Corpus Modeling and the Geometries of Text
The Oxford Handbook of the Sociology of Machine Learning(2023)
摘要
Abstract This chapter explores the theoretical implications of spatial metaphors in the field of computational text analysis and inspects how the properties of topologies aid and inhibit our theories of textual meaning. Rather than mining for “ground truth,” machine learning algorithms for text, especially word embedding models, provide a selectively simplistic map of the semantic space. The representation of that textual map depends not only on the choice of algorithm but also on the composition of the corpora used to train them. Along with reviewing the technical aspects of embedding text into space, this chapter surveys the consequences of training algorithms with internal and external objectives. The implications of different types of training corpora are enumerated, with particular attention to ethical considerations. More scholarship, institutional support, and technical infrastructure directed toward the careful building, documenting, and sharing of corpora as well as machine learning models trained on those corpora are recommended.
更多查看译文
AI 理解论文
溯源树
样例
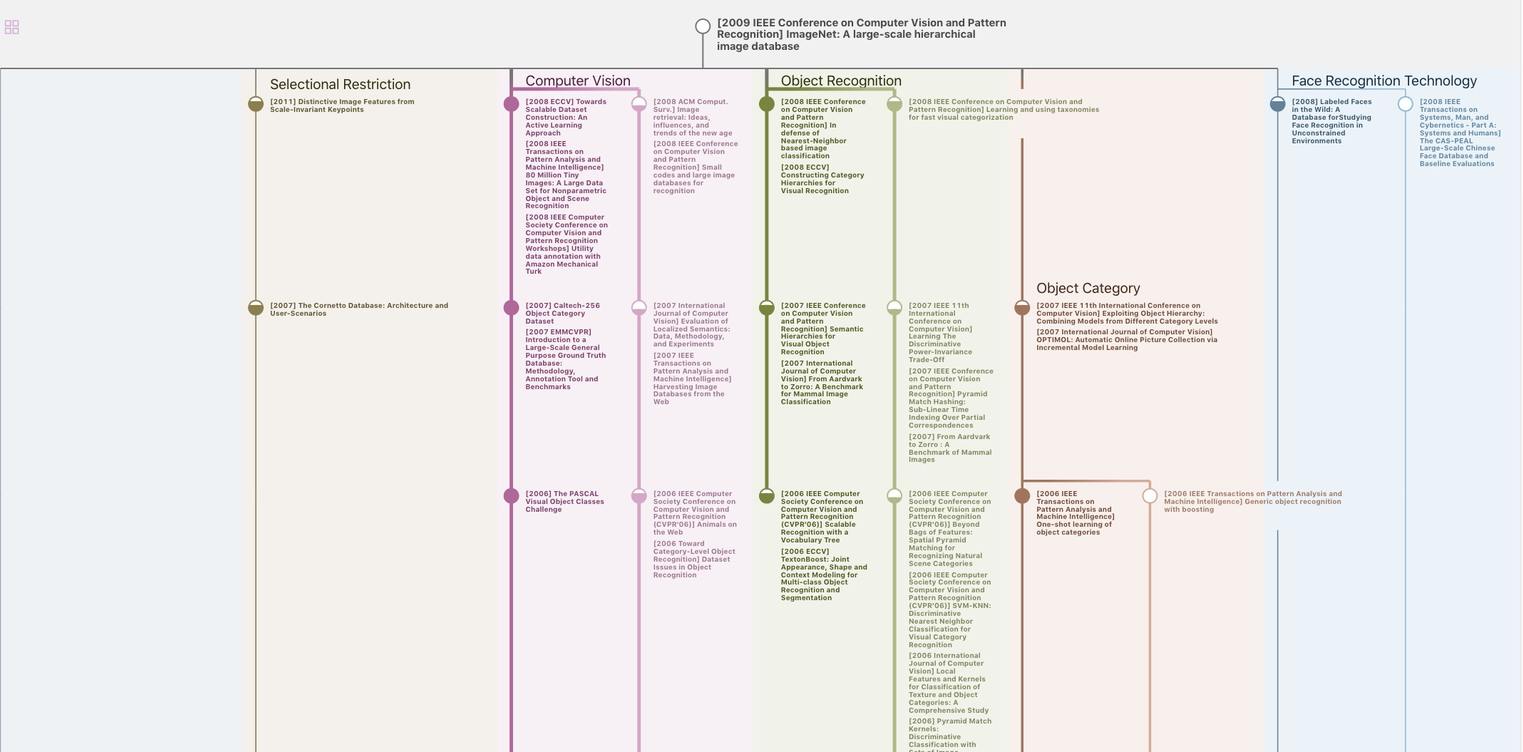
生成溯源树,研究论文发展脉络
Chat Paper
正在生成论文摘要