A novel deletion mutation in the LPA gene in a middle-aged woman with ischaemic stroke.
BMC medical genomics(2021)
摘要
BACKGROUND:Genetic diversity of the human LPA gene locus is associated with high plasma concentrations of lipoprotein(a) [Lp(a)]. High Lp(a) concentrations are strongly associated with a high incidence rate of ischaemic stroke.
CASE PRESENTATION:A 46-year-old female Chinese patient suffered from ischaemic stroke. Upon admission to the hospital, the patient was diagnosed with an elevated level of plasma Lp(a). The patient's clinical symptoms were alleviated by administration of basilar artery stent thrombectomy, mannitol, and aspirin. A novel compound heterozygous deletion of the region containing exons 3-16 covering kringle IV copy number variation (KIV CNV) domains in the LPA gene was observed in genetic analysis by next-generation sequencing and confirmed by qPCR.
CONCLUSIONS:In the current study, we reported a case of a 46-year-old female patient diagnosed with ischaemic stroke. This novel heterozygous deletion mutation in the LPA gene expands the spectrum of LPA mutations. Further study is required to understand the mechanism of LPA mutations in ischaemic stroke.
更多查看译文
AI 理解论文
溯源树
样例
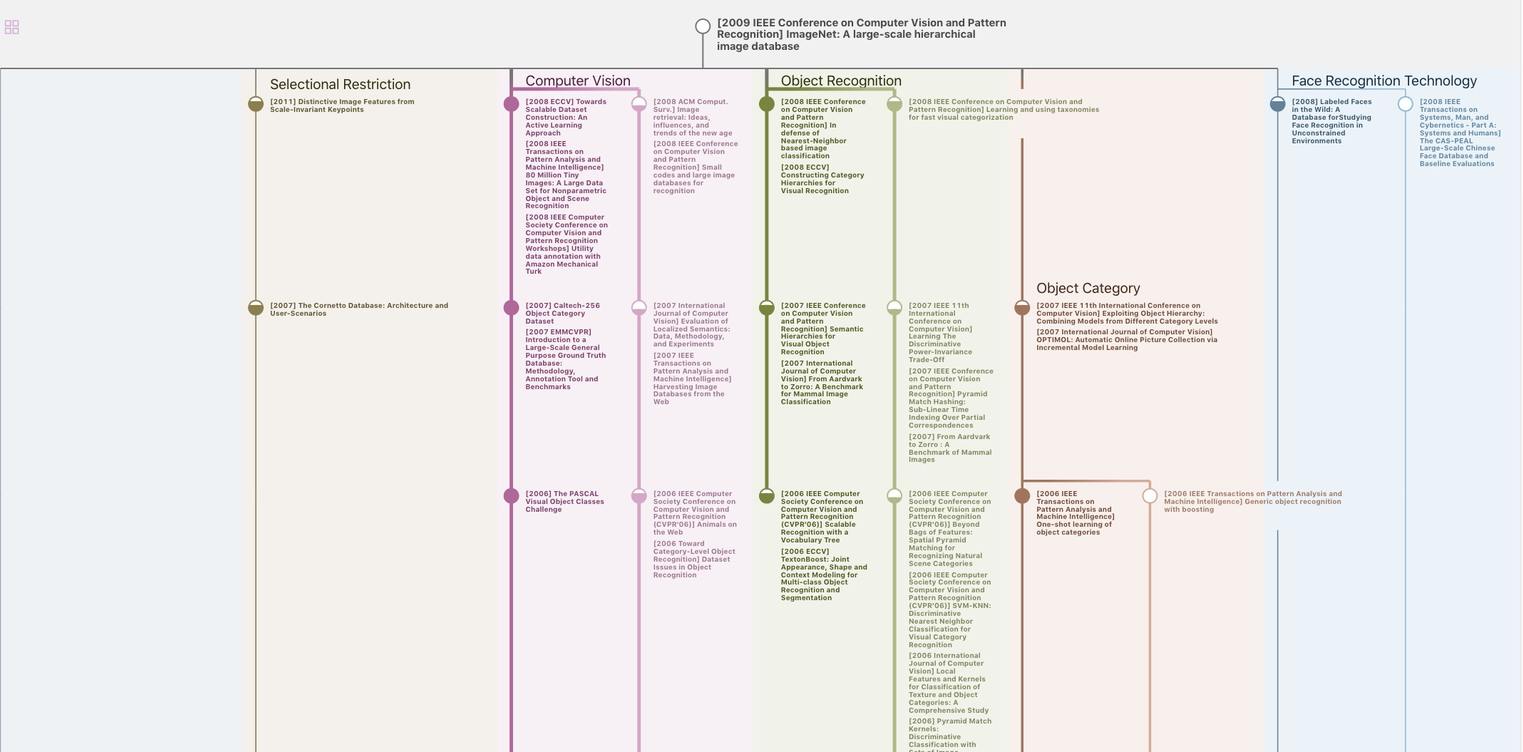
生成溯源树,研究论文发展脉络
Chat Paper
正在生成论文摘要