Deep Learning Image Denoising in PET: Quantitative Impact on Kinetic Modeling and Clinical Metrics
2023 IEEE Nuclear Science Symposium, Medical Imaging Conference and International Symposium on Room-Temperature Semiconductor Detectors (NSS MIC RTSD)(2023)
摘要
The increased sensitivity exhibited by long axial field-of-view (LAFOV) PET systems allows measurement of tracer kinetics across multiple organs and lesions at reduced doses and/or scan times. This flexibility has been utilized to perform dual-tracer imaging which in oncology offers complementary quantification about metabolic tumor processes. Yet to make dual-tracer studies more suitable for clinical practice, these scan protocols should be pushed towards lower injection doses and shorter scan times, but reduced count statistics inherently result in noisier PET images. Many have previously shown promising denoising performance of deep learning (DL) methods for low count PET images acquired at a single (static) late time point. However, only a few studies have investigated the use of DL to denoise dynamic PET data and the impact on kinetic modeling of parametric estimates. We first trained a DL algorithm (U-Net) with low-to-high count static
18
F-FDG datasets and evaluated its performance using noise and clinical metrics. We next assessed the transferability of this pre-trained DL model for frame-by-frame image denoising of dynamic
18
F-FGln and looked at the effect of such DL-based denoising on estimations of the volume of distribution of
18
F-FGln (kinetic parameter to quantify glutamine pool size). This work provides the foundation for our next investigations into the use of DL for optimizing scan protocols to make dual-tracer studies more practical, and more accurate.
更多查看译文
AI 理解论文
溯源树
样例
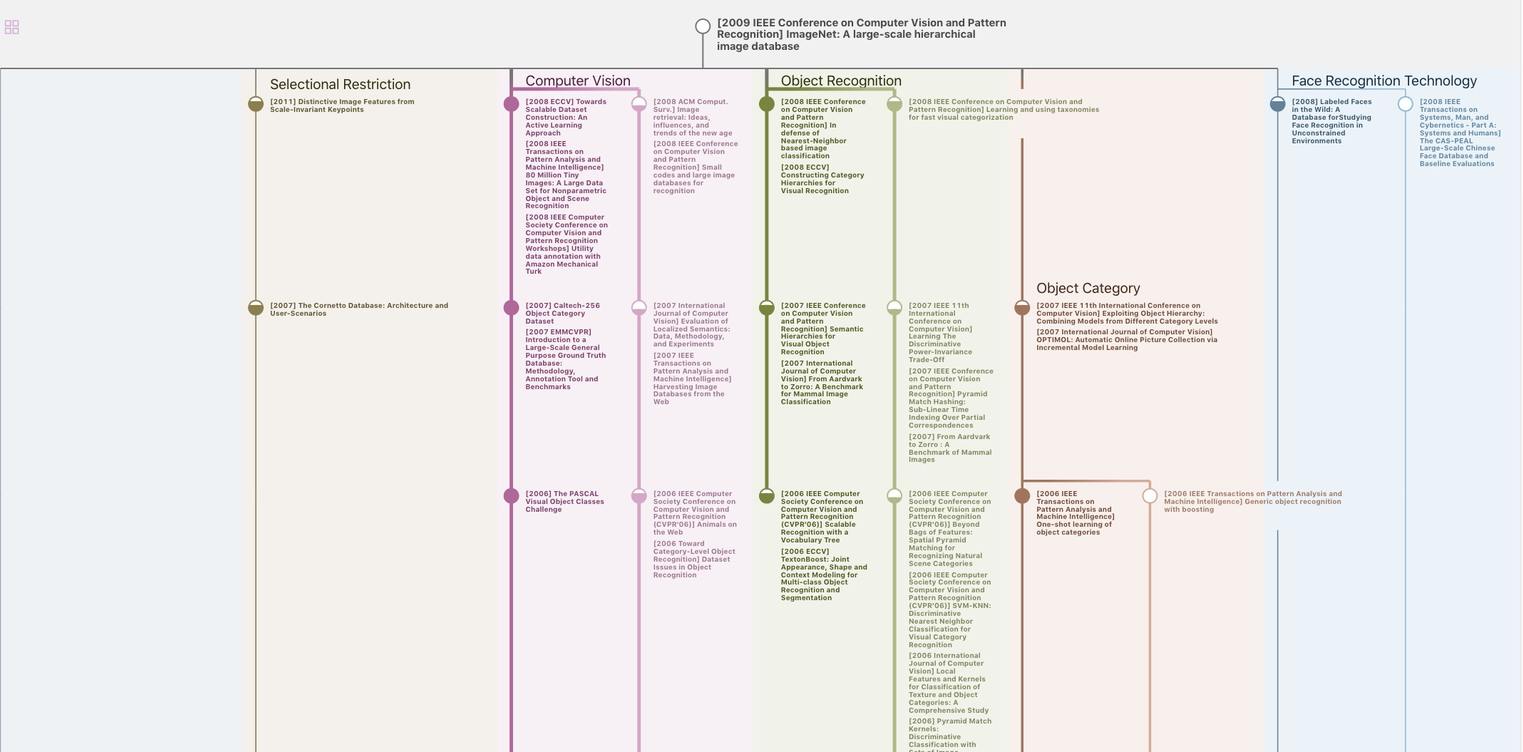
生成溯源树,研究论文发展脉络
Chat Paper
正在生成论文摘要