grained semantic information can be either labeled or unlabeled. In our experiments, we find that SSMGL can achieve better results (1) on both on-road and off-road benchmarks, (2) under different segmentation architectures, or (3) with different backbones. The method is plug-and-play, not specialized for autonomous driving applications, and can be easily extended to any other segmentation scenario. Moreover, our SSMGL approach does not increase the computational overhead in the inference stage.
更多查看译文
AI 理解论文
溯源树
样例
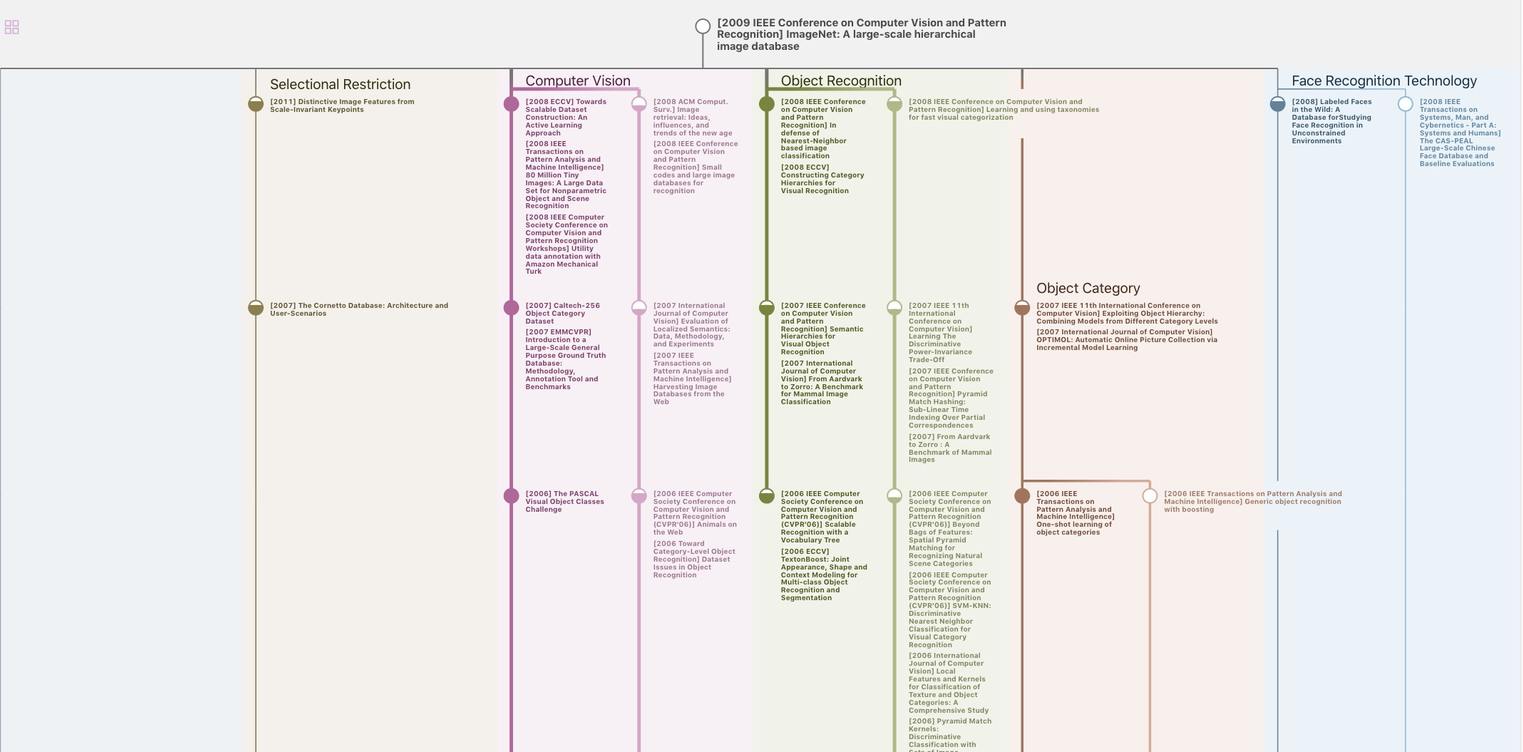
生成溯源树,研究论文发展脉络
Chat Paper
正在生成论文摘要